Bayesian optimal experimental design for constitutive model calibration
INTERNATIONAL JOURNAL OF MECHANICAL SCIENCES(2024)
Abstract
Computational simulation is increasingly relied upon for high/consequence engineering decisions, which necessitates a high confidence in the calibration of and predictions from complex material models. However, the calibration and validation of material models is often a discrete, multi-stage process that is decoupled from material characterization activities, which means the data collected does not always align with the data that is needed. To address this issue, an integrated workflow for delivering an enhanced characterization and cal-ibration procedure-Interlaced Characterization and Calibration (ICC)-is introduced and demonstrated. This framework leverages Bayesian optimal experimental design (BOED), which creates a line of communication between model calibration needs and data collection capabilities in order to optimize the information content gathered from the experiments for model calibration. Eventually, the ICC framework will be used in quasi real-time to actively control experiments of complex specimens for the calibration of a high-fidelity material model. This work presents the critical first piece of algorithm development and a demonstration in determining the optimal load path of a cruciform specimen with simulated data. Calibration results, obtained via Bayesian inference, from the integrated ICC approach are compared to calibrations performed by choosing the load path a priori based on human intuition, as is traditionally done. The calibration results are communicated through parameter uncertainties which are propagated to the model output space (i.e. stress-strain). In these exemplar problems, data generated within the ICC framework resulted in calibrated model parameters with reduced measures of uncertainty compared to the traditional approaches.
MoreTranslated text
Key words
Bayesian optimal experimental design,Bayesian inference,Constitutive model calibration,Expected information gain,Hill48,Plasticity
AI Read Science
Must-Reading Tree
Example
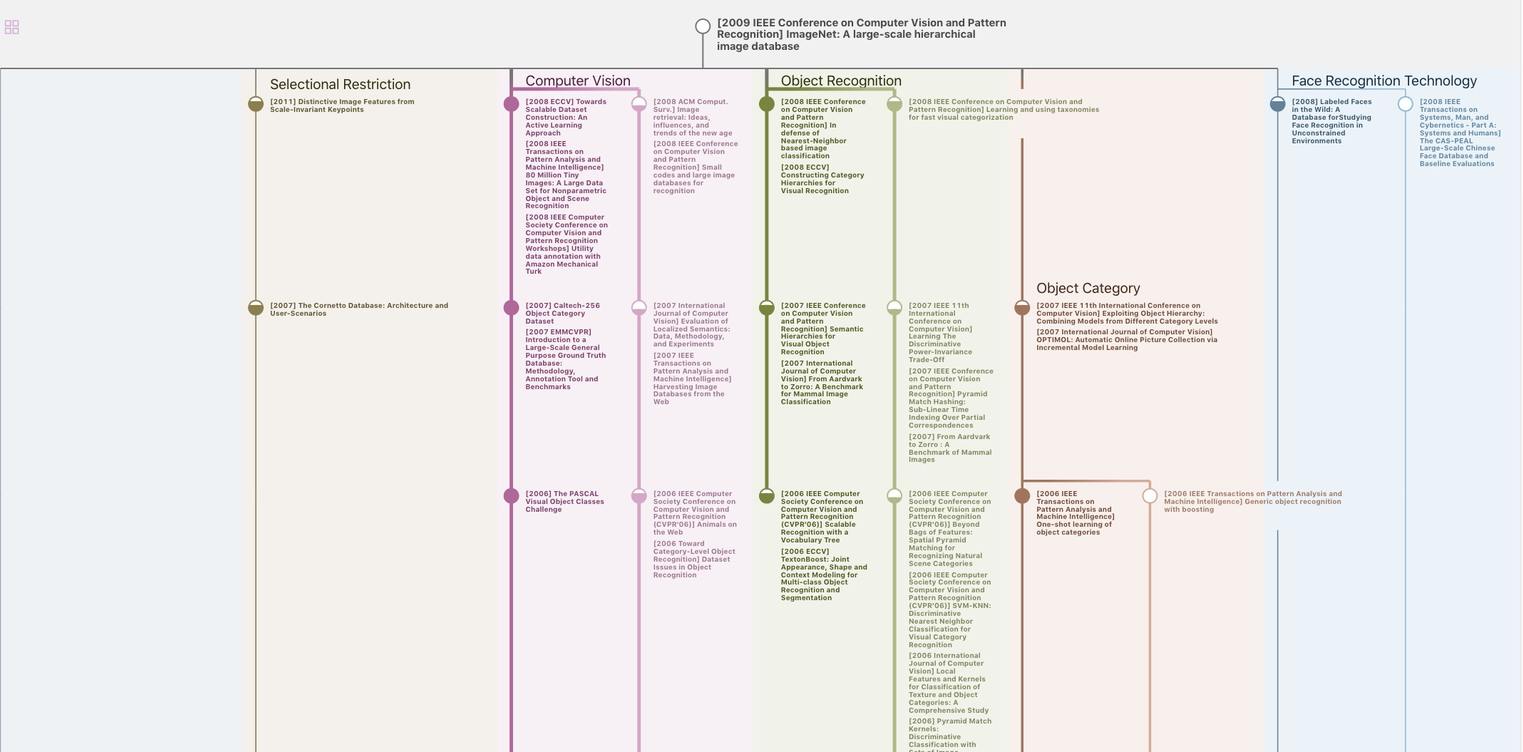
Generate MRT to find the research sequence of this paper
Chat Paper
Summary is being generated by the instructions you defined