Learning a deep language model for microbiomes: the power of large scale unlabeled microbiome data
bioRxiv (Cold Spring Harbor Laboratory)(2023)
摘要
We use open source human gut microbiome data to learn a microbial “language” model by adapting techniques from Natural Language Processing (NLP). Our microbial “language” model is trained in a self-supervised fashion (i.e., without additional external labels) to capture the interactions among different microbial species and the common compositional patterns in microbial communities. The learned model produces contextualized taxa representations that allow a single bacteria species to be represented differently according to the specific microbial environment it appears in. The model further provides a sample representation by collectively interpreting different bacteria species in the sample and their interactions as a whole. We show that, compared to baseline representations, our sample representation consistently leads to improved performance for multiple prediction tasks including predicting Irritable Bowel Disease (IBD) and diet patterns. Coupled with a simple ensemble strategy, it produces a highly robust IBD prediction model that generalizes well to microbiome data independently collected from different populations with substantial distribution shift. We visualize the contextualized taxa representations and find that they exhibit meaningful phylum-level structure, despite never exposing the model to such a signal. Finally, we apply an interpretation method to highlight bacterial species that are particularly influential in driving our model’s predictions for IBD.
### Competing Interest Statement
The authors have declared no competing interest.
更多查看译文
关键词
microbiomes,deep language model,learning
AI 理解论文
溯源树
样例
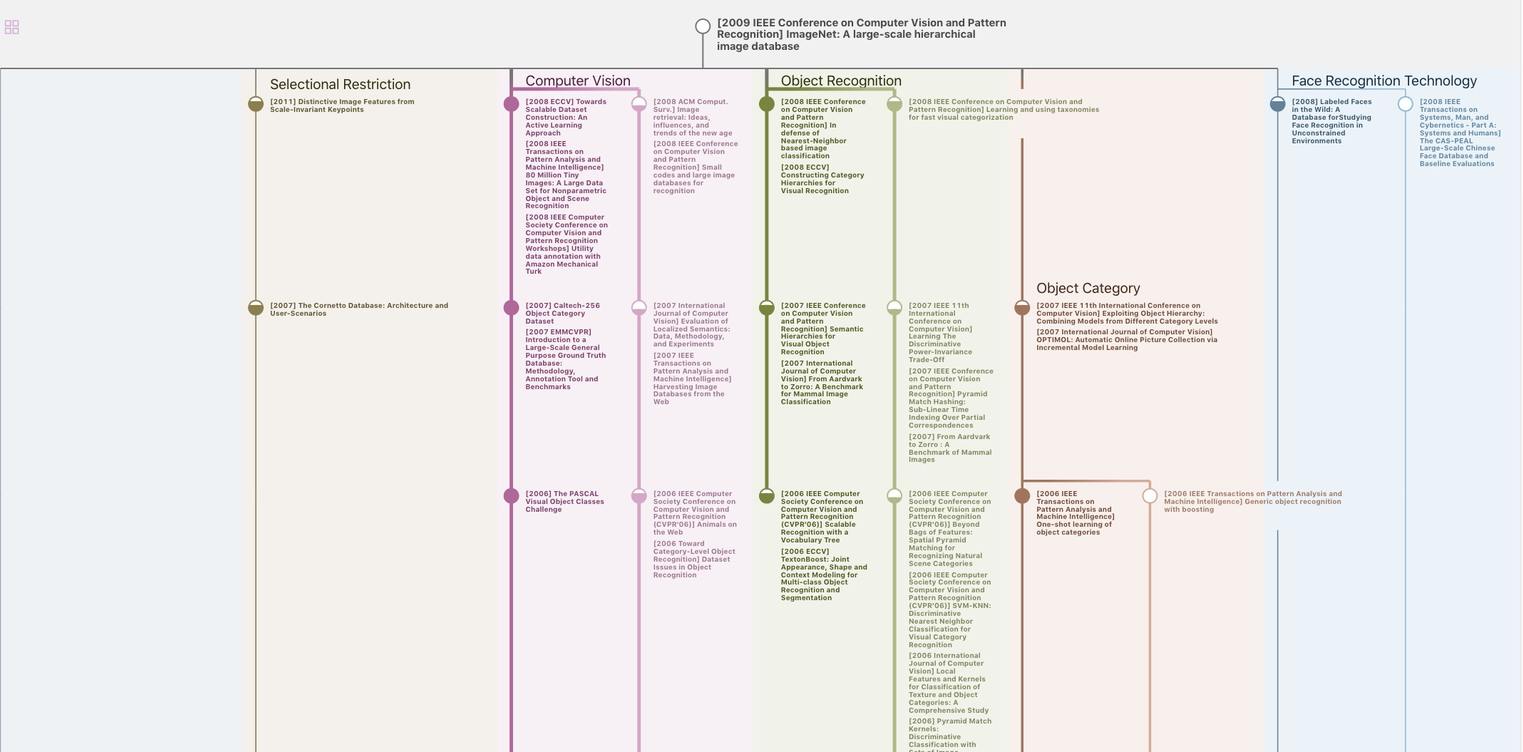
生成溯源树,研究论文发展脉络
Chat Paper
正在生成论文摘要