Long-term Prediction of Severe Hypoglycemia in Type 2 Diabetes Based on Multi-view Co-training
medRxiv (Cold Spring Harbor Laboratory)(2023)
摘要
Background Patients with type 2 diabetes mellitus (T2DM) who have severe hypoglycemia (SH) poses a considerable risk of long-term death, demanding urgent medical attention. Accurate prediction of SH remains challenging due to its multifactorial nature, contributed from factors such as medications, lifestyle choices, and metabolic measurements.
Method In this study, we propose a systematic approach to improve the robustness and accuracy of SH predictions using machine learning models, guided by clinical feature selection. Our focus is on developing one-year SH prediction models using both semi-supervised learning and supervised learning algorithms. Utilizing the clinical trial, namely Action to Control Cardiovascular Risk in Diabetes, which involves electronic health records for over 10,000 individuals, we specifically investigate adults with T2DM who are at an increased risk of cardiovascular complications.
Results Our results indicate that the application of a multi-view co-training method, incorporating the random forest algorithm, improves the specificity of SH prediction, while the same setup with Naive Bayes replacing random forest demonstrates better sensitivity. Our framework also provides interpretability of machine learning (XAI) models by identifying key predictors for hypoglycemia, including fast plasma glucose, hemoglobin A1c, general diabetes education, and NPH or L insulins.
Conclusion By enhancing prediction accuracy and identifying crucial predictive features, our study contributes to advancing the understanding and management of hypoglycemia in this population.
### Competing Interest Statement
The authors have declared no competing interest.
### Funding Statement
This study did not receive any funding
### Author Declarations
I confirm all relevant ethical guidelines have been followed, and any necessary IRB and/or ethics committee approvals have been obtained.
Yes
The details of the IRB/oversight body that provided approval or exemption for the research described are given below:
The ethics committee of Beth Israel Deaconess Medical Center determined that this study was exempt re: review and approval.
I confirm that all necessary patient/participant consent has been obtained and the appropriate institutional forms have been archived, and that any patient/participant/sample identifiers included were not known to anyone (e.g., hospital staff, patients or participants themselves) outside the research group so cannot be used to identify individuals.
Yes
I understand that all clinical trials and any other prospective interventional studies must be registered with an ICMJE-approved registry, such as ClinicalTrials.gov. I confirm that any such study reported in the manuscript has been registered and the trial registration ID is provided (note: if posting a prospective study registered retrospectively, please provide a statement in the trial ID field explaining why the study was not registered in advance).
Yes
I have followed all appropriate research reporting guidelines, such as any relevant EQUATOR Network research reporting checklist(s) and other pertinent material, if applicable.
Yes
The Action to Control Cardiovascular Risk in Diabetes (ACCORD) data is available upon request from the National Heart, Lung, and Blood Institute (NHLBI).
* ### Abbreviations
更多查看译文
关键词
severe hypoglycemia,diabetes,prediction,long-term,multi-view,co-training
AI 理解论文
溯源树
样例
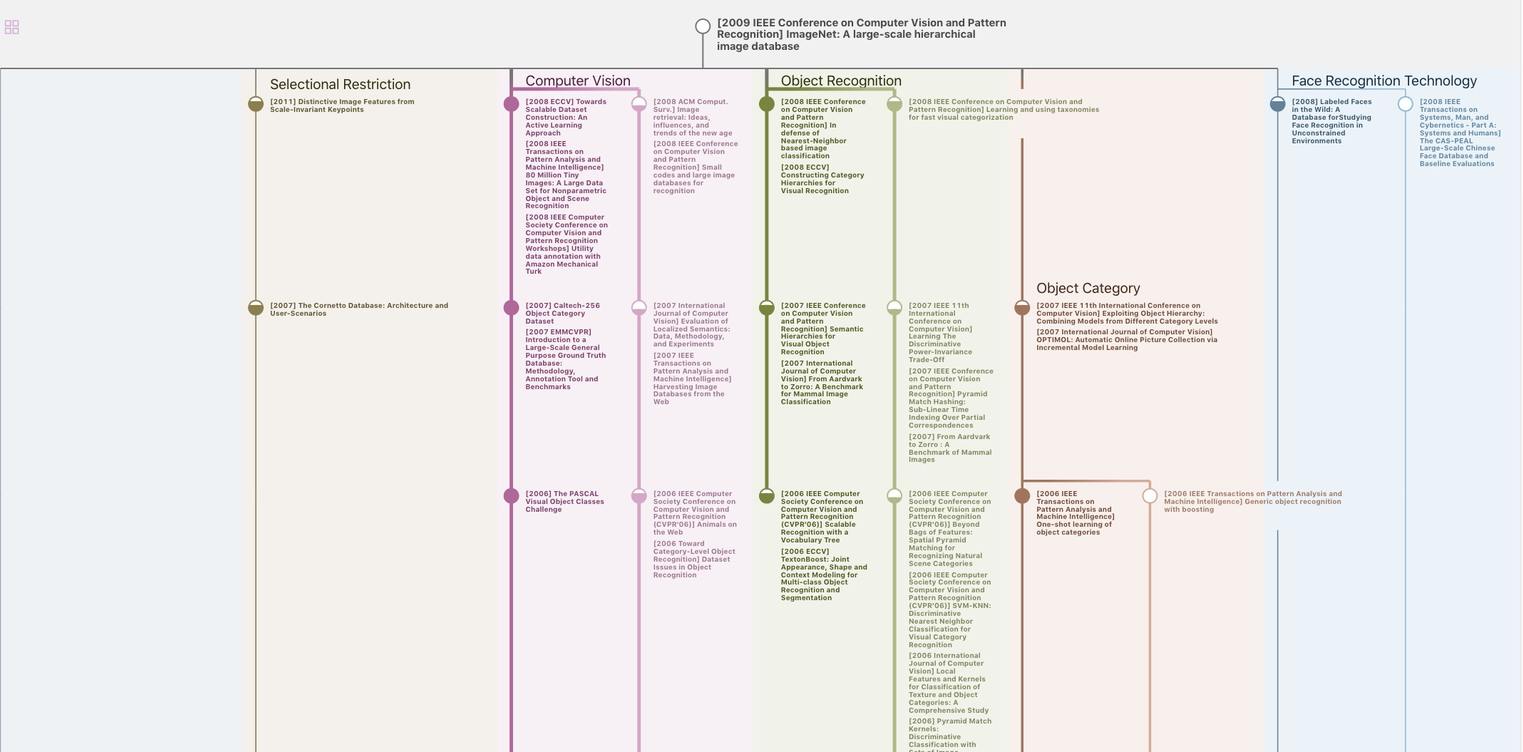
生成溯源树,研究论文发展脉络
Chat Paper
正在生成论文摘要