Machine learning force fields for molecular liquids: Ethylene Carbonate/Ethyl Methyl Carbonate binary solvent
npj Computational Materials(2023)
摘要
Highly accurate ab initio molecular dynamics (MD) methods are the gold standard for studying molecular mechanisms in the condensed phase, however, they are too expensive to capture many key properties that converge slowly with respect to simulation length and time scales. Machine learning (ML) approaches which reach the accuracy of ab initio simulation, and which are, at the same time, sufficiently affordable hold the key to bridging this gap. In this work we present a robust ML potential for the EC:EMC binary solvent, a key component of liquid electrolytes in rechargeable Li-ion batteries. We identify the necessary ingredients needed to successfully model this liquid mixture of organic molecules. In particular, we address the challenge posed by the separation of scale between intra- and inter-molecular interactions, which is a general issue in all condensed phase molecular systems.
更多查看译文
关键词
Atomistic models,Materials Science,general,Characterization and Evaluation of Materials,Mathematical and Computational Engineering,Theoretical,Mathematical and Computational Physics,Computational Intelligence,Mathematical Modeling and Industrial Mathematics
AI 理解论文
溯源树
样例
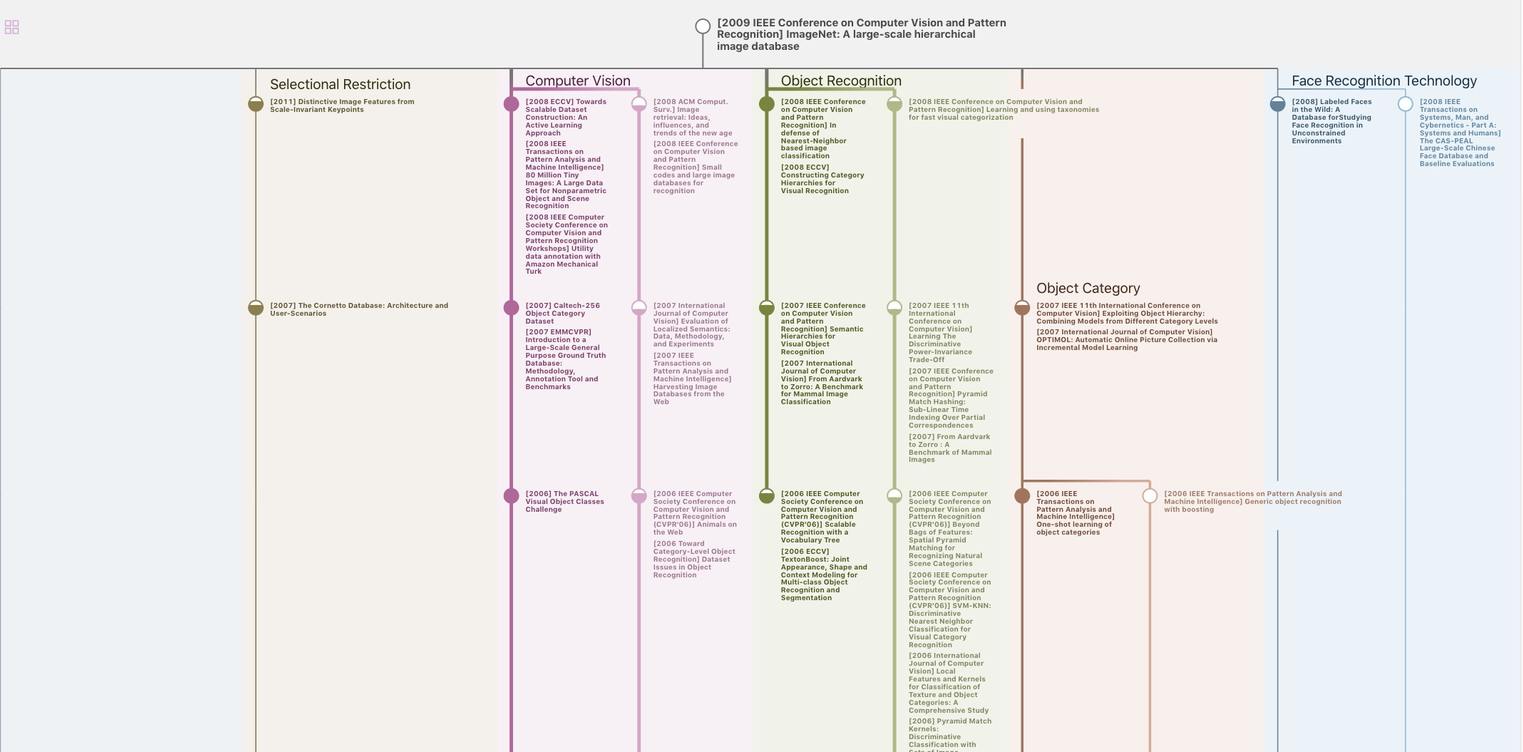
生成溯源树,研究论文发展脉络
Chat Paper
正在生成论文摘要