DETR-SPP: a fine-tuned vehicle detection with transformer
Multimedia Tools and Applications(2024)
摘要
Real-time vehicle detection is the most challenging and crucial task in intelligent transportation systems. Speed and accuracy are the most anticipated qualities for a vehicle detection model. The existing real-time vehicle detection models lack either one of these qualities, i.e., higher accuracy is achieved at the expense of speed and vice versa. This makes them unfit for real-time deployment, where both speed and accuracy are equally important. Also, occlusion is an inevitable factor that makes detection more complex and affects the system’s accuracy. Furthermore, there is no dedicated model for vehicle detection. This study proposes a better one-stage vehicle detection network, DETR-SPP, based on bipartite matching and a transformer encoder-decoder architecture. The feature extraction network, the Convolutional Neural Network (CNN), of the DEtection TRansformer (DETR) object detection model is modified to increase the real-time detection speed and accuracy. The spatial pyramid pooling concept is added to remove the fixed-size constraint and increase the learning capacity of the network. The network is trained only with vehicle classes from the MS COCO 2017 dataset, such as bus, car, motorcycle, and truck. When compared with the other state-of-the-art models, DETR-SPP gives higher accuracy in real-time vehicle detection. On the MS COCO 2017 dataset, the proposed model achieves a better mAP of 51.31%, which is 5.19% higher as compared to the DETR baseline model. Moreover, the proposed DETR-SPP attained a p value of 0.03 while performing the Wilcoxon signed-rank test. Thus, the proposed DETR-SPP is a better model for vehicle detection.
更多查看译文
关键词
Detection transformer,Intelligent transportation systems (ITS),Real-time vehicle detection,Spatial pyramid pooling (SPP),Bipartite matching
AI 理解论文
溯源树
样例
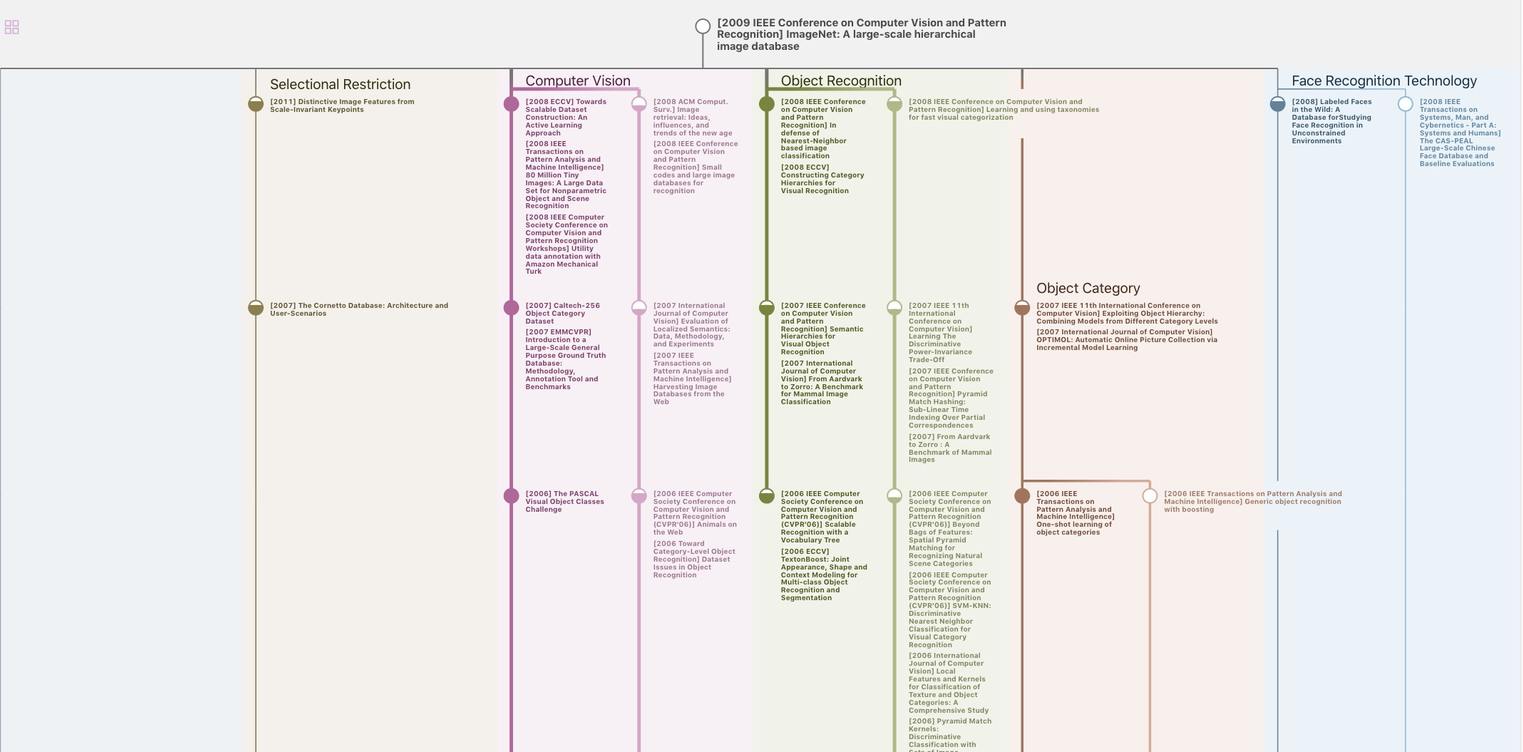
生成溯源树,研究论文发展脉络
Chat Paper
正在生成论文摘要