Important steps for artificial intelligence-based risk assessment of older adults.
The Lancet. Digital health(2023)
摘要
In The Lancet Digital Health, Xiaoli Liu and colleagues1Liu X Hu P Yeung W et al.Illness severity assessment of older adults in critical illness using machine learning (ELDER-ICU): an international multicentre study with subgroup bias evaluation.Lancet Digit Health. 2023; (published online Aug 17.)https://doi.org/10.1016/S2589-7500(23)00128-0Summary Full Text Full Text PDF Google Scholar present a retrospective, international multicentre study that is unique in its comprehensive and generalisable machine learning approach to assessing illness severity of older adults admitted to the intensive care unit. The study developed a machine learning model using relatively large datasets from 14 hospitals in the USA and validated it using 171 hospitals from the USA and Netherlands. Their study design included five steps: development, calibration, validation, subgroup bias evaluation, and explanation. Development included four different machine learning algorithms and handled class imbalance issues. Calibration ensured that the model output better resembled the true probabilities. Internal, external, and temporal validation added to the generalisability of the model. Subgroup analysis identified potential model bias. Finally, the Shapley Additive Explanations method2Lundberg SM Nair B Vavilala MS et al.Explainable machine-learning predictions for the prevention of hypoxaemia during surgery.Nat Biomed Eng. 2018; 2: 749-760Crossref PubMed Scopus (802) Google Scholar increased the interpretability of the model and identified the most significant risk predictors, enabling a simpler model (with fewer risk predictors) to be developed, which demonstrated comparable performance. The study makes several important contributions to the literature. Each of these five steps is important, but few previous studies include all steps. This study handled all five steps well, which is what makes it valuable and of high impact. The study design is well thought out and is hereby recommended for future machine learning studies to follow to develop robust, generalisable machine learning models. Although the study by Liu and colleagues1Liu X Hu P Yeung W et al.Illness severity assessment of older adults in critical illness using machine learning (ELDER-ICU): an international multicentre study with subgroup bias evaluation.Lancet Digit Health. 2023; (published online Aug 17.)https://doi.org/10.1016/S2589-7500(23)00128-0Summary Full Text Full Text PDF Google Scholar takes important steps towards artificial intelligence-based risk assessment of older adults, there are still issues that are important to handle to bridge the gap from a useful machine learning model to actual use in clinical practice. To take the next steps, Liu and colleagues need to focus more on the clinical relevance and utility of the study. Studies should aim to add value to clinical practice, and they should be initiated based on a particular clinical need, idea, challenge, or problem. Unfortunately, many studies start with a dataset and develop a machine learning model to present some findings, and only later try to identify possible clinical relevance. The latter approach is less likely to make it into clinical use. Additionally, the study needs to have a clear clinical workflow and context in mind in which the machine learning model should work. This is why stakeholder involvement should play a crucial role in all five steps mentioned above. Development of future use case scenarios could be one way of envisioning how a new machine learning model can fit into and add value to current clinical practice; for example, by providing a second opinion on a patient diagnosis, flagging a potential high-risk patient, or providing a prognosis for a patient's disease trajectory. Developing a well performing machine learning model is just a first step. The next step is to wrap the model in a decision support system (DSS) that is easy to use, provides interpretable output, and is well integrated into clinical practice and existing health IT systems. A holistic, interdisciplinary approach is required to develop useful and usable machine learning models and DSS. Many studies like that of Liu and colleagues1Liu X Hu P Yeung W et al.Illness severity assessment of older adults in critical illness using machine learning (ELDER-ICU): an international multicentre study with subgroup bias evaluation.Lancet Digit Health. 