Machine learning in predicting T-score in the Oxford classification system of IgA nephropathy
Frontiers in immunology(2023)
摘要
BackgroundImmunoglobulin A nephropathy (IgAN) is one of the leading causes of end-stage kidney disease (ESKD). Many studies have shown the significance of pathological manifestations in predicting the outcome of patients with IgAN, especially T-score of Oxford classification. Evaluating prognosis may be hampered in patients without renal biopsy. MethodsA baseline dataset of 690 patients with IgAN and an independent follow-up dataset of 1,168 patients were used as training and testing sets to develop the pathology T-score prediction (T-pre) model based on the stacking algorithm, respectively. The 5-year ESKD prediction models using clinical variables (base model), clinical variables and real pathological T-score (base model plus T-bio), and clinical variables and T-pre (base model plus T-pre) were developed separately in 1,168 patients with regular follow-up to evaluate whether T-pre could assist in predicting ESKD. In addition, an external validation set consisting of 355 patients was used to evaluate the performance of the 5-year ESKD prediction model using T-pre. ResultsThe features selected by AUCRF for the T-pre model included age, systolic arterial pressure, diastolic arterial pressure, proteinuria, eGFR, serum IgA, and uric acid. The AUC of the T-pre was 0.82 (95% CI: 0.80-0.85) in an independent testing set. For the 5-year ESKD prediction model, the AUC of the base model was 0.86 (95% CI: 0.75-0.97). When the T-bio was added to the base model, there was an increase in AUC [from 0.86 (95% CI: 0.75-0.97) to 0.92 (95% CI: 0.85-0.98); P = 0.03]. There was no difference in AUC between the base model plus T-pre and the base model plus T-bio [0.90 (95% CI: 0.82-0.99) vs. 0.92 (95% CI: 0.85-0.98), P = 0.52]. The AUC of the 5-year ESKD prediction model using T-pre was 0.93 (95% CI: 0.87-0.99) in the external validation set. ConclusionA pathology T-score prediction (T-pre) model using routine clinical characteristics was constructed, which could predict the pathological severity and assist clinicians to predict the prognosis of IgAN patients lacking kidney pathology scores.
更多查看译文
关键词
IgA nephropathy,machine learning,Oxford classification system,prediction model,end-stage kidney disease
AI 理解论文
溯源树
样例
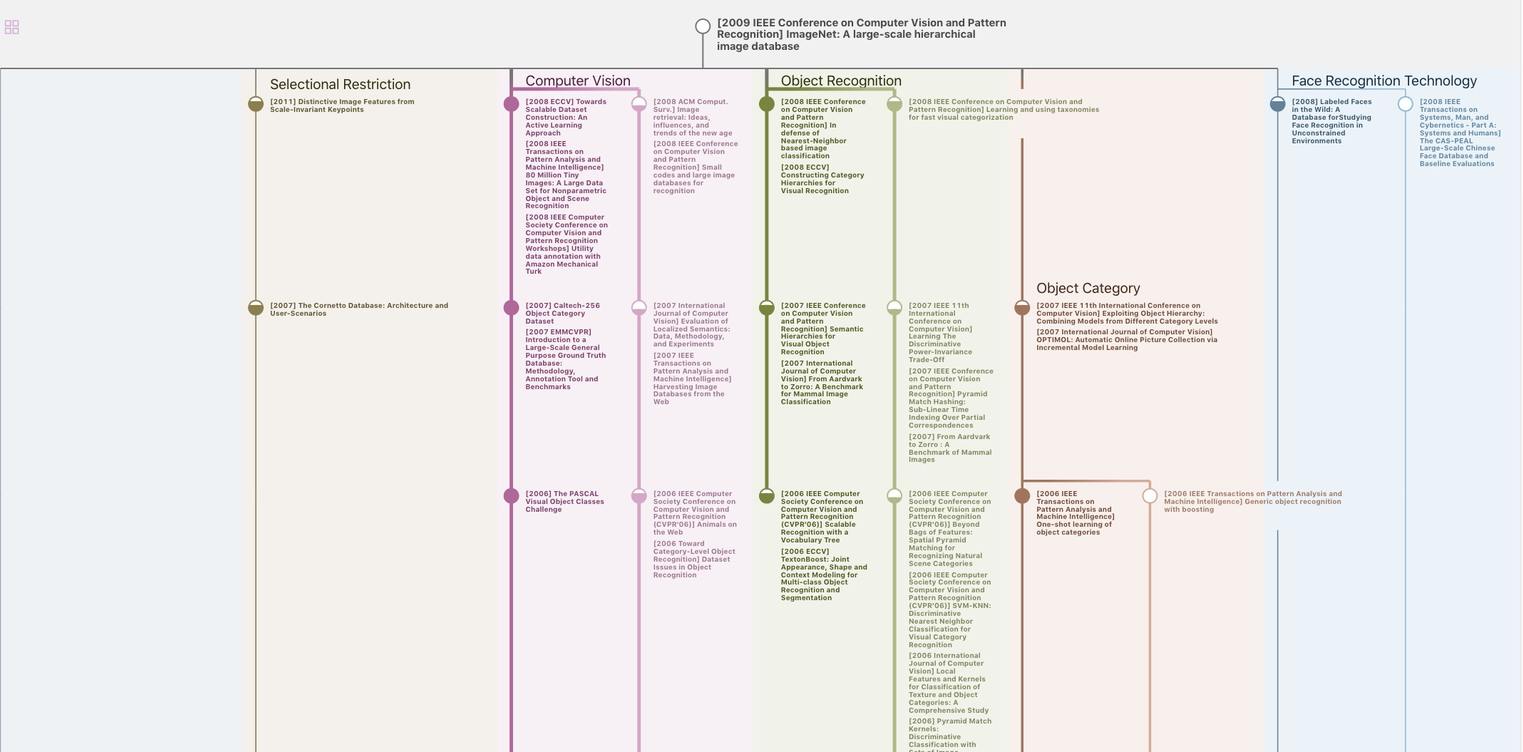
生成溯源树,研究论文发展脉络
Chat Paper
正在生成论文摘要