A threshold longitudinal Tobit quantile regression model for identification of treatment-sensitive subgroups based on interval-bounded longitudinal measurements and a continuous covariate.
Statistics in medicine(2023)
摘要
Identification of a subgroup of patients who may be sensitive to a specific treatment is an important problem in precision medicine. This article considers the case where the treatment effect is assessed by longitudinal measurements, such as quality of life scores assessed over the duration of a clinical trial, and the subset is determined by a continuous baseline covariate, such as age and expression level of a biomarker. Recently, a linear mixed threshold regression model has been proposed but it assumes the longitudinal measurements are normally distributed. In many applications, longitudinal measurements, such as quality of life data obtained from answers to questions on a Likert scale, may be restricted in a fixed interval because of the floor and ceiling effects and, therefore, may be skewed. In this article, a threshold longitudinal Tobit quantile regression model is proposed and a computational approach based on alternating direction method of multipliers algorithm is developed for the estimation of parameters in the model. In addition, a random weighting method is employed to estimate the variances of the parameter estimators. The proposed procedures are evaluated through simulation studies and applications to the data from clinical trials.
更多查看译文
关键词
quantile regression model,longitudinal tobit,continuous covariate
AI 理解论文
溯源树
样例
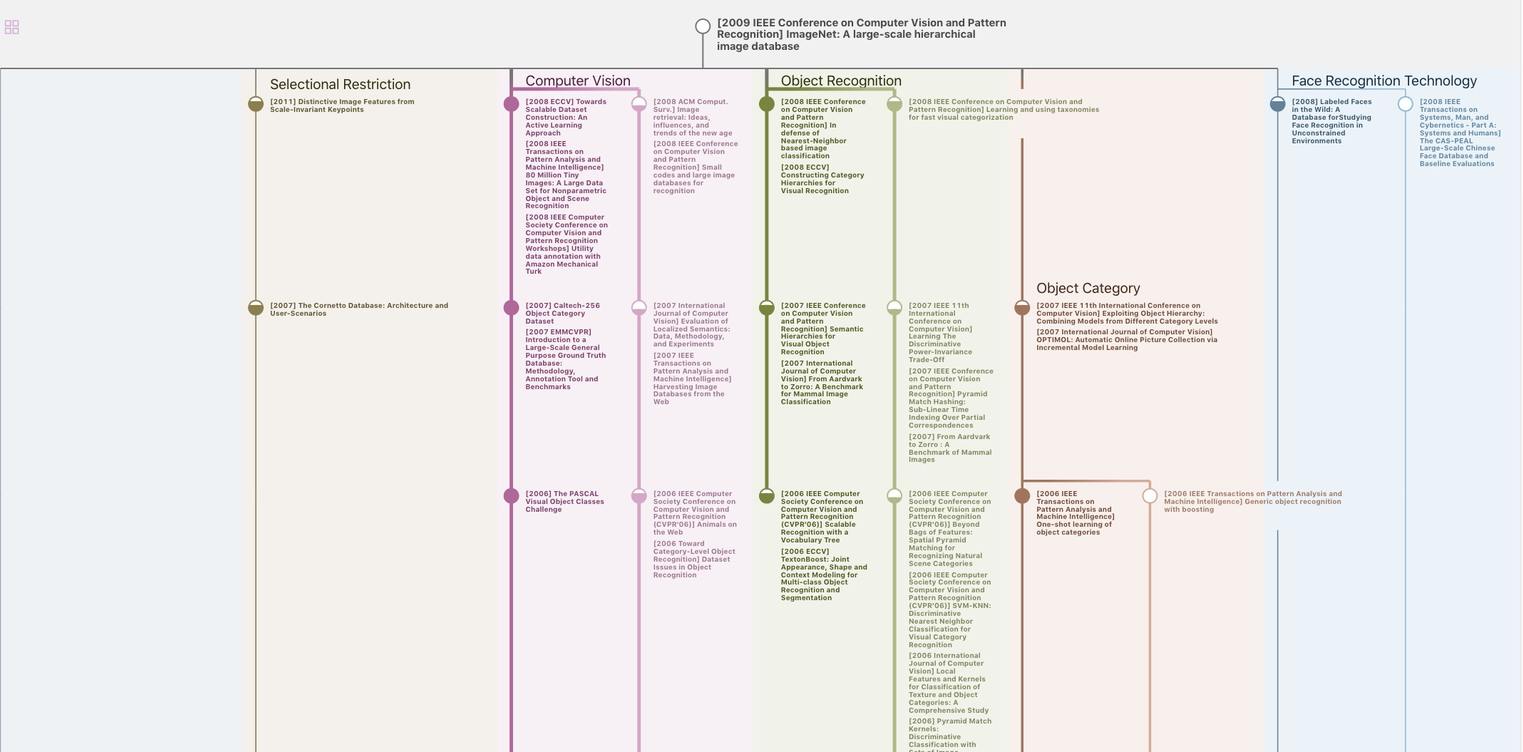
生成溯源树,研究论文发展脉络
Chat Paper
正在生成论文摘要