Applying t-Distributed Stochastic Neighbor Embedding for Improving Fingerprinting-Based Localization System
IEEE Sensors Letters(2023)
摘要
Large-scale location estimation is crucial for many artificial intelligence Internet of Things (IoT) applications in the era of smart cities. This letter proposes a deep learning-based outdoor positioning scheme for large-scale wireless settings using fingerprinting techniques. We first developed a feature extraction technique using t-distributed stochastic neighbor embedding (t-SNE) to extract dominant and distinguishable features while eliminating noises from the radio fingerprints. Afterward, we developed a deep learning-based coarse-fine localization framework to improve positioning performance significantly. Based on our numerical analysis, the proposed scheme reduces computation time by 64.41%, and the average positioning error is 38 cm. Therefore, the proposed approach significantly improved positioning accuracy and reduced computation time.
更多查看译文
关键词
Feature extraction technique,fingerprinting method,location estimation,long short-term memory (LSTM),signal fingerprints,support vector machine (SVM)
AI 理解论文
溯源树
样例
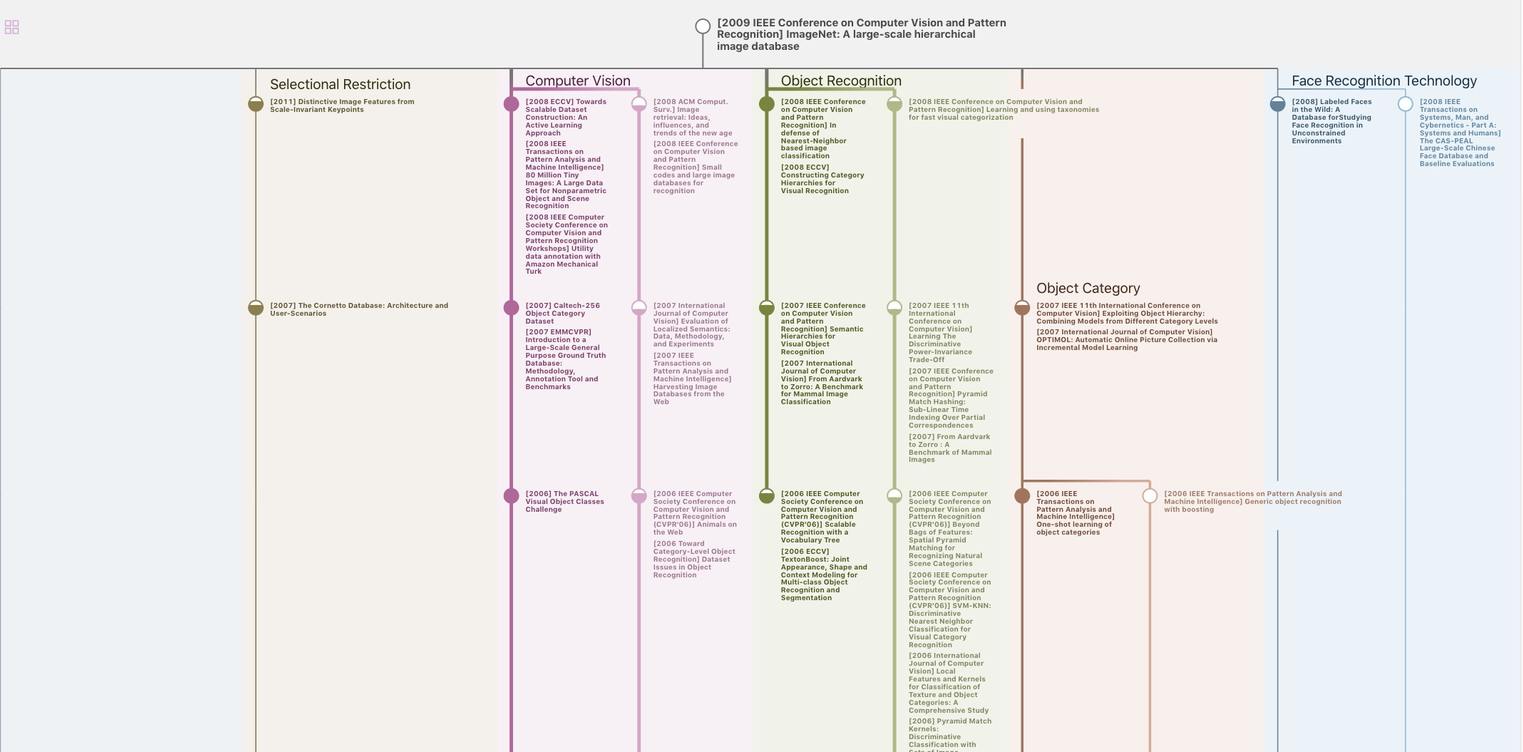
生成溯源树,研究论文发展脉络
Chat Paper
正在生成论文摘要