A Scale-Invariant Task Balancing Approach for Multi-Task Learning
CoRR(2023)
Abstract
Multi-task learning (MTL), a learning paradigm to learn multiple related tasks simultaneously, has achieved great success in various fields. However, task-balancing remains a significant challenge in MTL, with the disparity in loss/gradient scales often leading to performance compromises. In this paper, we propose a Scale-Invariant Multi-Task Learning (SI-MTL) method to alleviate the task-balancing problem from both loss and gradient perspectives. Specifically, SI-MTL contains a logarithm transformation which is performed on all task losses to ensure scale-invariant at the loss level, and a gradient balancing method, SI-G, which normalizes all task gradients to the same magnitude as the maximum gradient norm. Extensive experiments conducted on several benchmark datasets consistently demonstrate the effectiveness of SI-G and the state-of-the-art performance of SI-MTL.
MoreTranslated text
AI Read Science
Must-Reading Tree
Example
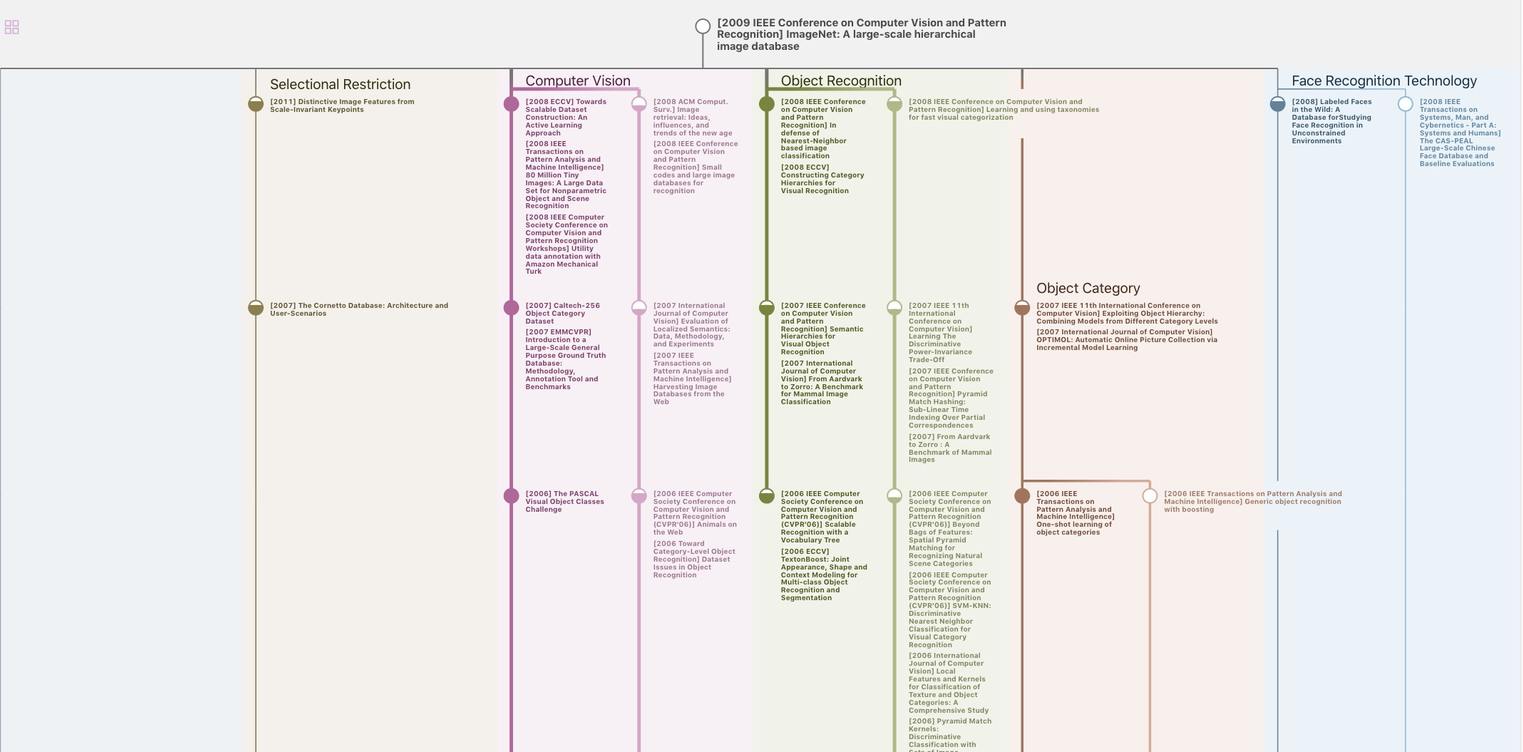
Generate MRT to find the research sequence of this paper
Chat Paper
Summary is being generated by the instructions you defined