Intrusion Detection based on Federated Learning: a systematic review
CoRR(2023)
摘要
The evolution of cybersecurity is undoubtedly associated and intertwined with the development and improvement of artificial intelligence (AI). As a key tool for realizing more cybersecure ecosystems, Intrusion Detection Systems (IDSs) have evolved tremendously in recent years by integrating machine learning (ML) techniques for the detection of increasingly sophisticated cybersecurity attacks hidden in big data. However, these approaches have traditionally been based on centralized learning architectures, in which data from end nodes are shared with data centers for analysis. Recently, the application of federated learning (FL) in this context has attracted great interest to come up with collaborative intrusion detection approaches where data does not need to be shared. Due to the recent rise of this field, this work presents a complete, contemporary taxonomy for FL-enabled IDS approaches that stems from a comprehensive survey of the literature in the time span from 2018 to 2022. Precisely, our discussion includes an analysis of the main ML models, datasets, aggregation functions, as well as implementation libraries, which are employed by the proposed FL-enabled IDS approaches. On top of everything else, we provide a critical view of the current state of the research around this topic, and describe the main challenges and future directions based on the analysis of the literature and our own experience in this area.
更多查看译文
关键词
federated learning
AI 理解论文
溯源树
样例
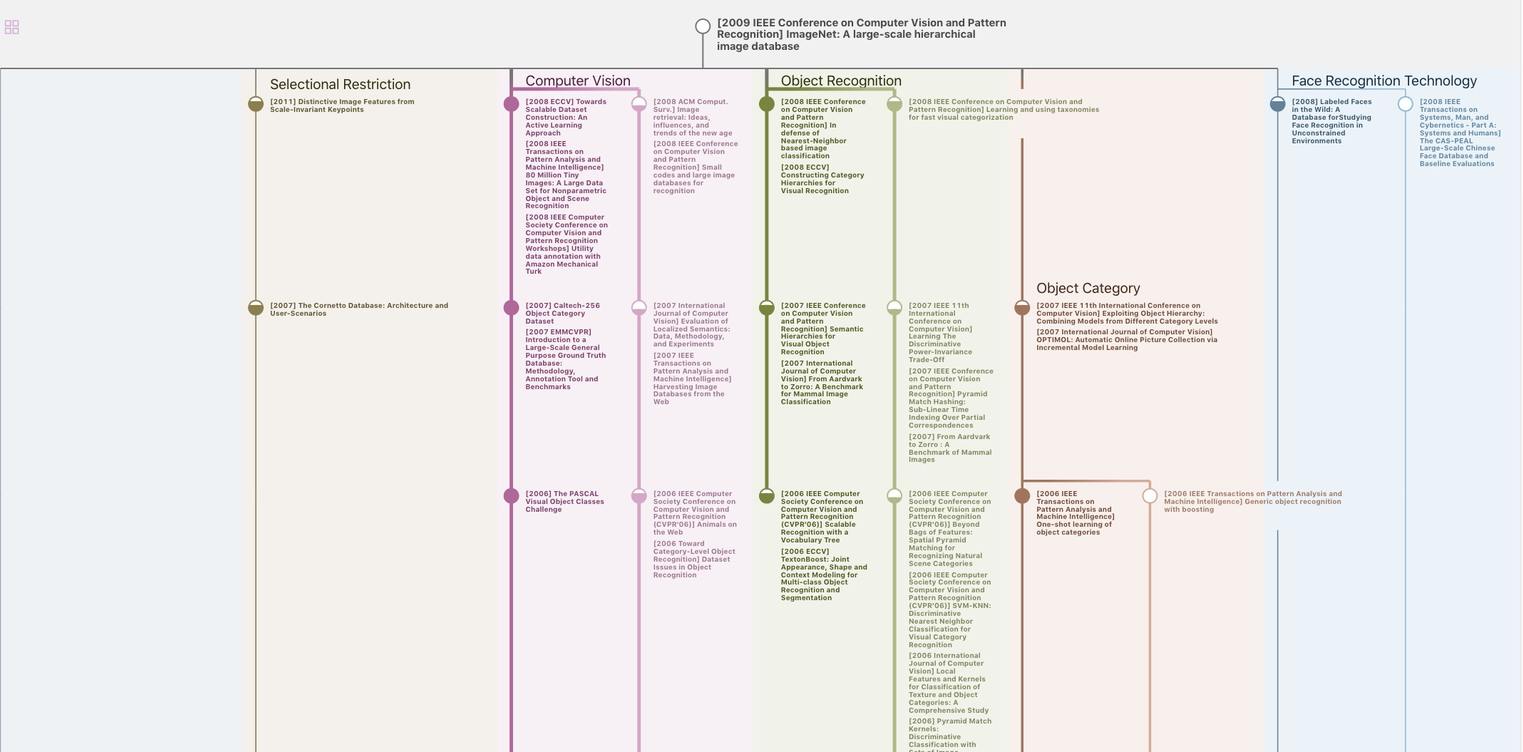
生成溯源树,研究论文发展脉络
Chat Paper
正在生成论文摘要