Enhanced machine learning approach with orthogonal frequency division multiplexing to avoid congestion in wireless communication system
Optical and Quantum Electronics(2023)
Abstract
In urban area vehicular adhoc network ascertaining appropriate channel for load balanced high data transferring, in dynamic network location is addressed by several industries and researchers using various routing algorithms. Particularly load balancing in traffic congestion and channel optimization in dynamic vehicular topology extends coverage and line-of-sight relay association issue and probability of hold-up link is high. For preventing problems instigated by traffic congestion and estimating optimistic channel for transferring high data rate without affecting network performances machine learning with orthogonal frequency division multiplexing and multi-input and multi-output (OFDM–MIMO) approach is incorporated for that takes care of load balancing and channel optimisation respectively in this paper for effective communication in vehicular network. Through miscellaneous simulations we implement Q-learning based load balancing routing (Q-LLBR) and OFDM–MIMO scheme is incorporated to achieve better performance in packet delivery ratio, latency, network utilization and signal to noise ratio are performed significantly to improve network performance in vehicular communications.
MoreTranslated text
Key words
Urban vehicular network,OFDM,MIMO,Machine learning,Packet delivery ratio,Latency,Signal to noise ratio (SNR)
AI Read Science
Must-Reading Tree
Example
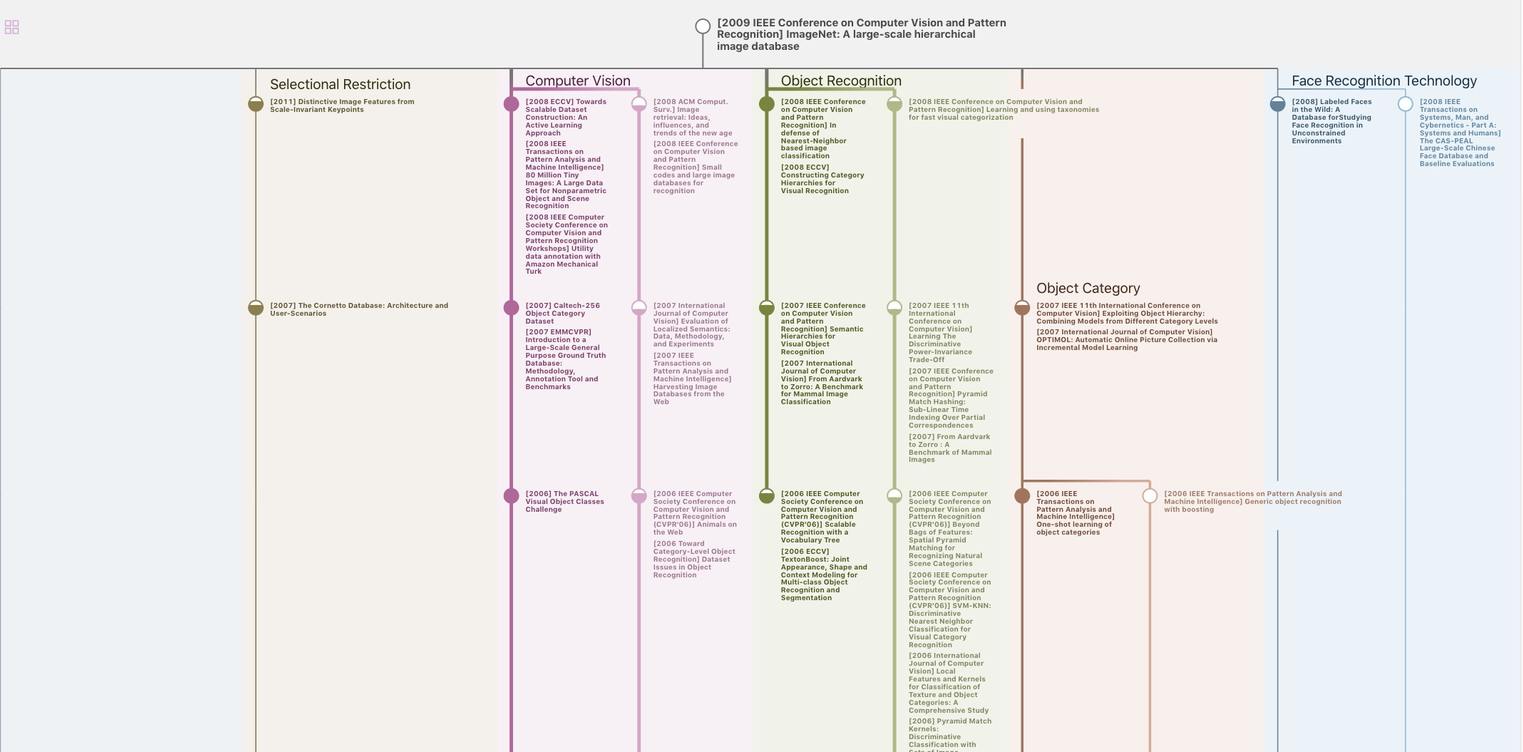
Generate MRT to find the research sequence of this paper
Chat Paper
Summary is being generated by the instructions you defined