Recurrent Neural Networks with more flexible memory: better predictions than rough volatility
CoRR(2023)
摘要
We extend recurrent neural networks to include several flexible timescales for each dimension of their output, which mechanically improves their abilities to account for processes with long memory or with highly disparate time scales. We compare the ability of vanilla and extended long short term memory networks (LSTMs) to predict asset price volatility, known to have a long memory. Generally, the number of epochs needed to train extended LSTMs is divided by two, while the variation of validation and test losses among models with the same hyperparameters is much smaller. We also show that the model with the smallest validation loss systemically outperforms rough volatility predictions by about 20% when trained and tested on a dataset with multiple time series.
更多查看译文
关键词
rough volatility,more flexible memory,neural networks,better predictions
AI 理解论文
溯源树
样例
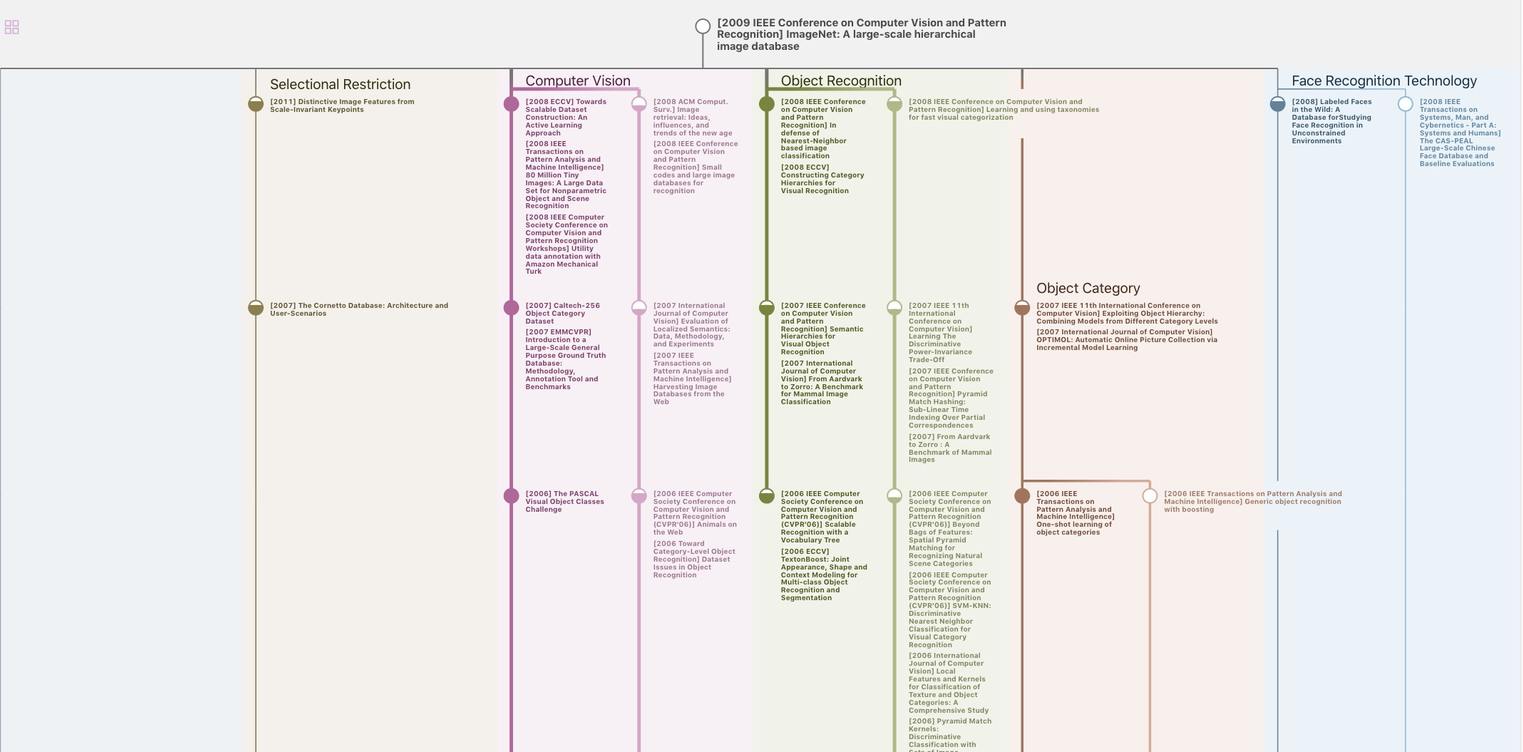
生成溯源树,研究论文发展脉络
Chat Paper
正在生成论文摘要