Deep Object Detection Network Based Automatic Spinopelvic Parameters Measurement Method for Adult Spinal Deformity Classification
2023 4th International Seminar on Artificial Intelligence, Networking and Information Technology (AINIT)(2023)
摘要
Adult spinal deformity (ASD) is a spinal disease with a high incidence and a large number of patients. The treatment of ASD usually requires the evaluation of parameters in the medical radiographic images of patients’. Currently, the parameter measurement of medical images is mainly performed by orthopedists manually, which is time-consuming, and the diagnoses may vary among different orthopedists. In this paper, we proposed an end-to-end spino pelvic parameters measurement approach, which consists of three parts: the preprocessing and labeling method for the X-ray image dataset, the construction of X-ray Feature Enhancing Detector (X-FEDet) based on cross-layer multi-scale feature fusion and spatial attention, and the calculation method of spinopelvic parameters based on object detection results. Experiments on the lateral full-spine X-ray dataset show that the proposed X-FEDet achieves 0.951 on AP
50
for the two classes, the first sacral vertebra and the femoral head, in the measurement of pelvic parameters. No significant difference was found between the results of the proposed measurement approach and the manual measurement of orthopedists. Our research suggests that object detection, which can get similar results and shows higher efficiency compared to manual measurement, could be applied in the measurement of spinopelvic parameters. The parameter measurement approach using object detection can potentially reduce the doctors' workload, and assist in the diagnosis of ASD.
更多查看译文
关键词
component,Adult Spinal Deformity,Spinopelvic parameters,Object detection,Spatial attention
AI 理解论文
溯源树
样例
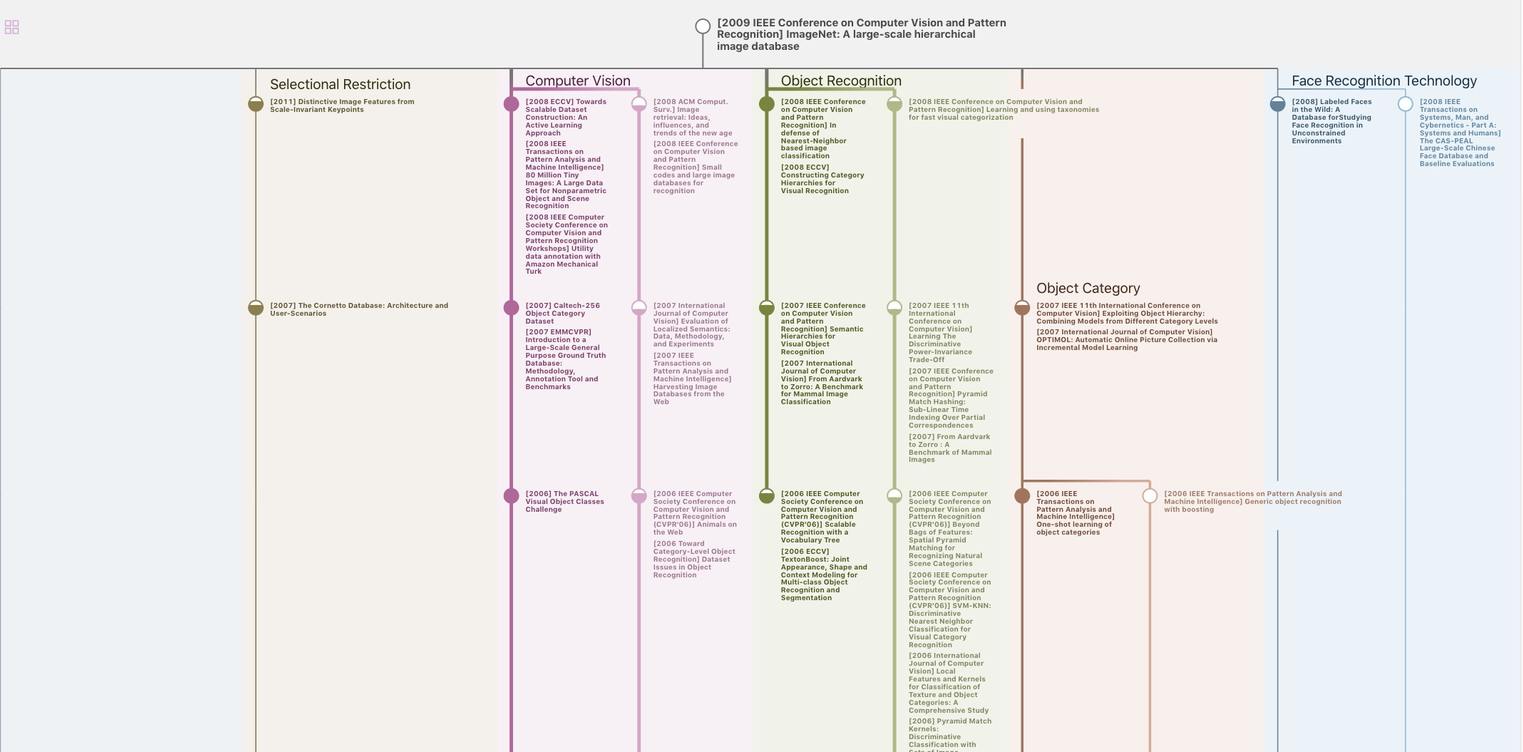
生成溯源树,研究论文发展脉络
Chat Paper
正在生成论文摘要