A Process-Structure-Property Simulation Framework for Quantifying Uncertainty in Additive Manufacturing: Application to Fatigue in Ti-6Al-4V
INTEGRATING MATERIALS AND MANUFACTURING INNOVATION(2023)
摘要
Metal additive manufacturing (AM) processes produce heterogeneous microstructures, leading to significant uncertainty in mechanical behavior. Process-induced defects cause additional uncertainty and can degrade performance, particularly for local processes like fatigue. However, time and monetary costs impose constraints on using repeated experiments to quantify this uncertainty across the process parameter space. Applying uncertainty quantification methods with computational materials simulations can help to alleviate these costs. In this paper, a physics-based process-structure–property (PSP) simulation framework is developed and applied to laser powder bed fusion AM of Ti-6Al-4V. Microstructures are generated from process-structure simulations that combine an analytical temperature field solution with a kinetic Monte Carlo-based model incorporating crystallographic texture and porosity development. The microstructures are passed to structure-property simulations comprising a fast Fourier transform-based crystal plasticity solver to predict a fatigue indicator parameter. Two uncertainty quantification problems are addressed: (1) probabilistic parameter calibration for the thermal model and (2) prediction of extreme values and the associated uncertainty of the fatigue indicator parameter using the full PSP framework. The simulations demonstrate the detrimental influence of keyhole porosity on fatigue, while also showing that pore-microstructure interaction increases uncertainty in the fatigue indicator parameter extreme values. The uncertainty quantification capabilities of the PSP framework provide a path toward using computational materials simulations to support qualification and certification of AM parts.
更多查看译文
关键词
Additive manufacturing,Uncertainty quantification,Fatigue,Crystal plasticity,Kinetic Monte Carlo
AI 理解论文
溯源树
样例
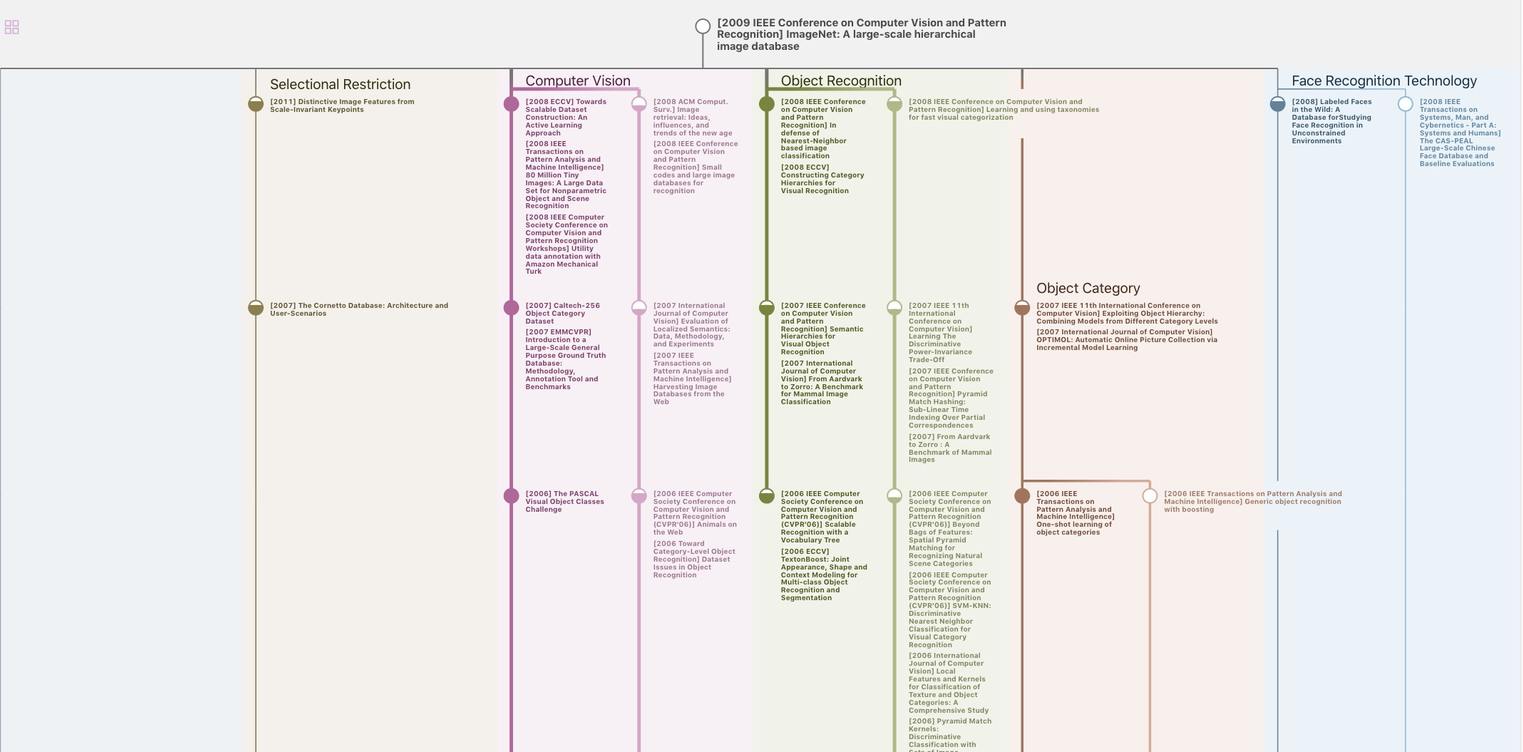
生成溯源树,研究论文发展脉络
Chat Paper
正在生成论文摘要