Splicing defects in rare diseases: transcriptomics and machine learning strategies towards genetic diagnosis.
Briefings in bioinformatics(2023)
Abstract
Genomic variants affecting pre-messenger RNA splicing and its regulation are known to underlie many rare genetic diseases. However, common workflows for genetic diagnosis and clinical variant interpretation frequently overlook splice-altering variants. To better serve patient populations and advance biomedical knowledge, it has become increasingly important to develop and refine approaches for detecting and interpreting pathogenic splicing variants. In this review, we will summarize a few recent developments and challenges in using RNA sequencing technologies for rare disease investigation. Moreover, we will discuss how recent computational splicing prediction tools have emerged as complementary approaches for revealing disease-causing variants underlying splicing defects. We speculate that continuous improvements to sequencing technologies and predictive modeling will not only expand our understanding of splicing regulation but also bring us closer to filling the diagnostic gap for rare disease patients.
MoreTranslated text
Key words
splicing, rare disease, variant interpretation, diagnostics, RNA sequencing, machine learning
AI Read Science
Must-Reading Tree
Example
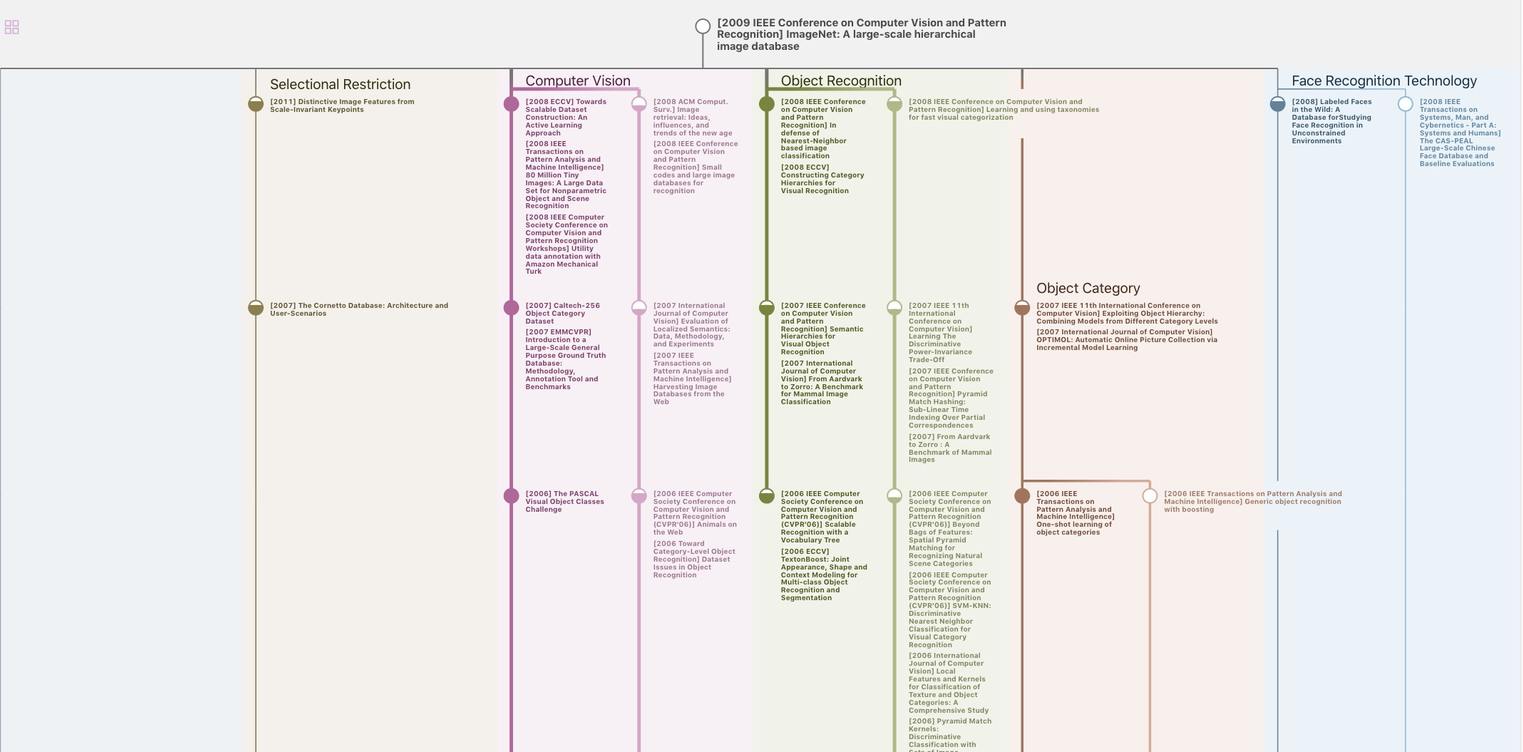
Generate MRT to find the research sequence of this paper
Chat Paper
Summary is being generated by the instructions you defined