Automatic and Immediate Detection of Uterine Fibroids Thermally Ablated Regions Using Diffusion-Weighted Imaging and Deep Learning
2023 4th International Seminar on Artificial Intelligence, Networking and Information Technology (AINIT)(2023)
摘要
The automatic intraoperative monitoring of the uterine fibroid ablation treatment area using the Magnetic resonance guided high intensity focused ultrasound system (MRgFUS) remains a significant issue due to time-consuming procedures and inefficient manual segmentation. Dynamic contrast enhanced magnetic resonance imaging (DCE-MRI) is the traditional method and gold standard for evaluating ablation treatment region. But it has the disadvantages of slow imaging and the inability to perform multiple examinations due to the toxicity of the Gadolinium-based contrast agent (GBCA). After analyzing the similarity and consistency of DCE and diffusion weighted imaging (DWI) ablation treatment regions, the segmentation algorithm of the high-intensity focused ultrasound ablation region based on DWI and deep learning is developed to ensure the real-time, effectiveness and safety of ablation region monitoring. Twenty female patients with histologically confirmed uterine fibroids were selected to undergo MRgFUS treatment along with DWI and DCE examination. An end-to-end multimodal image segmentation network DWIseg-net was constructed to segment the ablation regions in DWI, and performed 5-fold cross validation, using non perfusion volume (NPV) in DCE as the gold standard. The proposed DWIseg network directly detects the ablation treatment region on intraoperative DWI, resulting in a DSC of 0.8845, which is superior to previous methods. The ablation treatment rate detected by DWIseg net showed no statistically significant difference compared to the standard NPV (
$p=0.3883$
). The DWIseg network proposed in this study immediately detects the treatment completion region of uterine fibroids treated by MRgFUS, which helps doctors timely adjust the treatment plan during surgery and improve the one-time success rate of surgical treatment.
更多查看译文
关键词
MRgFUS,DWI,Deep learning
AI 理解论文
溯源树
样例
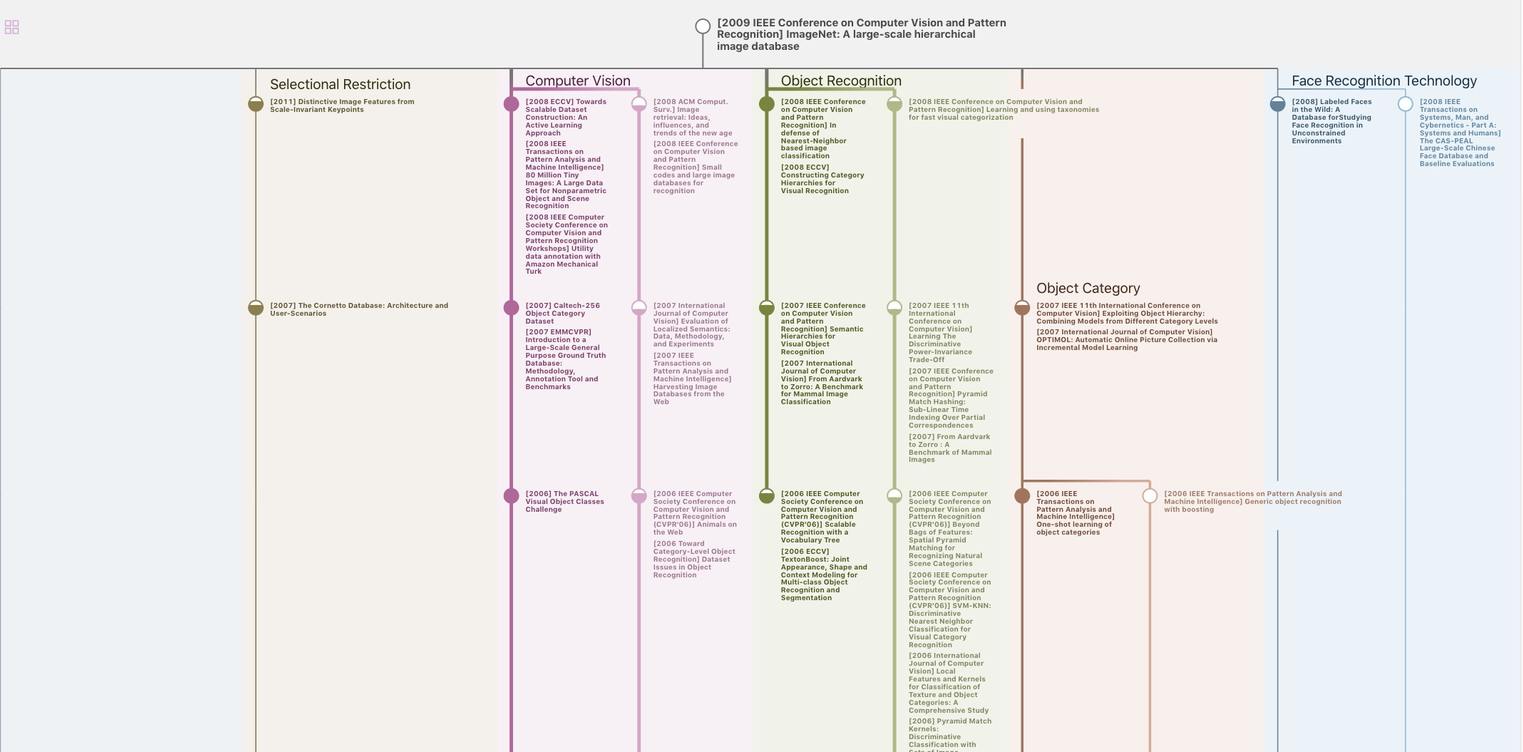
生成溯源树,研究论文发展脉络
Chat Paper
正在生成论文摘要