D-SAT: dual semantic aggregation transformer with dual attention for medical image segmentation
PHYSICS IN MEDICINE AND BIOLOGY(2024)
摘要
Objective. Medical image segmentation is significantly essential to assist clinicians in facilitating a quick and accurate diagnoses. However, most of the existing methods are still challenged by the loss of semantic information, blurred boundaries and the huge semantic gap between the encoder and decoder. Approach. To tackle these issues, a dual semantic aggregation transformer with dual attention is proposed for medical image segmentation. Firstly, the dual-semantic feature aggregation module is designed to build a bridge between convolutional neural network (CNN) and Transformer, effectively aggregating CNN's local feature detail ability and Transformer's long-range modeling ability to mitigate semantic information loss. Thereafter, the strip spatial attention mechanism is put forward to alleviate the blurred boundaries during encoding by constructing pixel-level feature relations across CSWin Transformer blocks from different spatial dimensions. Finally, a feature distribution gated attention module is constructed in the skip connection between the encoder and decoder to decrease the large semantic gap by filtering out the noise in low-level semantic information when fusing low-level and high-level semantic features during decoding. Main results. Comprehensive experiments conducted on abdominal multi-organ segmentation, cardiac diagnosis, polyp segmentation and skin lesion segmentation serve to validate the generalization and effectiveness of the proposed dual semantic aggregation transformer with dual attention (D-SAT). The superiority of D-SAT over current state-of-the-art methods is substantiated by both subjective and objective evaluations, revealing its remarkable performance in terms of segmentation accuracy and quality. Significance. The proposed method subtly preserves the local feature details and global context information in medical image segmentation, providing valuable support to improve diagnostic efficiency for clinicians and early disease control for patients. Code is available at https://github.com/Dxkm/D-SAT.
更多查看译文
关键词
medical image segmentation,transformer,dual-semantic feature aggregation,strip spatial attention,feature distribution gated attention
AI 理解论文
溯源树
样例
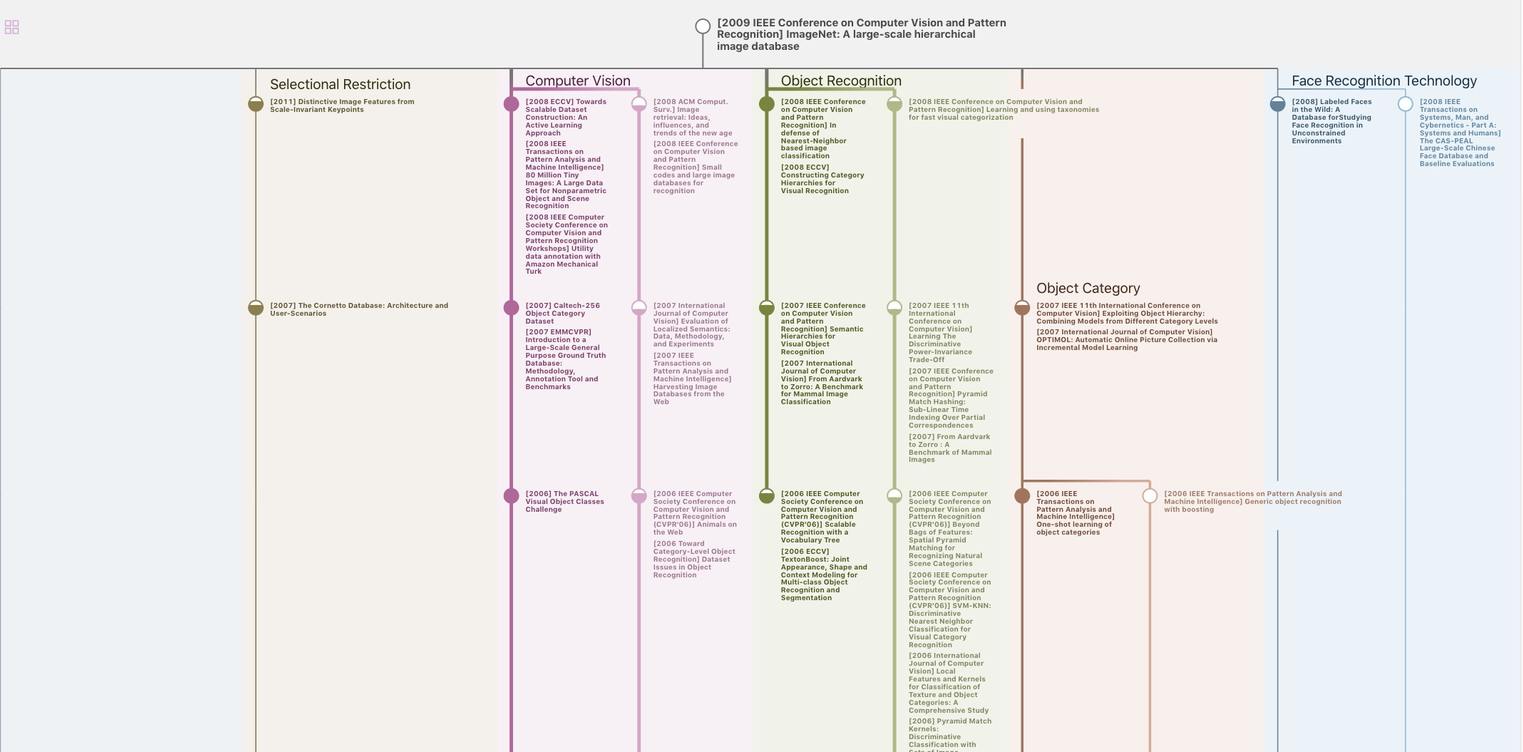
生成溯源树,研究论文发展脉络
Chat Paper
正在生成论文摘要