PREFER: Prompt Ensemble Learning via Feedback-Reflect-Refine
AAAI 2024(2024)
Abstract
As an effective tool for eliciting the power of Large Language Models (LLMs), prompting has recently demonstrated unprecedented abilities across a variety of complex tasks. To further improve the performance, prompt ensemble has attracted substantial interest for tackling the hallucination and instability of LLMs. However, existing methods usually adopt a two-stage paradigm, which requires a pre-prepared set of prompts with substantial manual effort, and is unable to perform directed optimization for different weak learners. In this paper, we propose a simple, universal, and automatic method named PREFER (Prompt Ensemble learning via Feedback-Reflect-Refine) to address the stated limitations. Specifically, given the fact that weak learners are supposed to focus on hard examples during boosting, PREFER builds a feedback mechanism for reflecting on the inadequacies of existing weak learners. Based on this, the LLM is required to automatically synthesize new prompts for iterative refinement. Moreover, to enhance stability of the prompt effect evaluation, we propose a novel prompt bagging method involving forward and backward thinking, which is superior to majority voting and is beneficial for both feedback and weight calculation in boosting. Extensive experiments demonstrate that our PREFER achieves state-of-the-art performance in multiple types of tasks by a significant margin. We have made our code publicly available.
MoreTranslated text
Key words
NLP: (Large) Language Models,ML: Calibration & Uncertainty Quantification,NLP: Text Classification
AI Read Science
Must-Reading Tree
Example
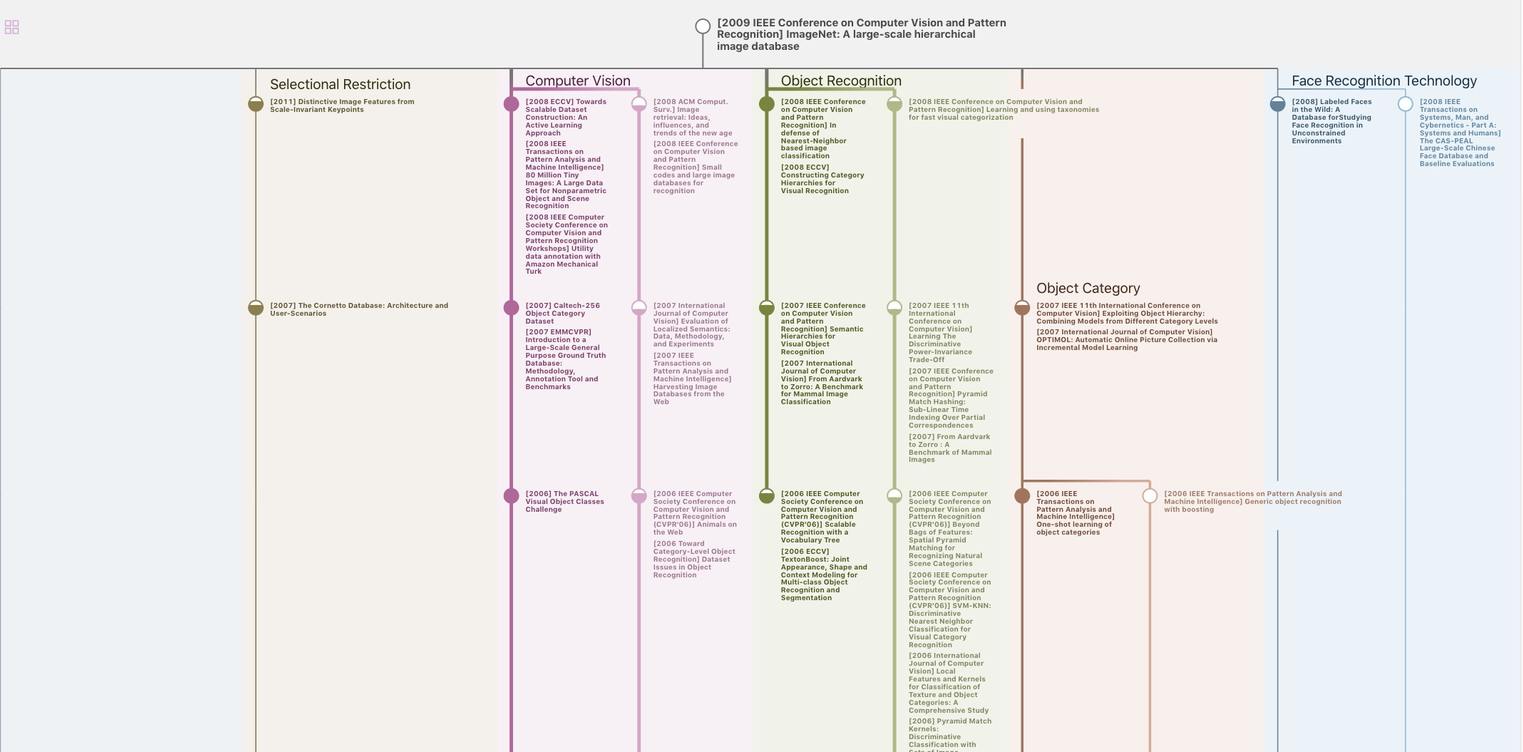
Generate MRT to find the research sequence of this paper
Chat Paper
Summary is being generated by the instructions you defined