Enhanced PET imaging using progressive conditional deep image prior.
Physics in medicine and biology(2023)
Abstract
Unsupervised learning-based methods have been proven to be an effective way to improve the image quality of positron emission tomography (PET) images when a large dataset is not available. However, when the gap between the input image and the target PET image is large, direct unsupervised learning can be challenging and easily lead to reduced lesion detectability. We aim to develop a new unsupervised learning method to improve lesion detectability in patient studies.
Approach: We applied the deep progressive learning strategy to bridge the gap between the input image and the target image. The one-step unsupervised learning is decomposed into two unsupervised learning steps. The input image of the first network is an anatomical image and the input image of the second network is a PET image with a low noise level. The output of the first network is also used as the prior image to generate the target image of the second network by iterative reconstruction method.
Results: The performance of the proposed method was evaluated through the phantom and patient studies and compared with non-deep learning, supervised learning and unsupervised learning methods. The results showed that the proposed method was superior to non-deep learning and unsupervised methods, and is comparable to the supervised method.
Significance: A progressive unsupervised learning method was proposed, which can improve image noise performance and lesion detectability.
.
MoreTranslated text
Key words
PET image reconstruction, neural network, deep image prior, deep progressive learning
AI Read Science
Must-Reading Tree
Example
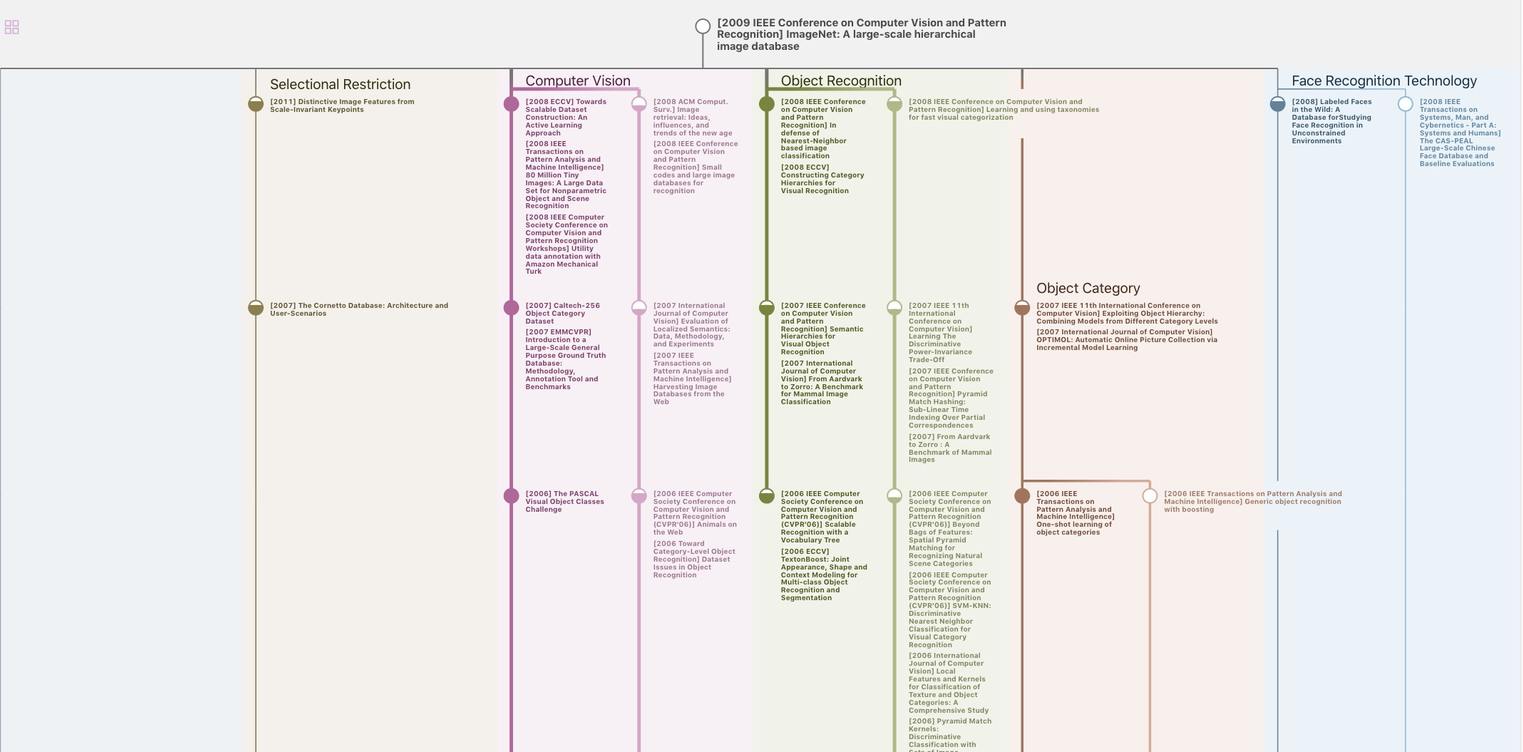
Generate MRT to find the research sequence of this paper
Chat Paper
Summary is being generated by the instructions you defined