Decoupled conditional contrastive learning with variable metadata for prostate lesion detection
CoRR(2023)
摘要
Early diagnosis of prostate cancer is crucial for efficient treatment. Multi-parametric Magnetic Resonance Images (mp-MRI) are widely used for lesion detection. The Prostate Imaging Reporting and Data System (PI-RADS) has standardized interpretation of prostate MRI by defining a score for lesion malignancy. PI-RADS data is readily available from radiology reports but is subject to high inter-reports variability. We propose a new contrastive loss function that leverages weak metadata with multiple annotators per sample and takes advantage of inter-reports variability by defining metadata confidence. By combining metadata of varying confidence with unannotated data into a single conditional contrastive loss function, we report a 3% AUC increase on lesion detection on the public PI-CAI challenge dataset. Code is available at: https://github.com/camilleruppli/decoupled_ccl
更多查看译文
关键词
conditional contrastive learning,detection,variable metadata
AI 理解论文
溯源树
样例
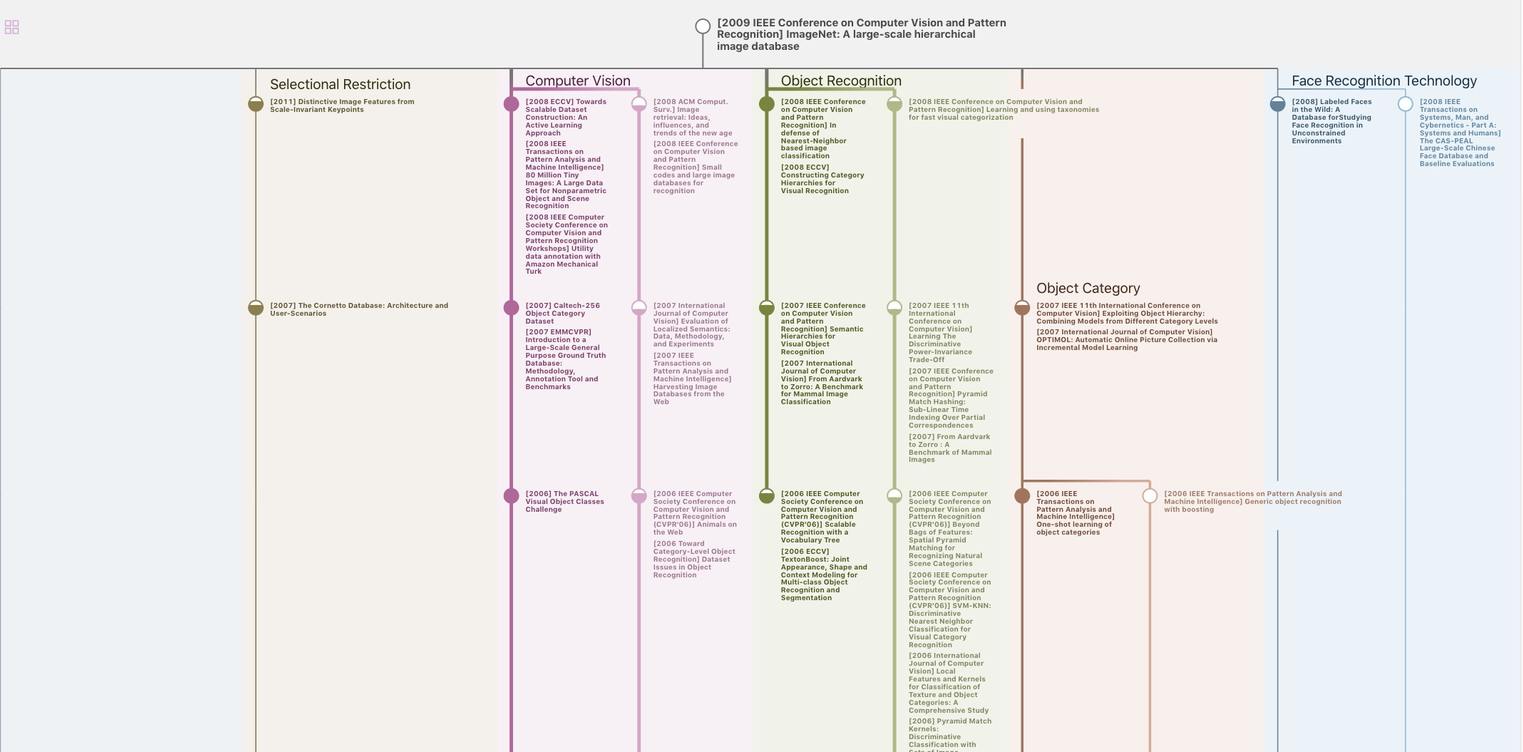
生成溯源树,研究论文发展脉络
Chat Paper
正在生成论文摘要