High-throughput generic single-entity sequencing using droplet microfluidics
bioRxiv (Cold Spring Harbor Laboratory)(2023)
摘要
Single-cell sequencing has revolutionized our understanding of cellular heterogeneity by providing a micro-level perspective over the past decades. Although heterogeneity is essential for various biological communities, the currently demonstrated platform predominantly focuses on eukaryotic cells without cell walls and their transcriptomics [1][1],[2][2], leaving significant gaps in the study of omics from other single biological entities such as bacteria and viruses. Due to the difficulty of isolating and acquiring their DNA[3][3], contemporary methodologies for the characterization of generic biological entities remain conspicuously constrained, with low throughput[4][4], compromised lysis efficiency[5][5], and highly fragmented genomes[6][6]. Herein, we present the Generic Single Entity Sequencing platform (GSE-Seq), which boasts ample versatility, high throughput, and high coverage, and is enabled by an innovative workflow, addressing the critical challenges in single entities sequencing: (1) one-step manufacturing of massive barcodes, (2) degradable hydrogel-based in situ sample processing and whole genome amplification, (3) integrated in-drop library preparation, (4) compatible long-read sequencing. By GSE-Seq, we have achieved a significant milestone by enabling high-throughput, long-read single-entity profiling of dsDNA and ssDNA from single virus sequencing (SV-seq) and single bacteria sequencing (SB-seq) of the human gut and marine sediment for the first time. Notably, our analysis uncovered previously overlooked viral and bacterial dark matter and phage-host interactions. In summary, the presented conceptually new workflow offers a toolbox based on droplet microfluidics to tackle the persistent challenges in high-throughput profiling to generic applications, which hold immense promise for diverse biological entities, especially hard-to-lyse cells.
### Competing Interest Statement
Guoping Wang, Liuyang Zhao, Fuyang Qu, Yi-Ping Ho, and Jun Yu, are the inventors in the pending patent application related this work.
[1]: #ref-1
[2]: #ref-2
[3]: #ref-3
[4]: #ref-4
[5]: #ref-5
[6]: #ref-6
更多查看译文
关键词
high-throughput,single-entity
AI 理解论文
溯源树
样例
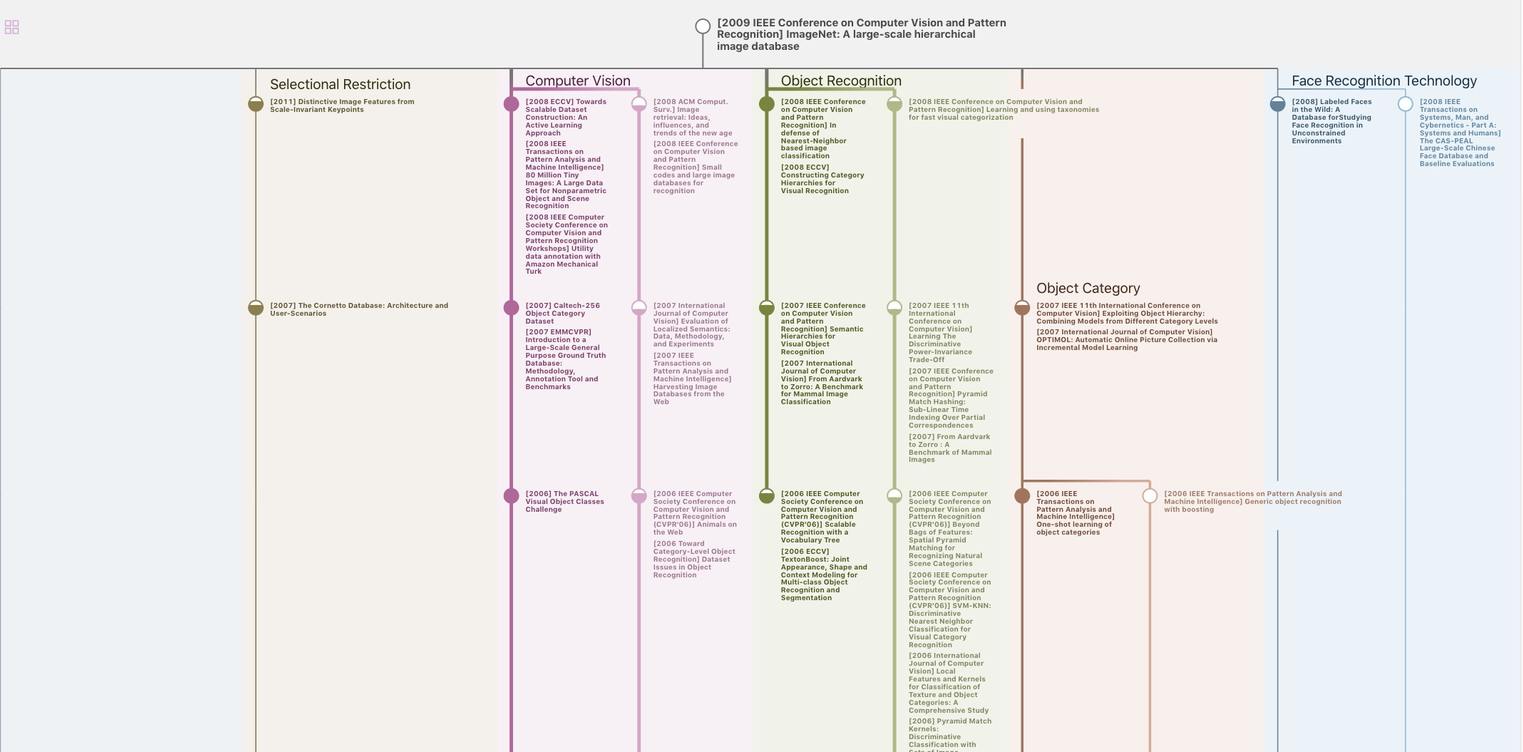
生成溯源树,研究论文发展脉络
Chat Paper
正在生成论文摘要