Path-Constrained State Estimation for Rail Vehicles
CoRR(2023)
摘要
Globally rising demand for transportation by rail is pushing existing infrastructure to its capacity limits, necessitating the development of accurate, robust, and high-frequency positioning systems to ensure safe and efficient train operation. As individual sensor modalities cannot satisfy the strict requirements of robustness and safety, a combination thereof is required. We propose a path-constrained sensor fusion framework to integrate various modalities while leveraging the unique characteristics of the railway network. To reflect the constrained motion of rail vehicles along their tracks, the state is modeled in 1D along the track geometry. We further leverage the limited action space of a train by employing a novel multi-hypothesis tracking to account for multiple possible trajectories a vehicle can take through the railway network. We demonstrate the reliability and accuracy of our fusion framework on multiple tram datasets recorded in the city of Zurich, utilizing Visual-Inertial Odometry for local motion estimation and a standard GNSS for global localization. We evaluate our results using ground truth localizations recorded with a RTK-GNSS, and compare our method to standard baselines. A Root Mean Square Error of 4.78 m and a track selectivity score of up to 94.9 % have been achieved.
更多查看译文
关键词
Rail Vehicle,Root Mean Square Error,Mean Square Error,Global Navigation Satellite System,Motion Estimation,Railway Network,Global Localization,Sensor Modalities,Visual-inertial Odometry,State Space,Multiple Hypothesis,Network Operators,Inertial Measurement Unit,Transition Model,Position Estimation,Particle Filter,Railway Line,Vehicle Position,Vehicle State,Extended Kalman Filter,Simultaneous Localization And Mapping,End Of Segment,Track Segment,Measurement Update,Rail Network,Global Coordinates,Current Tracking,Outlier Rejection,Robot Operating System,Real-world Datasets
AI 理解论文
溯源树
样例
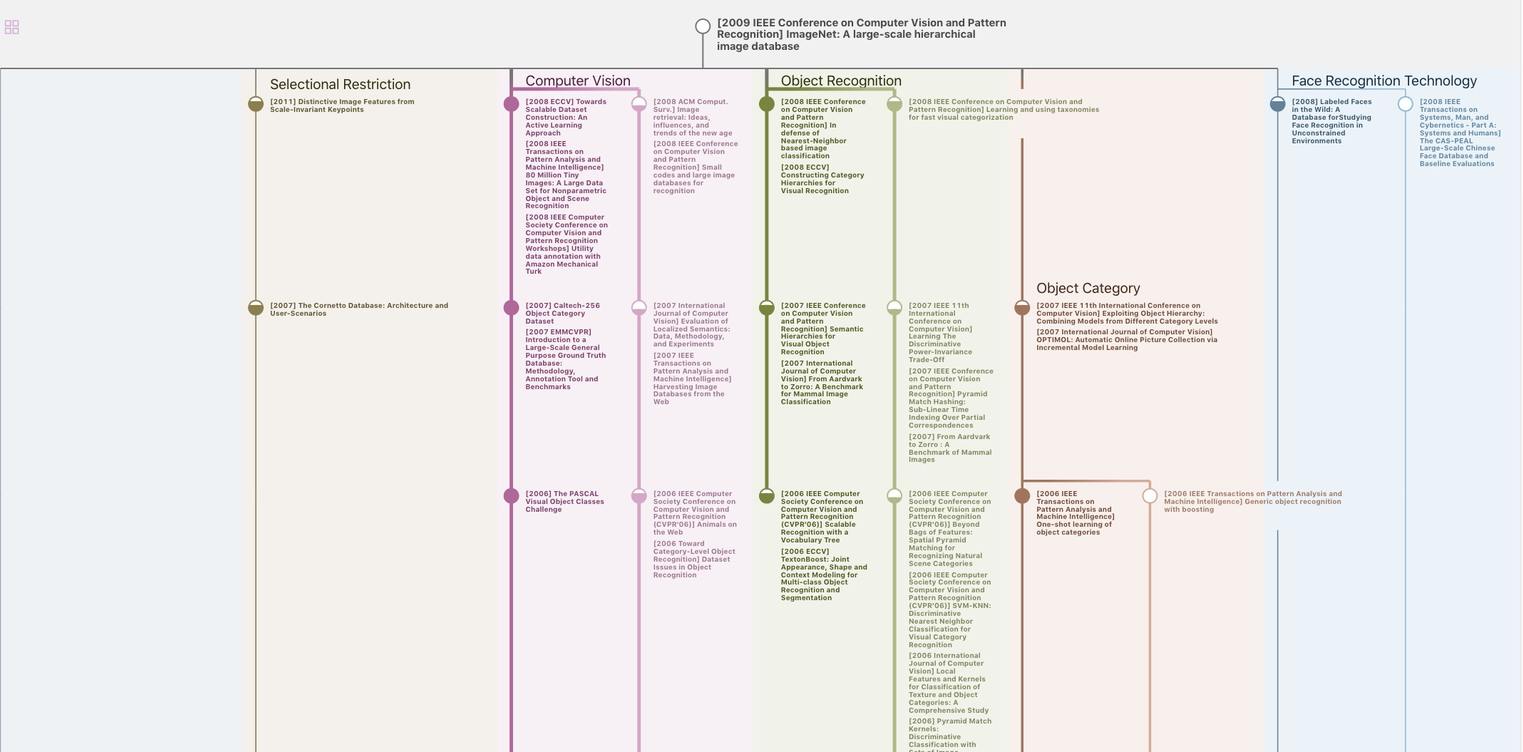
生成溯源树,研究论文发展脉络
Chat Paper
正在生成论文摘要