Edge Artificial Intelligence for real-time automatic quantification of filariasis in mobile microscopy
medrxiv(2023)
摘要
Filariasis, a neglected tropical disease caused by roundworms, is a significant public health concern in many tropical countries. Microscopic examination of blood samples can detect and differentiate parasite species, but it is time consuming and requires expert microscopists, a resource that is not always available. In this context, artificial intelligence (AI) can assist in the diagnosis of this disease by automatically detecting and differentiating microfilarias. In line with the target product profile for lymphatic filariasis as defined by the World Health Organization, we developed an edge AI system running on a smartphone whose camera is aligned with the ocular of an optical microscope that detects and differentiates filarias species in real time without the internet connection. Our object detection algorithm that uses the Single-Shot Detection (SSD) MobileNet V2 detection model was developed with 115 cases, 85 cases with 1903 fields of view and 3342 labels for model training, and 30 cases with 484 fields of view and 873 labels for model validation before clinical validation, is able to detect microfilarias at 10x magnification and distinguishes four species of them at 40x magnification: Loa loa, Mansonella perstans, Wuchereria bancrofti , and Brugia malayi . We validated our augmented microscopy system in the clinical environment by replicating the diagnostic workflow encompassed examinations at 10x and 40x with the assistance of the AI models analyzing 18 samples with the AI running on a middle range smartphone. It achieved an overall precision of 94.14%, recall of 91.90% and F1 score of 93.01% for the screening algorithm and 95.46%, 97.81% and 96.62% for the species differentiation algorithm respectively. This innovative solution has the potential to support filariasis diagnosis and monitoring, particularly in resource-limited settings where access to expert technicians and laboratory equipment is scarce.
### Competing Interest Statement
LL, ED, ND, DB-P, CCab, DC, OD, JG-V, AB, MP, ER-J, MJL-C, AS and MLO work for Spotlab and/or hold shares or phantom shares of Spotlab. The rest of the authors declare no competing interests.
### Clinical Trial
ISRCTN 38872
### Funding Statement
This project has received funding from the European Union Horizon 2020 research and innovation programme under grant agreement No 881062. LL was supported by a predoctoral grant IND2019/TIC-17167 (Comunidad de Madrid). This work was supported by the Bill and Melinda Gates Foundation (grant number Edge-Spot project INV-051355).
### Author Declarations
I confirm all relevant ethical guidelines have been followed, and any necessary IRB and/or ethics committee approvals have been obtained.
Yes
The details of the IRB/oversight body that provided approval or exemption for the research described are given below:
Ethical approval was obtained from the Research Ethics Committee (REC) Instituto de Salud Carlos III, Spain (CEI PI 74_2020).
I confirm that all necessary patient/participant consent has been obtained and the appropriate institutional forms have been archived, and that any patient/participant/sample identifiers included were not known to anyone (e.g., hospital staff, patients or participants themselves) outside the research group so cannot be used to identify individuals.
Yes
I understand that all clinical trials and any other prospective interventional studies must be registered with an ICMJE-approved registry, such as ClinicalTrials.gov. I confirm that any such study reported in the manuscript has been registered and the trial registration ID is provided (note: if posting a prospective study registered retrospectively, please provide a statement in the trial ID field explaining why the study was not registered in advance).
Yes
I have followed all appropriate research reporting guidelines, such as any relevant EQUATOR Network research reporting checklist(s) and other pertinent material, if applicable.
Yes
Images and labels can be shared for research purposes upon request. Please contact Miguel Luengo-Oroz (miguel{at}spotlab.ai).
更多查看译文
关键词
filariasis,automatic quantification,edge,artificial intelligence,real-time
AI 理解论文
溯源树
样例
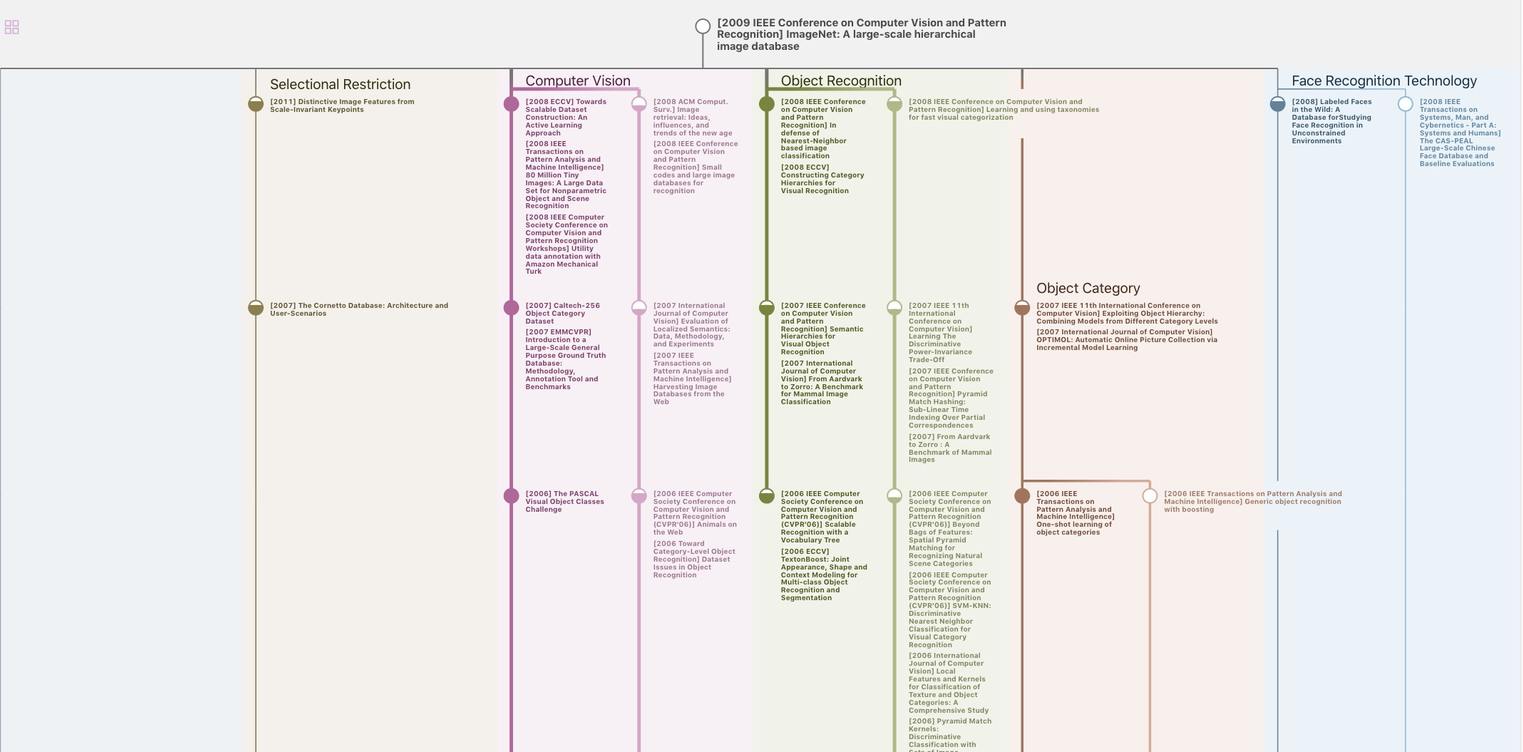
生成溯源树,研究论文发展脉络
Chat Paper
正在生成论文摘要