Predicting Protein-Ligand Binding Structure Using E(n) Equivariant Graph Neural Networks
bioRxiv (Cold Spring Harbor Laboratory)(2023)
摘要
Drug design is a costly and time-consuming process, often taking more than 12 years and costing up to billions of dollars. The COVID-19 pandemic has signified the urgent need for accelerated drug development. The initial stage of drug design involves the identification of ligands that exhibit a strong affinity for specific binding sites on protein targets (receptors), along with the determination of their precise binding conformation (3-dimensional (3D) structure). However, accurately determining the 3D conformation of a ligand binding with its target remains challenging due to the limited capability of exploring the huge chemical and protein structure space. To address this challenge, we propose a new E(n) Equivariant Graph Neural Network (EGNN) method for predicting the 3D binding structures of ligands and proteins. By treating proteins and ligands as graphs, the method extracts residue/atom-level node and edge features and utilizes physicochemical and geometrical properties of proteins and ligands to predict their binding structures. The results demonstrate the promising potential of EGNN for predicting ligand-protein binding poses.
### Competing Interest Statement
The authors have declared no competing interest.
更多查看译文
关键词
equivariant graph neural networks,neural networks,protein-ligand
AI 理解论文
溯源树
样例
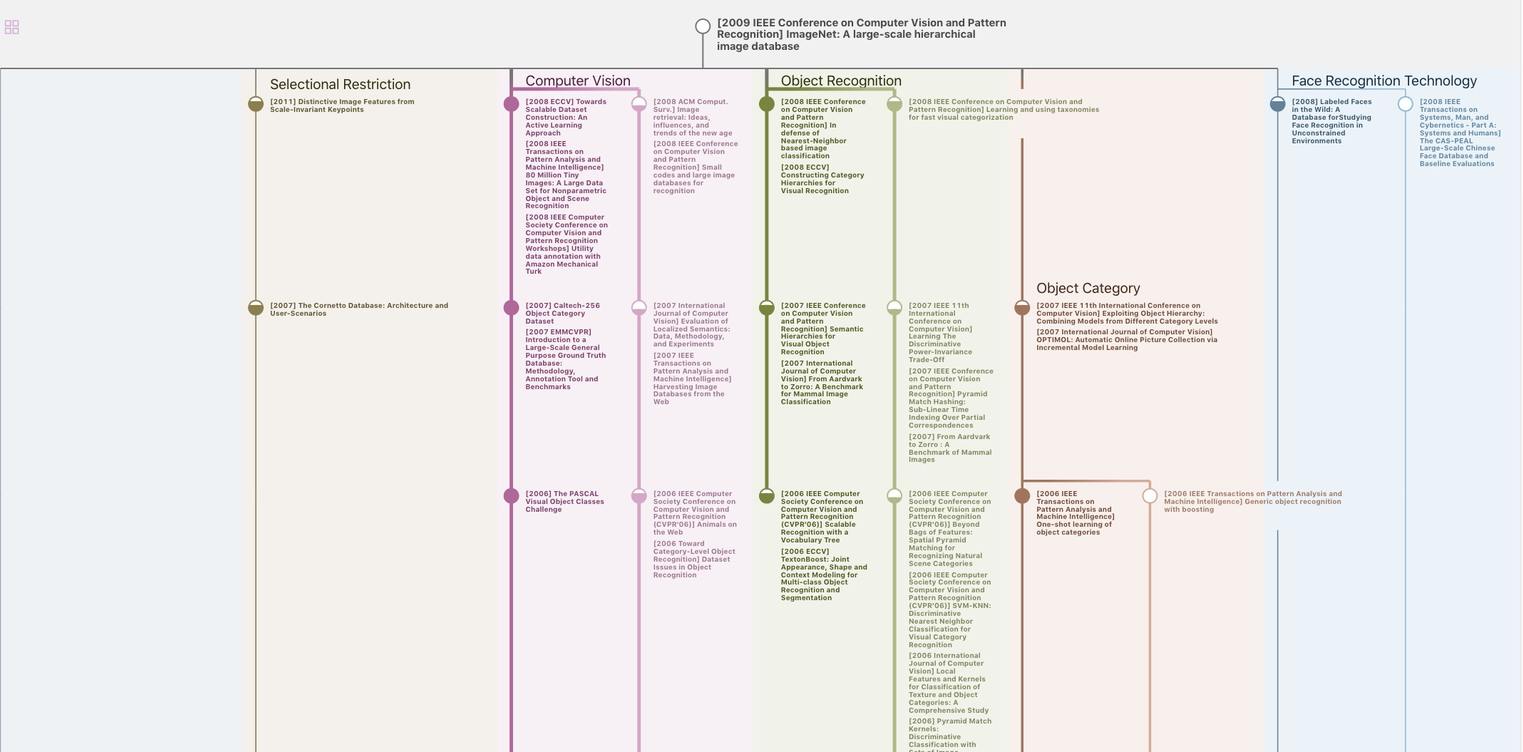
生成溯源树,研究论文发展脉络
Chat Paper
正在生成论文摘要