Generalizing the Min-Max Regret Criterion using Ordered Weighted Averaging
arXiv (Cornell University)(2023)
摘要
In decision making under uncertainty, several criteria have been studied to aggregate the performance of a solution over multiple possible scenarios, including the ordered weighted averaging (OWA) criterion and min-max regret. This paper introduces a novel generalization of min-max regret, leveraging the modeling power of OWA to enable a more nuanced expression of preferences in handling regret values. This new OWA regret approach is studied both theoretically and numerically. We derive several properties, including polynomially solvable and hard cases, and introduce an approximation algorithm. Through computational experiments using artificial and real-world data, we demonstrate the advantages of our OWAR method over the conventional min-max regret approach, alongside the effectiveness of the proposed clustering heuristics.
更多查看译文
关键词
ordered weighted
AI 理解论文
溯源树
样例
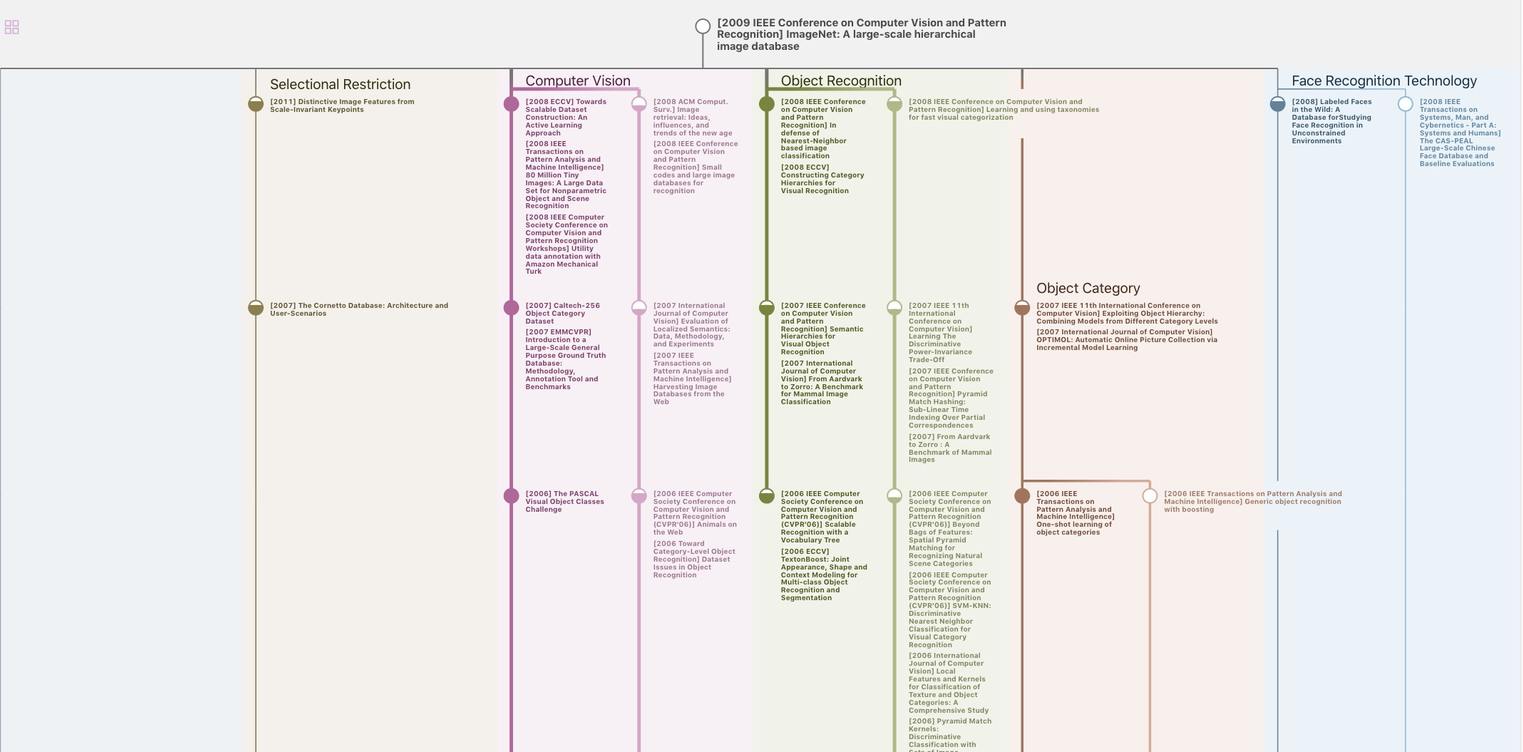
生成溯源树,研究论文发展脉络
Chat Paper
正在生成论文摘要