Scalable Lattice Sampling using Factorized Generative Models
arXiv (Cornell University)(2023)
摘要
Boltzmann distributions over lattices are pervasive in Computational Physics. Sampling them becomes increasingly difficult with the increase in the number of dimensions, especially near critical regions, e.g., phase transitions or continuum limits. Conditional generative models are emerging as promising tools for sampling in critical regions. When conditioned on the parameters to be varied, they can be efficaciously extended to critical regions without the need for retraining. However, current approaches do not scale well to large lattices. We present a novel approach called Parallelizable Block Metropolis-within-Gibbs (PBMG) for generating samples for any local lattice model. It factorizes the joint distribution of lattice into local parametric kernels, thereby allowing efficient sampling of very large lattices. We optimize the model with reverse Kullback-Leibler divergence (RKLD) to avoid the need for ground truth samples. Since the local distributions are simpler, the model is not affected by mode collapse, which generally occurs while training with RKLD. We validate our approach on the XY model and the Scalar $\phi^4$ theory. PBMG achieves high acceptance rates and the observable statistics estimated from the samples match the ground truth.
更多查看译文
关键词
scalable lattice sampling,models
AI 理解论文
溯源树
样例
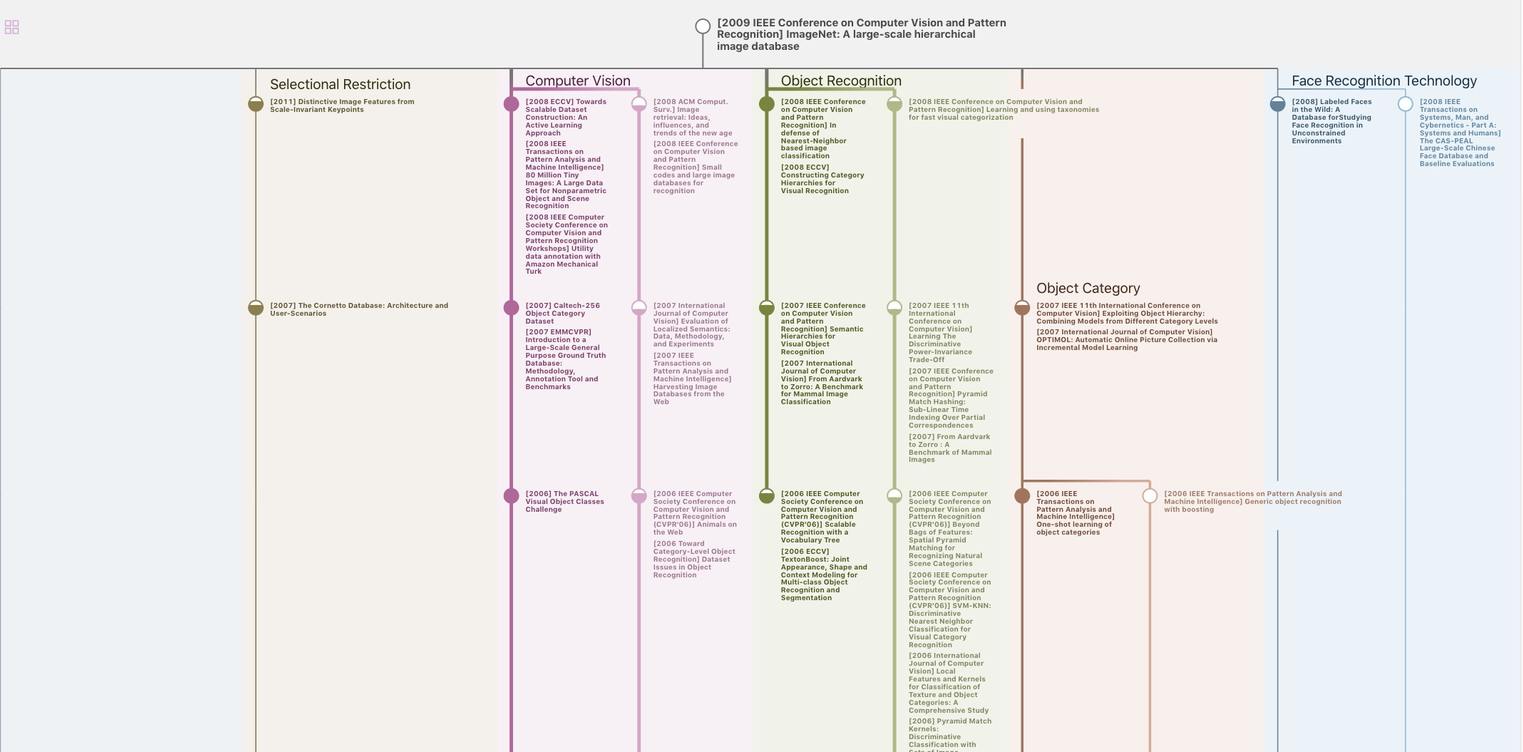
生成溯源树,研究论文发展脉络
Chat Paper
正在生成论文摘要