Domain-Adaptive Device Fingerprints for Network Access Authentication Through Multifractal Dimension Representation
CoRR(2023)
摘要
RF data-driven device fingerprinting through the use of deep learning has recently surfaced as a potential solution for automated network access authentication. Traditional approaches are commonly susceptible to the domain adaptation problem where a model trained on data from one domain performs badly when tested on data from a different domain. Some examples of a domain change include varying the device location or environment and varying the time or day of data collection. In this work, we propose using multifractal analysis and the variance fractal dimension trajectory (VFDT) as a data representation input to the deep neural network to extract device fingerprints that are domain generalizable. We analyze the effectiveness of the proposed VFDT representation in detecting device-specific signatures from hardware-impaired IQ signals, and evaluate its robustness in real-world settings, using an experimental testbed of 30 WiFi-enabled Pycom devices under different locations and at different scales. Our results show that the VFDT representation improves the scalability, robustness and generalizability of the deep learning models significantly compared to when using raw IQ data.
更多查看译文
关键词
network access authentication,domain-adaptive
AI 理解论文
溯源树
样例
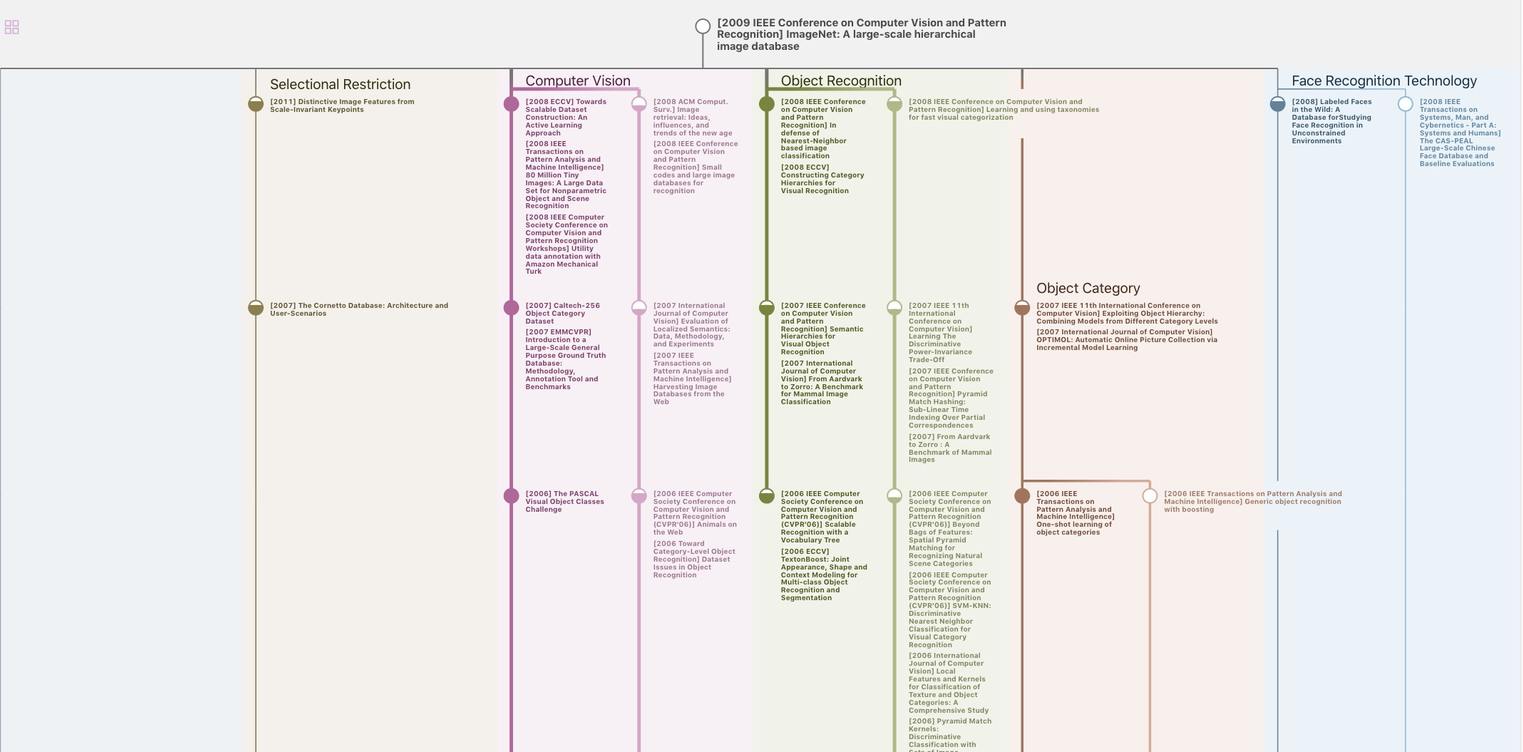
生成溯源树,研究论文发展脉络
Chat Paper
正在生成论文摘要