QMLMaterial RA Quantum Machine Learning Software for Material Design and Discovery
Journal of chemical theory and computation(2023)
摘要
Structuralelucidation of chemical compounds is challenging experimentally,and theoretical chemistry methods have added important insight intomolecules, nanoparticles, alloys, and materials geometries and properties.However, finding the optimum structures is a bottleneck due to thehuge search space, and global search algorithms have been used successfullyfor this purpose. In this work, we present the quantum machine learningsoftware/agent for materials design and discovery (QMLMaterial), intendedfor automatic structural determination in silico forseveral chemical systems: atomic clusters, atomic clusters and thespin multiplicity together, doping in clusters or solids, vacanciesin clusters or solids, adsorption of molecules or adsorbents on surfaces,and finally atomic clusters on solid surfaces/materials or encapsulatedin porous materials. QMLMaterial is an artificial intelligence (AI)software based on the active learning method, which uses machine learningregression algorithms and their uncertainties for decision makingon the next unexplored structures to be computed, increasing the probabilityof finding the global minimum with few calculations as more data isobtained. The software has different acquisition functions for decisionmaking (e.g., expected improvement and lower confidence bound). Also,the Gaussian process is available in the AI framework for regression,where the uncertainty is obtained analytically from Bayesian statistics.For the artificial neural network and support vector regressor algorithms,the uncertainty can be obtained by K-fold cross-validation or nonparametricbootstrap resampling methods. The software is interfaced with severalquantum chemistry codes and atomic descriptors, such as the many-bodytensor representation. QMLMaterial's capabilities are highlightedin the current work by its applications in the following systems:Na-20, Mo6C3 (where the spin multiplicitywas considered), H2O@CeNi3O5, Mg-8@graphene, Na3Mg3@CNT (carbon nanotube).
更多查看译文
关键词
quantum machine learning software,qmlmaterial─a design,machine learning,discovery
AI 理解论文
溯源树
样例
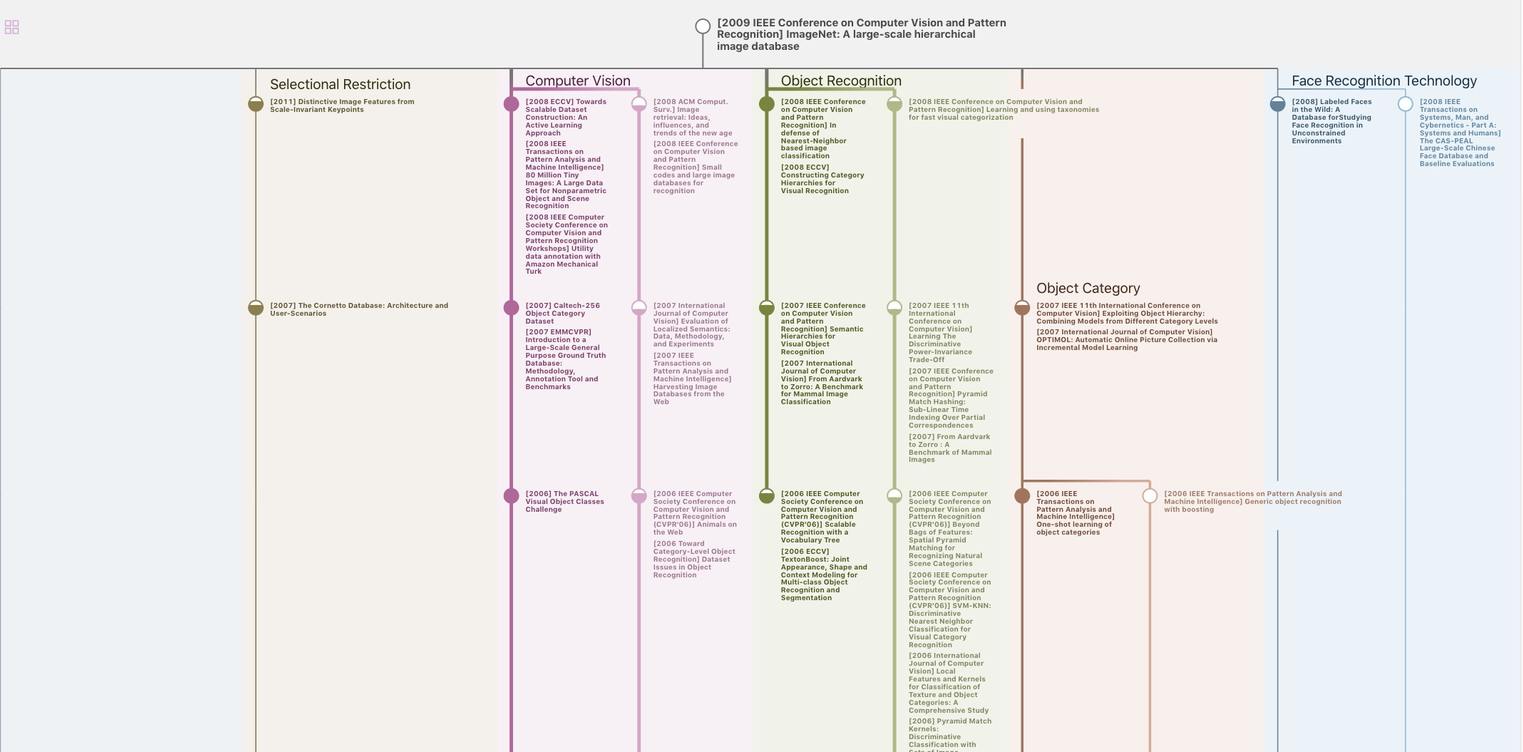
生成溯源树,研究论文发展脉络
Chat Paper
正在生成论文摘要