Few-Shot Anomaly Detection in Text with Deviation Learning
NEURAL INFORMATION PROCESSING, ICONIP 2023, PT II(2024)
摘要
Most current methods for detecting anomalies in text concentrate on constructing models solely relying on unlabeled data. These models operate on the presumption that no labeled anomalous examples are available, which prevents them from utilizing prior knowledge of anomalies that are typically present in small numbers in many realworld applications. Furthermore, these models prioritize learning feature embeddings rather than optimizing anomaly scores directly, which could lead to suboptimal anomaly scoring and inefficient use of data during the learning process. In this paper, we introduce FATE, a deep few-shot learning-based framework that leverages limited anomaly examples and learns anomaly scores explicitly in an end-to-end method using deviation learning. In this approach, the anomaly scores of normal examples are adjusted to closely resemble reference scores obtained from a prior distribution. Conversely, anomaly samples are forced to have anomalous scores that considerably deviate from the reference score in the upper tail of the prior. Additionally, our model is optimized to learn the distinct behavior of anomalies by utilizing a multi-head self-attention layer and multiple instance learning approaches. Comprehensive experiments on several benchmark datasets demonstrate that our proposed approach attains a new level of state-of-the-art performance (Our code is available at https://github.com/arav1ndajay/fate/).
更多查看译文
关键词
Anomaly detection,Natural language processing,Few-shot learning,Text anomaly,Deviation learning
AI 理解论文
溯源树
样例
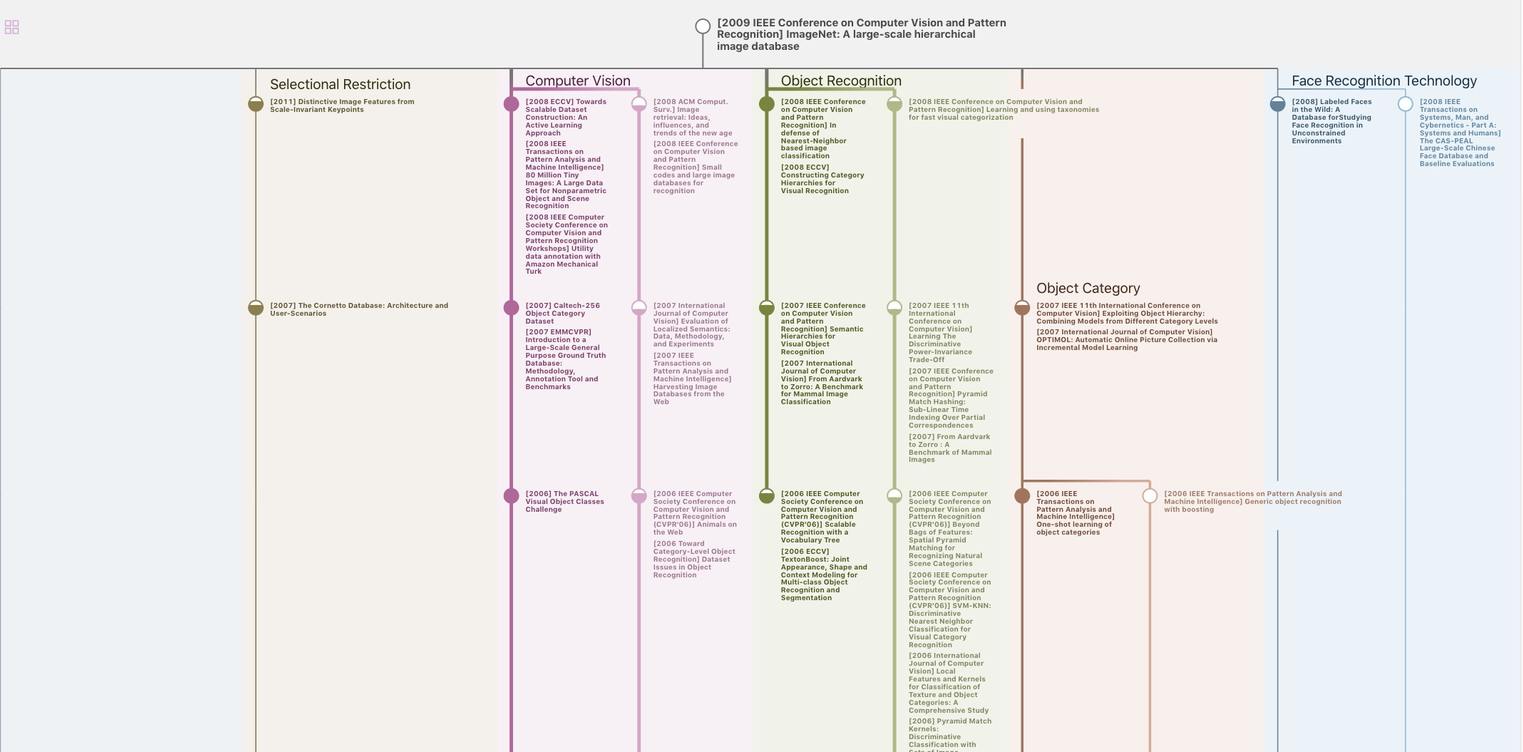
生成溯源树,研究论文发展脉络
Chat Paper
正在生成论文摘要