Robust time series recovery and classification using test-time noise simulator networks.
Proceedings of the ... IEEE International Conference on Acoustics, Speech, and Signal Processing. ICASSP (Conference)(2023)
摘要
Time-series are commonly susceptible to various types of corruption due to sensor-level changes and defects which can result in missing samples, sensor and quantization noise, unknown calibration, unknown phase shifts etc. These corruptions cannot be easily corrected as the noise model may be unknown at the time of deployment. This also results in the inability to employ pre-trained classifiers, trained on (clean) source data. In this paper, we present a general framework and models for time-series that can make use of (unlabeled) test samples to estimate the noise model-entirely at test time. To this end, we use a coupled decoder model and an additional neural network which acts as a learned noise model simulator. We show that the framework is able to "clean" the data so as to match the source training data statistics and the cleaned data can be directly used with a pre-trained classifier for robust predictions. We perform empirical studies on diverse application domains with different types of sensors, clearly demonstrating the effectiveness and generality of this method.
更多查看译文
关键词
Signal recovery,DNN,time series
AI 理解论文
溯源树
样例
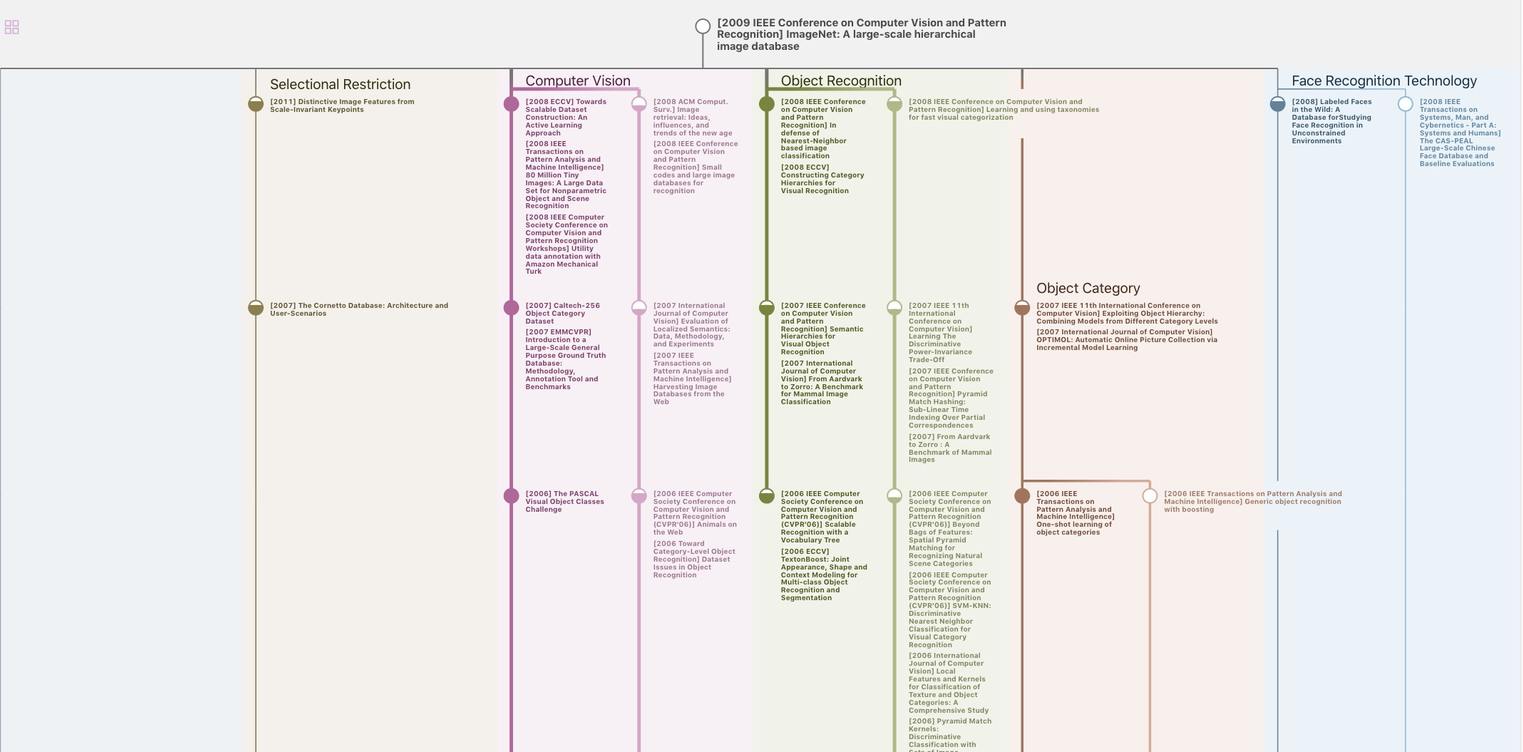
生成溯源树,研究论文发展脉络
Chat Paper
正在生成论文摘要