2023; (published online Aug 17.)https://doi.org/10.1016/S2589-7500(23)00128-0Summary Full Text Full Text PDF Google Scholar are retrospective and leave the prospective validation in clinical practice to future work. Previous work has demonstrated that the step from a retrospective study to a prospective study3Fournaise A Espensen N Jakobsen A Andersen-Ranberg K Increasing primary health-care services are associated with acute short-term hospitalization of Danes aged 70 years and older.Eur Geriatr Med. 2017; 8: 435-439Crossref Scopus (7) Google Scholar, 4Fournaise A Lauridsen JT Nissen SK et al.Structured decision support to prevent hospitalisations of community-dwelling older adults in Denmark (PATINA): an open-label, stepped-wedge, cluster-randomised controlled trial.Lancet Healthy Longev. 2023; 4: e132-e142Summary Full Text Full Text PDF PubMed Google Scholar can be tedious and challenging. Due to legal, IT system, or other issues, it may be difficult to obtain real-time access to the same data for the prospective study that were used in the retrospective study. Hence, machine learning models may have to be completely rebuilt. It is also important to keep in mind who the DSS is intended for (ie, patient, relative, home carer, nurse, general practitioner, or specialist doctor) so as to design, test, and evaluate it accordingly, involving the relevant stakeholders. Finally, experience from a prospective study running in three Danish municipalities4Fournaise A Lauridsen JT Nissen SK et al.Structured decision support to prevent hospitalisations of community-dwelling older adults in Denmark (PATINA): an open-label, stepped-wedge, cluster-randomised controlled trial.Lancet Healthy Longev. 2023; 4: e132-e142Summary Full Text Full Text PDF PubMed Google Scholar revealed local specifics that affect the usability of the system and different human preferences. This points towards DSS solutions that are configurable to accommodate different user and usage needs and preferences, such as different threshold values that trigger DSS actions and alerts. This underlines the importance of having a clear clinical workflow and context in mind, and designing the entire path from retrospective study to prospective study and beyond from the beginning. The study by Liu and colleagues1Liu X Hu P Yeung W et al.Illness severity assessment of older adults in critical illness using machine learning (ELDER-ICU): an international multicentre study with subgroup bias evaluation.Lancet Digit Health. 2023; (published online Aug 17.)https://doi.org/10.1016/S2589-7500(23)00128-0Summary Full Text Full Text PDF Google Scholar and other recent studies5Placido D Yuan B Hjaltelin JX et al.A deep learning algorithm to predict risk of pancreatic cancer from disease trajectories.Nat Med. 2023; 29: 1113-1122Crossref PubMed Scopus (6) Google Scholar, 6Thorsen-Meyer HC Placido D Kaas-Hansen BS et al.Discrete-time survival analysis in the critically ill: a deep learning approach using heterogeneous data.NPJ Digit Med. 2022; 5: 142Crossref PubMed Scopus (4) Google Scholar fit well into the current research practice of high-impact studies that, in addition to model development, have an increased focus on aspects of calibration of models, assessment of bias, external validation to increase generalisability, and explainability to increase interpretability using tools like the Shapley Additive Explanations method.2Lundberg SM Nair B Vavilala MS et al.Explainable machine-learning predictions for the prevention of hypoxaemia during surgery.Nat Biomed Eng. 2018; 2: 749-760Crossref PubMed Scopus (802) Google Scholar These studies clearly demonstrate the potential of using artificial intelligence in clinical practice. Ultimately, the advancements made by these studies will generate enough momentum and interest to bridge the challenging gap from retrospective machine learning models to DSS in clinical use. I declare no competing interests. Illness severity assessment of older adults in critical illness using machine learning (ELDER-ICU): an international multicentre study with subgroup bias evaluationThe ELDER-ICU model reliably predicts the risk of in-hospital mortality using routinely collected clinical features. The predictions could inform clinicians about patients who are at elevated risk of deterioration. Prospective validation of this model in clinical practice and a process for continuous performance monitoring and model recalibration are needed. Full-Text PDF Open Access
更多查看译文
关键词
risk assessment,older adults,intelligence-based
AI 理解论文
溯源树
样例
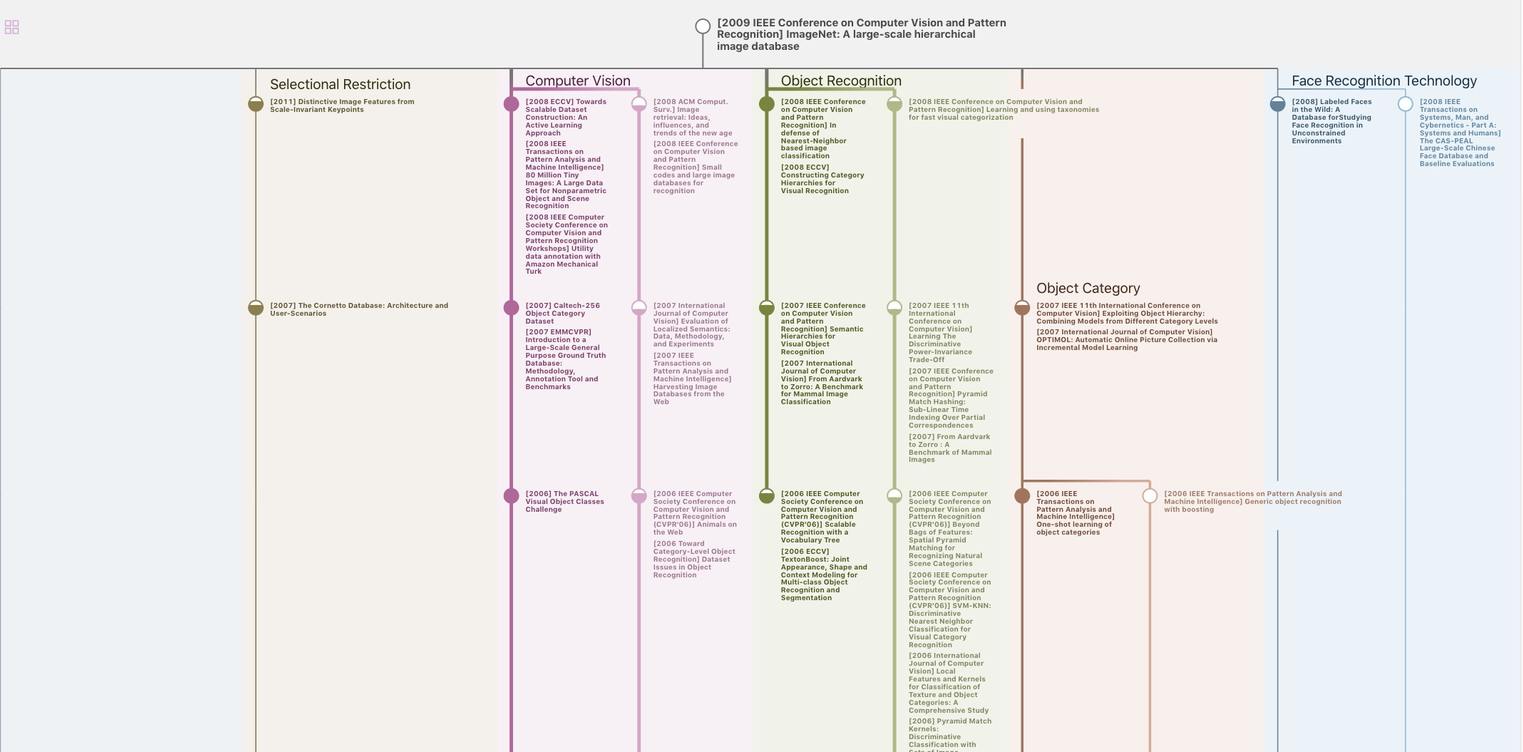
生成溯源树,研究论文发展脉络
Chat Paper
正在生成论文摘要