Risk Score Models for Unplanned Urinary Tract Infection Hospitalization
medRxiv (Cold Spring Harbor Laboratory)(2023)
摘要
Annually, urinary tract infections (UTIs) affect over a hundred million people worldwide. Early detection of high-risk individuals can help prevent hospitalization for UTIs, which imposes significant economic and social burden on patients and caregivers. We present two methods to generate risk score models for unplanned UTI hospitalization. We utilize a sample of patients from the insurance claims data provided by the Centers for Medicare and Medicaid Services to develop and validate the proposed methods. Our dataset encompasses a wide range of features, such as demographics, medical history, and healthcare utilization of the patients along with provider quality metrics and community-based metrics. The proposed methods scale and round the coefficients of an underlying logistic regression model to create scoring tables. We present computational experiments to evaluate the prediction performance of both models. We also discuss different features of these models with respect to their impact on interpretability. Our findings emphasize the effectiveness of risk score models as practical tools for identifying high-risk patients and provide a quantitative assessment of the significance of various risk factors in unplanned UTI hospitalizations such as admission to ICU in the last 3 months, cognitive disorders and low inpatient, outpatient and carrier costs in the last 6 months.
### Competing Interest Statement
The authors have declared no competing interest.
### Funding Statement
The author(s) received no specific funding for this work.
### Author Declarations
I confirm all relevant ethical guidelines have been followed, and any necessary IRB and/or ethics committee approvals have been obtained.
Yes
The details of the IRB/oversight body that provided approval or exemption for the research described are given below:
The overall project with human subjects research was reviewed by the Institutional Research Board at North Carolina State University (IRB Protocol 20528). Data storage, maintenance, and protection are governed by the Data Use Agreement between the university and the CMS.
I confirm that all necessary patient/participant consent has been obtained and the appropriate institutional forms have been archived, and that any patient/participant/sample identifiers included were not known to anyone (e.g., hospital staff, patients or participants themselves) outside the research group so cannot be used to identify individuals.
Yes
I understand that all clinical trials and any other prospective interventional studies must be registered with an ICMJE-approved registry, such as [ClinicalTrials.gov][1]. I confirm that any such study reported in the manuscript has been registered and the trial registration ID is provided (note: if posting a prospective study registered retrospectively, please provide a statement in the trial ID field explaining why the study was not registered in advance).
Yes
I have followed all appropriate research reporting guidelines, such as any relevant EQUATOR Network research reporting checklist(s) and other pertinent material, if applicable.
Yes
The data underlying the results presented in the study are available from Centers for Medicare and Medicaid Services ([data.cms.gov][2]).
[1]: https://ClinicalTrials.gov
[2]: https://data.cms.gov
更多查看译文
关键词
urinary tract infection,risk score models
AI 理解论文
溯源树
样例
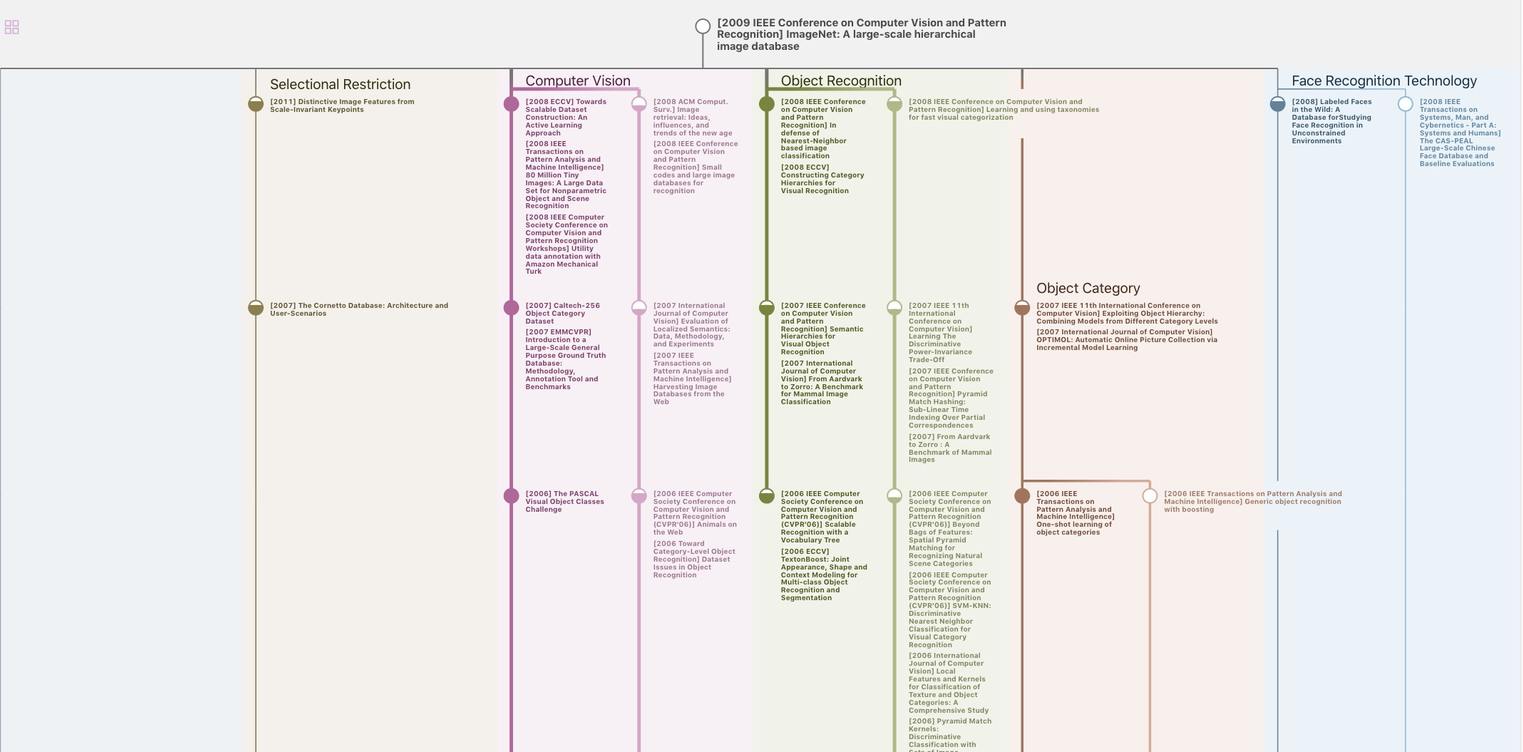
生成溯源树,研究论文发展脉络
Chat Paper
正在生成论文摘要