Machine learning illuminates how diet influences the evolution of yeast galactose metabolism
bioRxiv (Cold Spring Harbor Laboratory)(2023)
摘要
How genomic differences contribute to phenotypic differences across species is a major question in biology. The recently characterized genomes, isolation environments, and qualitative patterns of growth on 122 sources and conditions of 1,154 strains from 1,049 fungal species (nearly all known) in the subphylum Saccharomycotina provide a powerful, yet complex, dataset for addressing this question. In recent years, machine learning has been successfully used in diverse analyses of biological big data. Using a random forest classification algorithm trained on these genomic, metabolic, and/or environmental data, we predicted growth on several carbon sources and conditions with high accuracy from presence/absence patterns of genes and of growth in other conditions. Known structural genes involved in assimilation of these sources were important features contributing to prediction accuracy, whereas isolation environmental data were poor predictors. By further examining growth on galactose, we found that it can be predicted with high accuracy from either genomic (92.6%) or growth data in 120 other conditions (83.3%) but not from isolation environment data (65.7%). When we combined genomic and growth data, we noted that prediction accuracy was even higher (93.4%) and that, after the GAL actose utilization genes, the most important feature for predicting growth on galactose was growth on galactitol. These data raised the hypothesis that several species in two orders, Serinales and Pichiales (containing Candida auris and the genus Ogataea , respectively), have an alternative galactose utilization pathway because they lack the GAL genes. Growth and biochemical assays of several of these species confirmed that they utilize galactose through an oxidoreductive D-galactose pathway, rather than the canonical GAL pathway. We conclude that machine learning is a powerful tool for investigating the evolution of the yeast genotype-phenotype map and that it can help uncover novel biology, even in well-studied traits.
### Competing Interest Statement
A. R. is a scientific consultant for LifeMine Therapeutics, Inc. The authors declare no other competing interests.
更多查看译文
关键词
yeast,metabolism,machine learning,diet,evolution
AI 理解论文
溯源树
样例
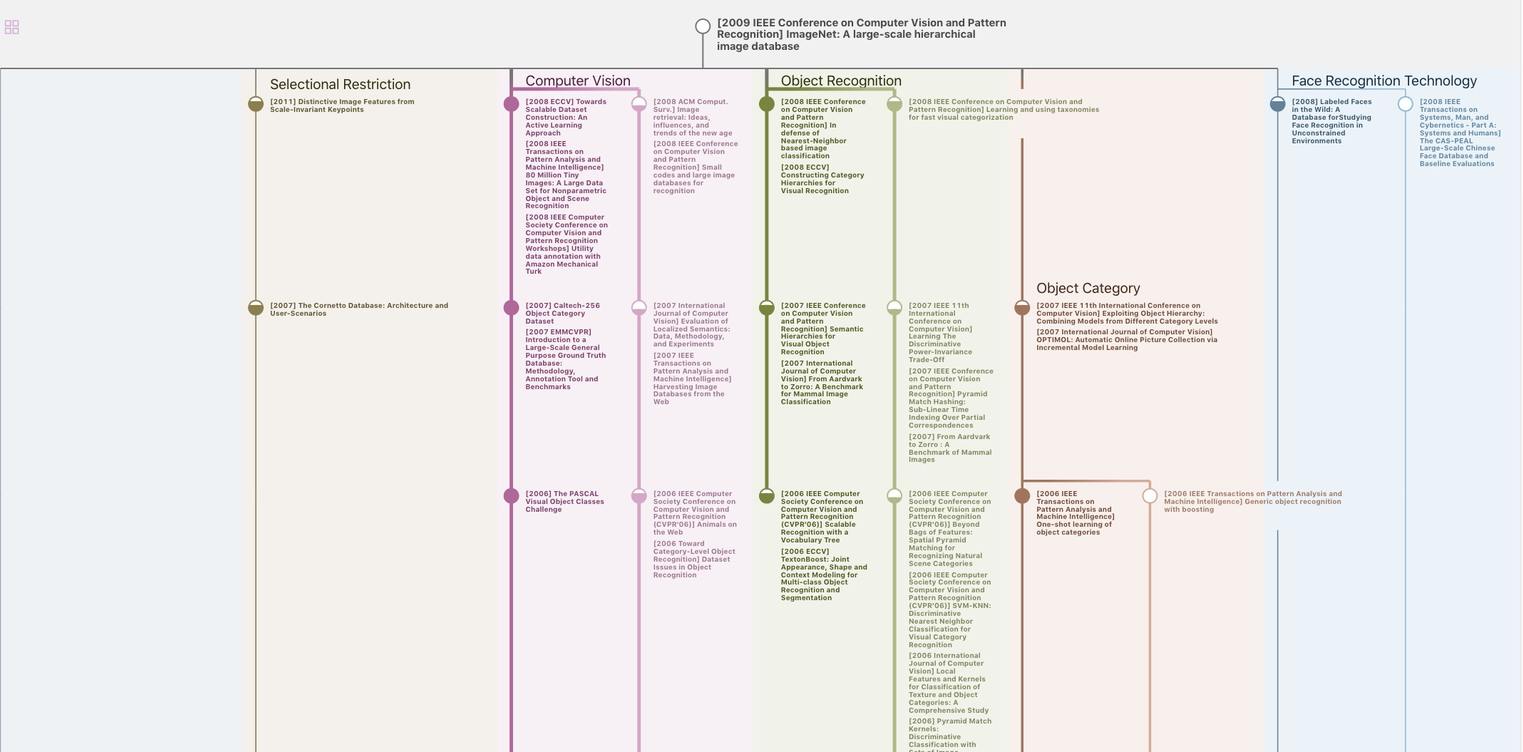
生成溯源树,研究论文发展脉络
Chat Paper
正在生成论文摘要