Fair comparisons of cause-specific and relative survival by accounting for the systematic removal of patients from risk-sets.
Cancer epidemiology(2023)
摘要
BACKGROUND:In population-based cancer studies it is common to try to isolate the impact of cancer by estimating net survival. Net survival is defined as the probability of surviving cancer in the absence of any other-causes of death. Net survival can be estimated either in the cause-specific or relative survival framework. Cause-specific survival considers deaths from the cancer as the event of interest. Relative survival incorporates general population expected mortality rates to represent the other-cause mortality rate. Estimation approaches in both frameworks are impacted by the systematic removal of patients from the risk-set, commonly referred to as informative censoring in the cause-specific framework. In the relative survival framework, the Pohar Perme estimator combats the effect of this systematic removal of patients through weighting. When the two frameworks have been compared, informative censoring is rarely accounted for in the cause-specific framework.
METHODS:We investigate the use of weighted cause-specific Kaplan-Meier estimates to overcome the impact of informative censoring and compared approaches to defining weights. Individuals remaining in the risk-set are upweighted using their predicted other-cause survival obtained through various model-based approaches. We also compare weights derived from expected mortality rates. We applied the approaches to US cancer registry data and conducted a simulation study.
RESULTS:Using weighted cause-specific estimates provides a better estimate of marginal net survival. The unweighted Kaplan-Meier estimates have a similar bias to the Ederer II method for relative survival. Weighted Kaplan-Meier estimates are unbiased and similar to the Pohar Perme estimator. There was little variation between the several weighting approaches.
CONCLUSION:In comparisons of cause-specific and relative survival, it is important to compare "like-with-like", therefore, a weighted approach should be considered for both frameworks. If researchers are interested in obtaining net measures in a cause-specific framework, then weighting is needed to account for informative censoring.
更多查看译文
AI 理解论文
溯源树
样例
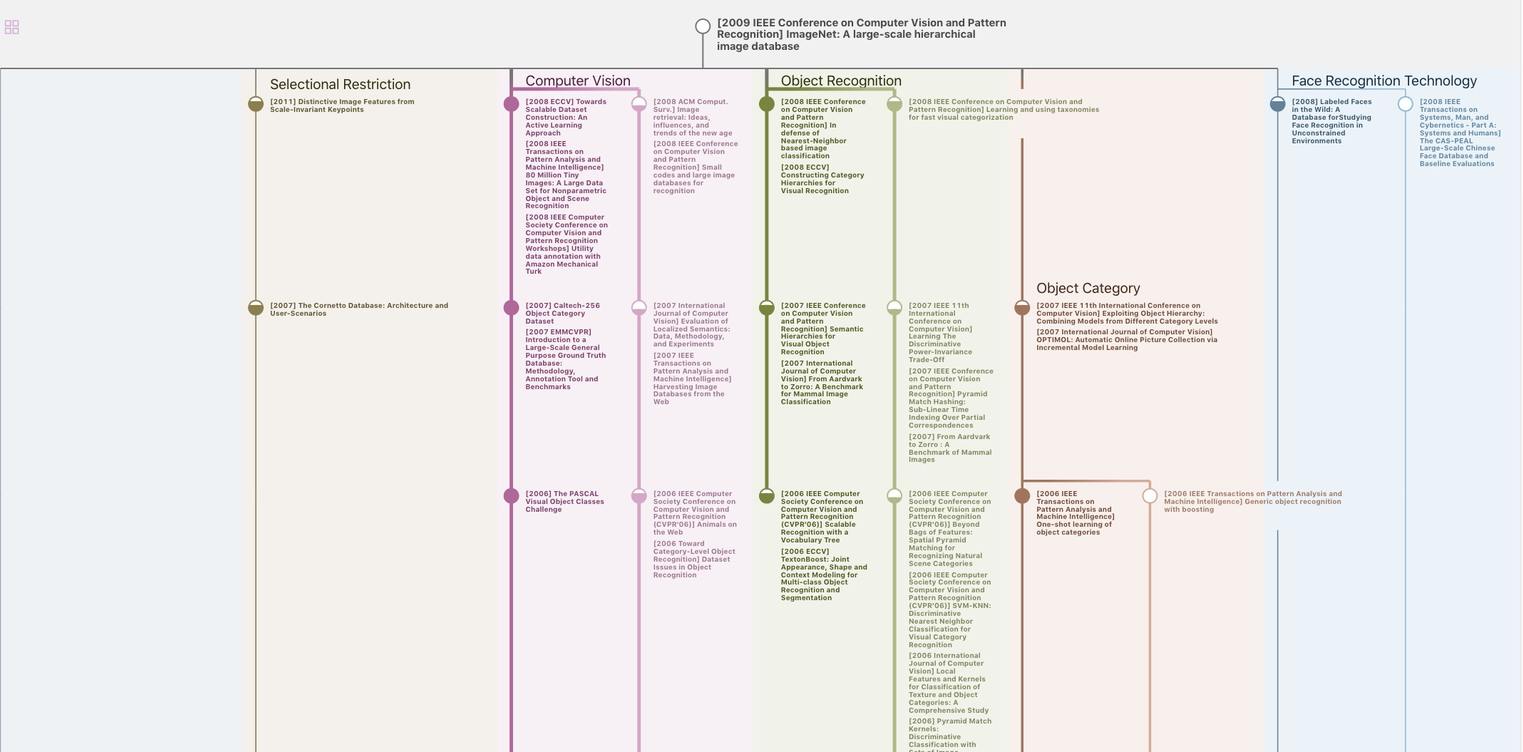
生成溯源树,研究论文发展脉络
Chat Paper
正在生成论文摘要