Evaluation of different classification methods using electronic nose data to diagnose sarcoidosis.
Journal of breath research(2023)
摘要
Electronic nose (eNose) technology is an emerging diagnostic application, using artificial intelligence (AI) to classify human breath patterns. These patterns can be used to diagnose medical conditions. Sarcoidosis is an often difficult to diagnose disease, as no standard procedure or conclusive test exists. An accurate diagnostic model based on eNose data could therefore be helpful in clinical decision making.
Aim: The aim of this paper is to evaluate the performance of various dimensionality reduction methods and classifiers in order to design an accurate diagnostic model for sarcoidosis.
Methods: Various methods of dimensionality reduction and multiple hyperparameter optimised classifiers were tested and cross-validated on a dataset of patients with pulmonary sarcoidosis (n=224) and other interstitial lung disease (n=317). Best performing methods were selected to create a model to diagnose patients with sarcoidosis. Nested cross-validation was applied to calculate the overall diagnostic performance.
Results: A classification model with feature selection and random forest classifier showed the highest accuracy. The overall diagnostic performance resulted in an accuracy of 87.1% and area-under-the-curve of 91.2%.
Conclusion: After comparing different dimensionality reduction methods and classifiers, a highly accurate model to diagnose a patient with sarcoidosis using eNose data was created. The random forest classifier and feature selection showed the best performance. The presented systematic approach could also be applied to other eNose datasets to compare methods and select the optimal diagnostic model.
更多查看译文
关键词
electronic nose data,different classification methods
AI 理解论文
溯源树
样例
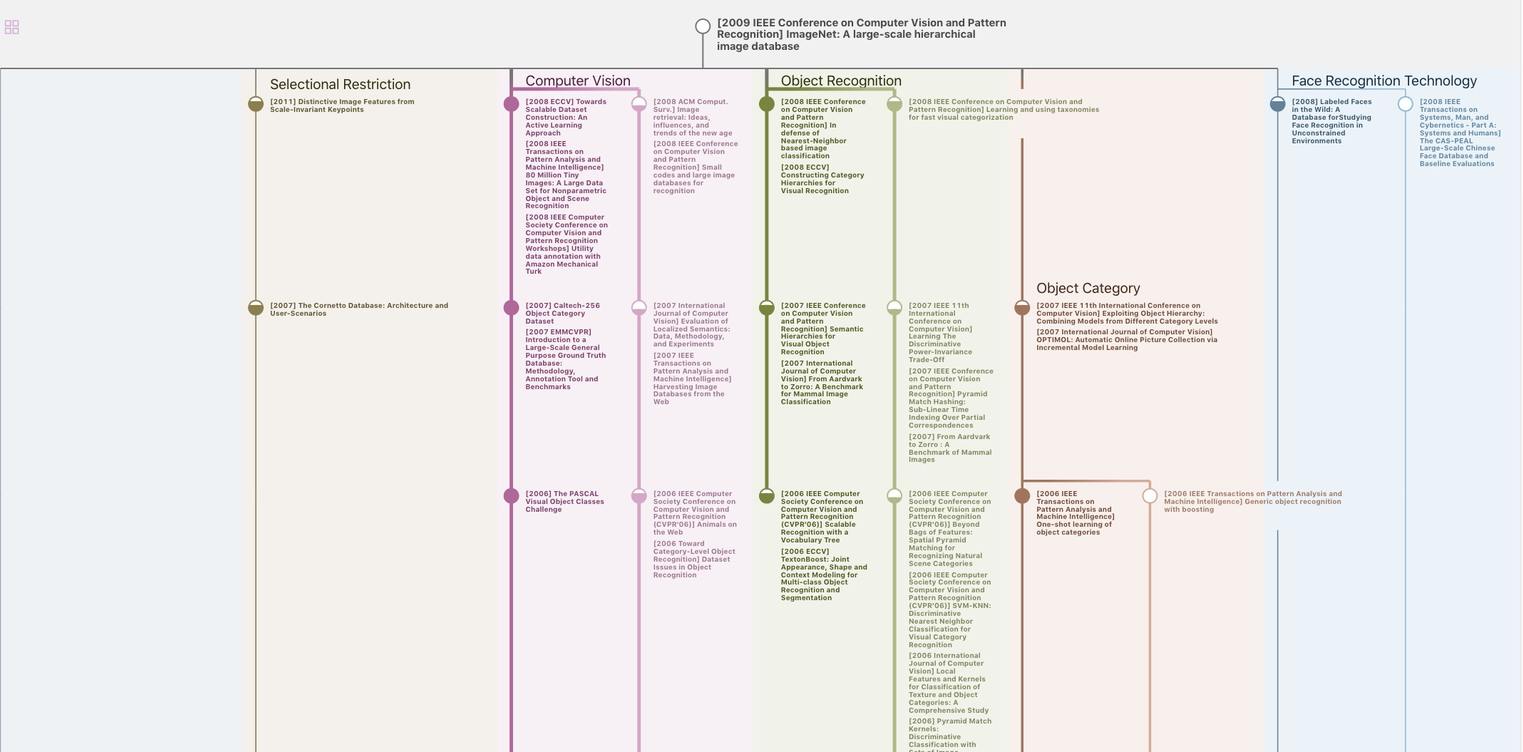
生成溯源树,研究论文发展脉络
Chat Paper
正在生成论文摘要