Beyond expectations: Residual Dynamic Mode Decomposition and Variance for Stochastic Dynamical Systems
NONLINEAR DYNAMICS(2023)
摘要
Koopman operators linearize nonlinear dynamical systems, making their spectral information of crucial interest. Numerous algorithms have been developed to approximate these spectral properties, and Dynamic Mode Decomposition (DMD) stands out as the poster child of projection-based methods. Although the Koopman operator itself is linear, the fact that it acts in an infinite-dimensional space of observables poses various challenges. These include spurious modes, essential spectra, and the verification of Koopman mode decompositions. While recent work has addressed these challenges for deterministic systems, there remains a notable gap in verified DMD methods tailored for stochastic systems, where the Koopman operator measures the expectation of observables. We show that it is necessary to go beyond expectations to address these issues. By incorporating variance into the Koopman framework, we address these challenges. Through an additional DMD-type matrix, we approximate the sum of a squared residual and a variance term, each of which can be approximated individually using batched snapshot data. This allows verified computation of the spectral properties of stochastic Koopman operators, controlling the projection error. We also introduce the concept of variance-pseudospectra to gauge statistical coherency. Finally, we present a suite of convergence results for the spectral quantities of stochastic Koopman operators. Our study concludes with practical applications using both simulated and experimental data. In neural recordings from awake mice, we demonstrate how variance-pseudospectra can reveal physiologically significant information unavailable to standard expectation-based dynamical models.
更多查看译文
关键词
Dynamical systems,Koopman operator,Data-driven discovery,Dynamic mode decomposition,Spectral theory,Error bounds,Stochastic systems
AI 理解论文
溯源树
样例
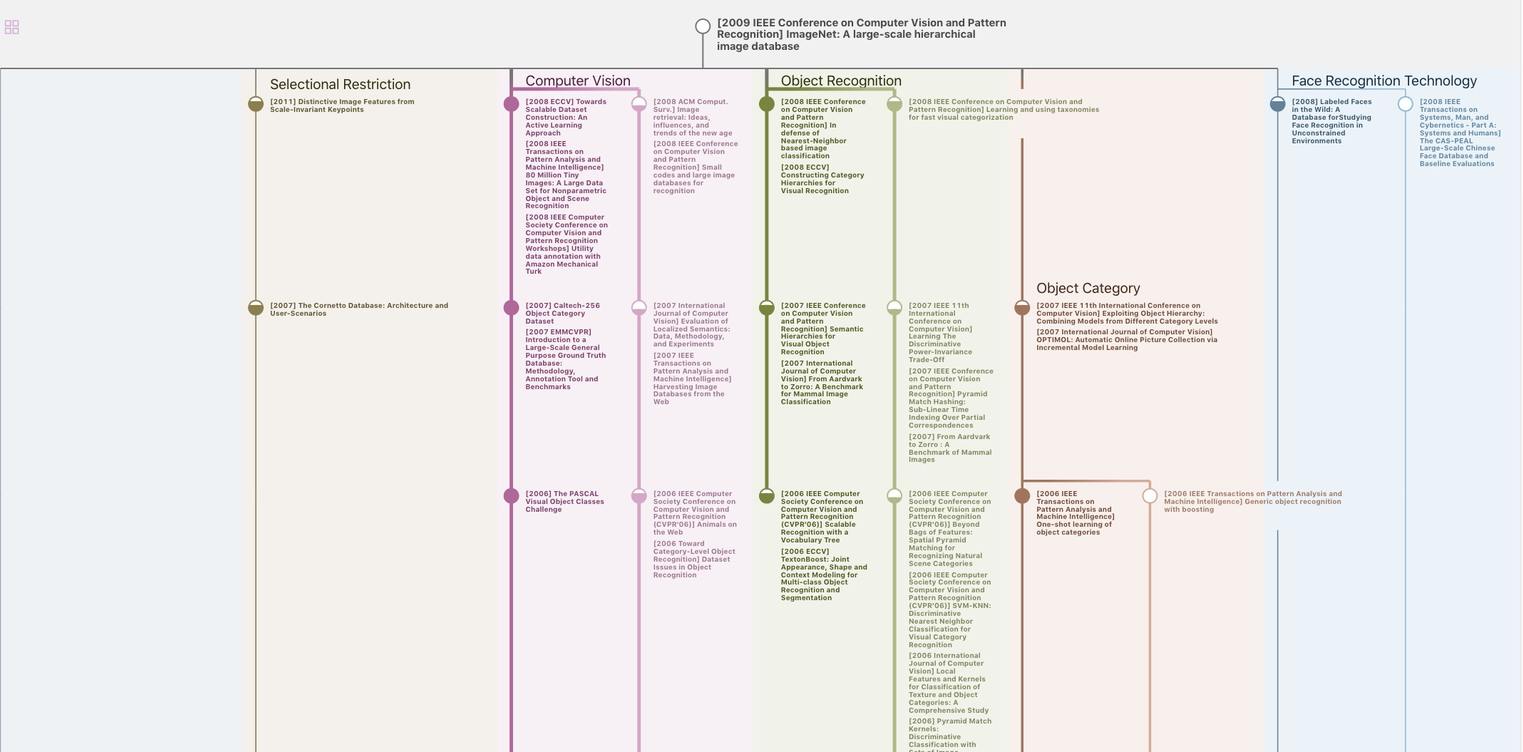
生成溯源树,研究论文发展脉络
Chat Paper
正在生成论文摘要