Data-driven decision-focused surrogate modeling
AICHE JOURNAL(2024)
摘要
We introduce the concept of decision-focused surrogate modeling for solving computationally challenging nonlinear optimization problems in real-time settings. The proposed data-driven framework seeks to learn a simpler, for example, convex, surrogate optimization model that is trained to minimize the decision prediction error, which is defined as the difference between the optimal solutions of the original and the surrogate optimization models. The learning problem, formulated as a bilevel program, can be viewed as a data-driven inverse optimization problem to which we apply a decomposition-based solution algorithm from previous work. We validate our framework through numerical experiments involving the optimization of common nonlinear chemical processes such as chemical reactors, heat exchanger networks, and material blending systems. We also present a detailed comparison of decision-focused surrogate modeling with standard data-driven surrogate modeling methods and demonstrate that our approach is significantly more data-efficient while producing simple surrogate models with high decision prediction accuracy.
更多查看译文
关键词
decision-focused learning,hybrid modeling,inverse optimization,surrogate modeling
AI 理解论文
溯源树
样例
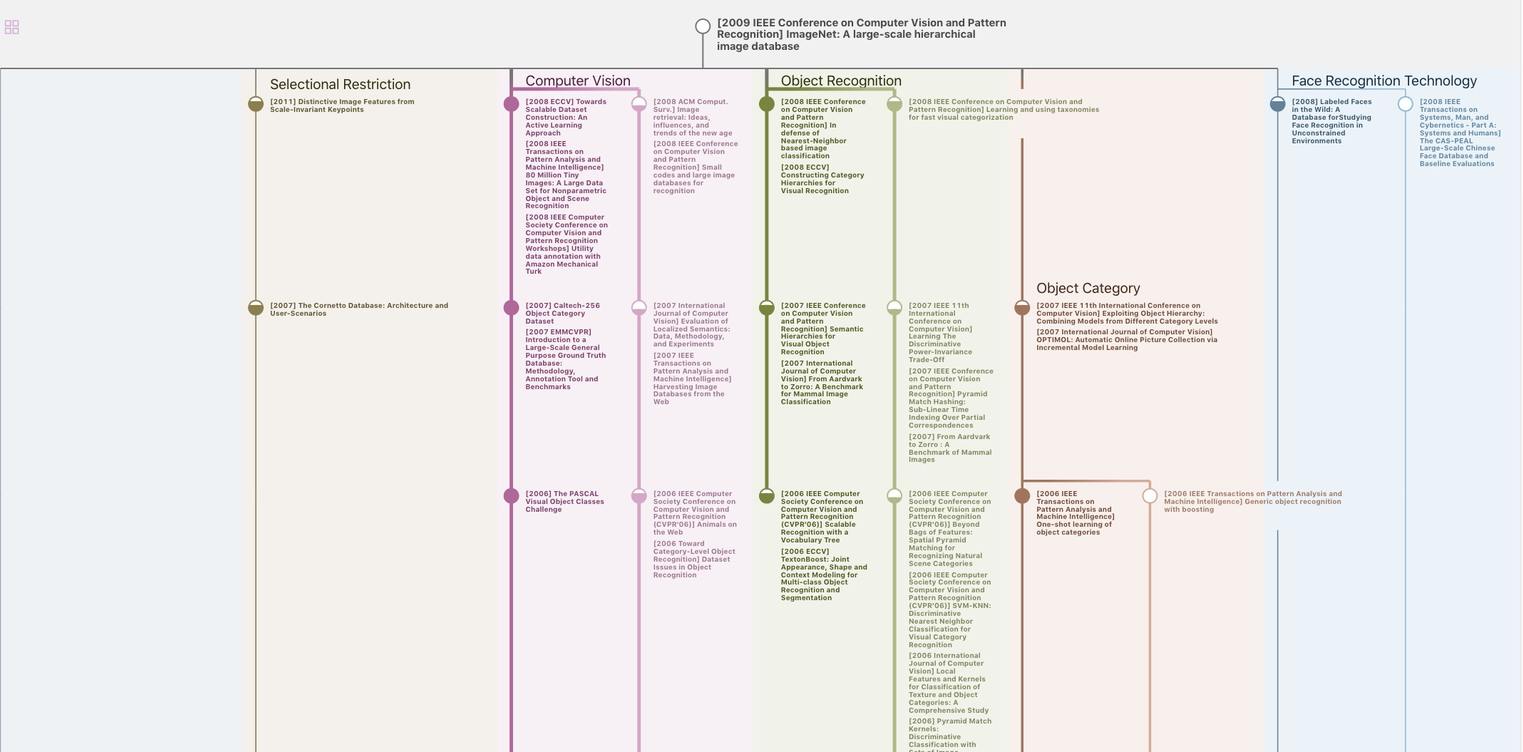
生成溯源树,研究论文发展脉络
Chat Paper
正在生成论文摘要