Boosting Few-shot Action Recognition with Graph-guided Hybrid Matching
CoRR(2023)
摘要
Class prototype construction and matching are core aspects of few-shot action recognition. Previous methods mainly focus on designing spatiotemporal relation modeling modules or complex temporal alignment algorithms. Despite the promising results, they ignored the value of class prototype construction and matching, leading to unsatisfactory performance in recognizing similar categories in every task. In this paper, we propose GgHM, a new framework with Graph-guided Hybrid Matching. Concretely, we learn task-oriented features by the guidance of a graph neural network during class prototype construction, optimizing the intra- and inter-class feature correlation explicitly. Next, we design a hybrid matching strategy, combining frame-level and tuple-level matching to classify videos with multivariate styles. We additionally propose a learnable dense temporal modeling module to enhance the video feature temporal representation to build a more solid foundation for the matching process. GgHM shows consistent improvements over other challenging baselines on several few-shot datasets, demonstrating the effectiveness of our method. The code will be publicly available at https://github.com/jiazheng-xing/GgHM.
更多查看译文
关键词
action,hybrid matching,recognition,few-shot,graph-guided
AI 理解论文
溯源树
样例
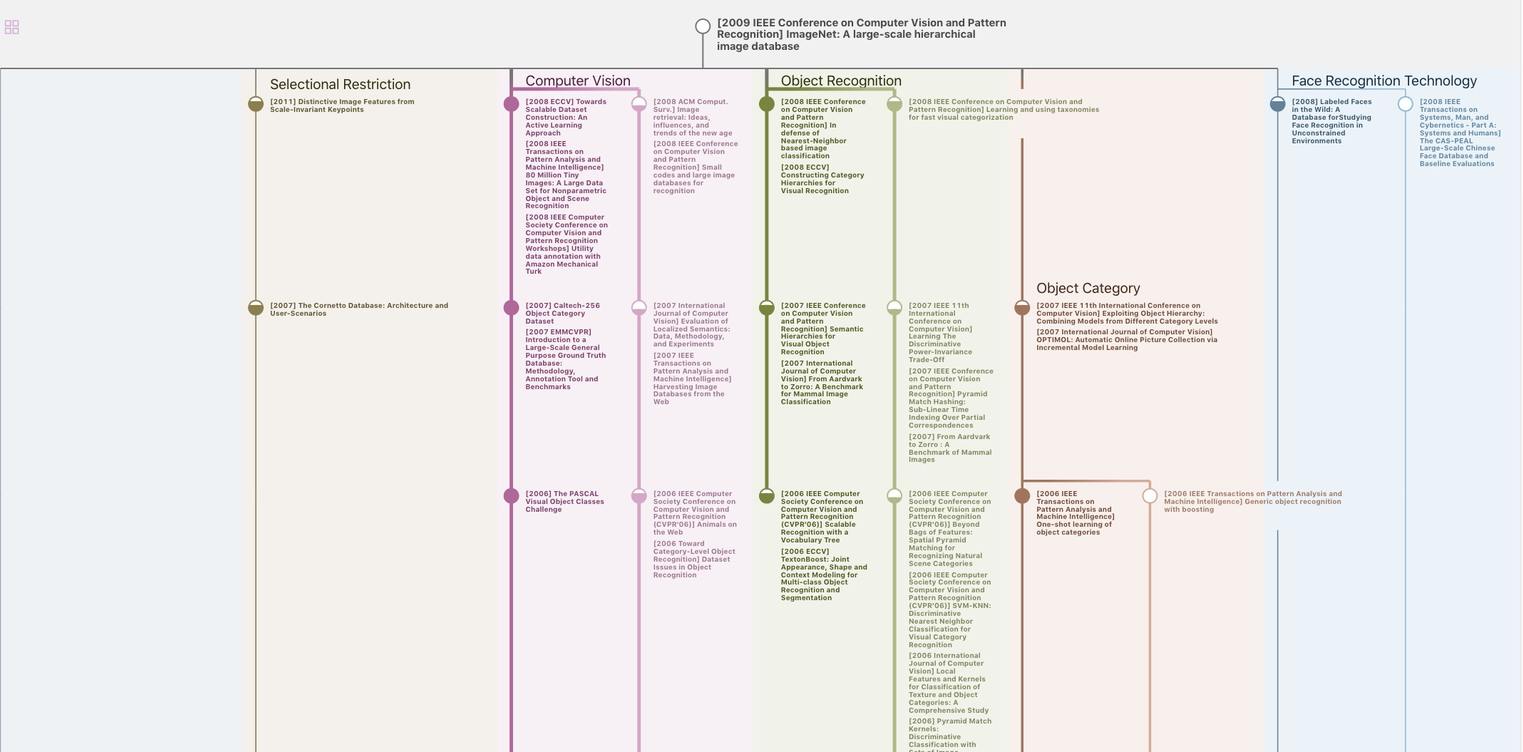
生成溯源树,研究论文发展脉络
Chat Paper
正在生成论文摘要