On a Divergence-Based Prior Analysis of Stick-Breaking Processes
arXiv (Cornell University)(2023)
摘要
The nonparametric view of Bayesian inference has transformed statistics and many of its applications. The canonical Dirichlet process and other more general families of nonparametric priors have served as a gateway to solve frontier uncertainty quantification problems of large, or infinite, nature. This success has been greatly due to available constructions and representations of such distributions, which in turn have lead to a variety of sampling schemes. Undoubtedly, the two most useful constructions are the one based on normalization of homogeneous completely random measures and that based on stick-breaking processes, as well as various particular cases. Understanding their distributional features and how different random probability measures compare among themselves is a key ingredient for their proper application. In this paper, we explore the prior discrepancy, through a divergence-based analysis, of extreme classes of stick-breaking processes. Specifically, we investigate the random Kullback-Leibler divergences between the Dirichlet process and the geometric process, as well as some of their moments. Furthermore, we also perform the analysis within the general exchangeable stick-breaking class of nonparametric priors, leading to appealing results.
更多查看译文
关键词
prior analysis,divergence-based,stick-breaking
AI 理解论文
溯源树
样例
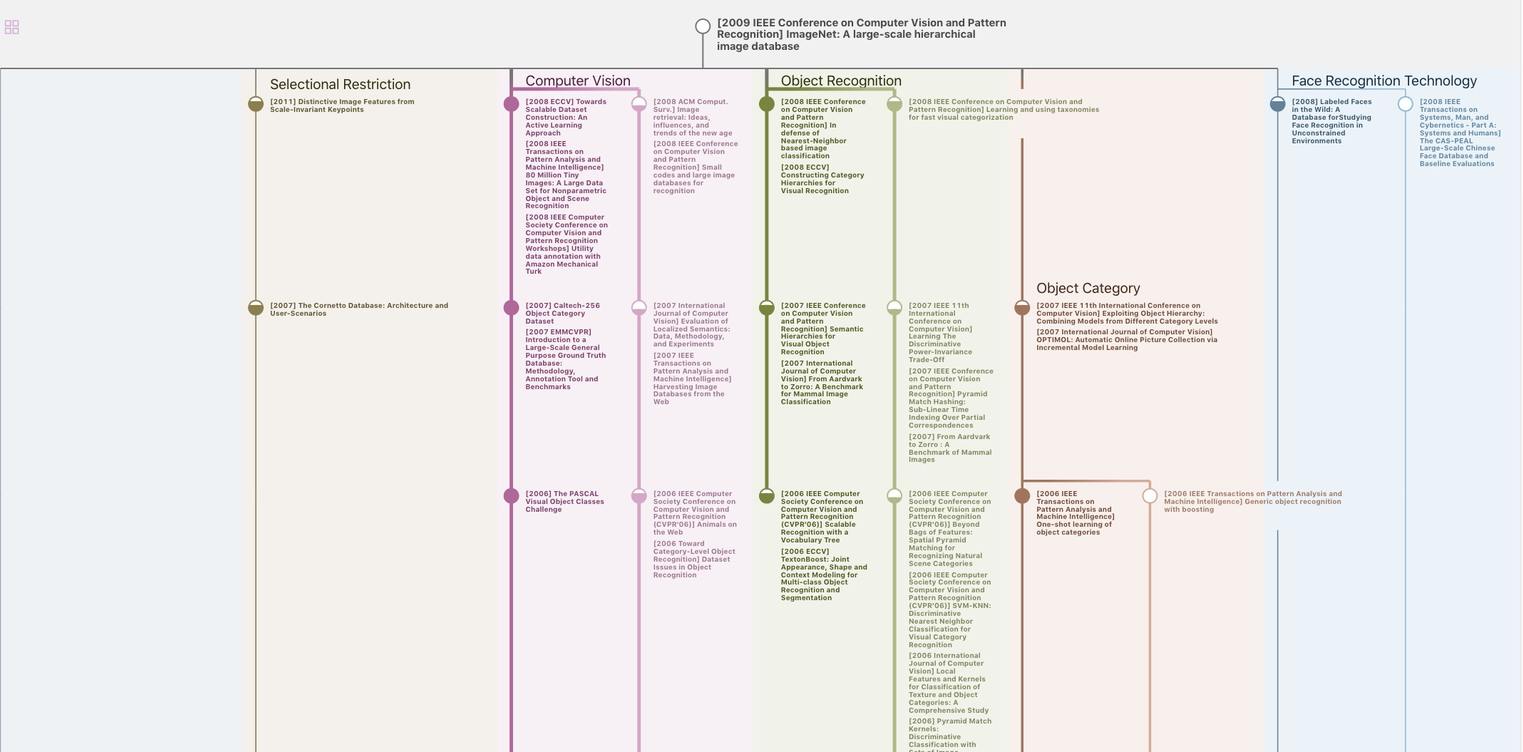
生成溯源树,研究论文发展脉络
Chat Paper
正在生成论文摘要