Emotion Embeddings $\unicode{x2014}$ Learning Stable and Homogeneous Abstractions from Heterogeneous Affective Datasets
arXiv (Cornell University)(2023)
摘要
Human emotion is expressed in many communication modalities and media formats and so their computational study is equally diversified into natural language processing, audio signal analysis, computer vision, etc. Similarly, the large variety of representation formats used in previous research to describe emotions (polarity scales, basic emotion categories, dimensional approaches, appraisal theory, etc.) have led to an ever proliferating diversity of datasets, predictive models, and software tools for emotion analysis. Because of these two distinct types of heterogeneity, at the expressional and representational level, there is a dire need to unify previous work on increasingly diverging data and label types. This article presents such a unifying computational model. We propose a training procedure that learns a shared latent representation for emotions, so-called emotion embeddings, independent of different natural languages, communication modalities, media or representation label formats, and even disparate model architectures. Experiments on a wide range of heterogeneous affective datasets indicate that this approach yields the desired interoperability for the sake of reusability, interpretability and flexibility, without penalizing prediction quality. Code and data are archived under https://doi.org/10.5281/zenodo.7405327 .
更多查看译文
关键词
heterogeneous affective datasets,emotion embeddings,abstractions
AI 理解论文
溯源树
样例
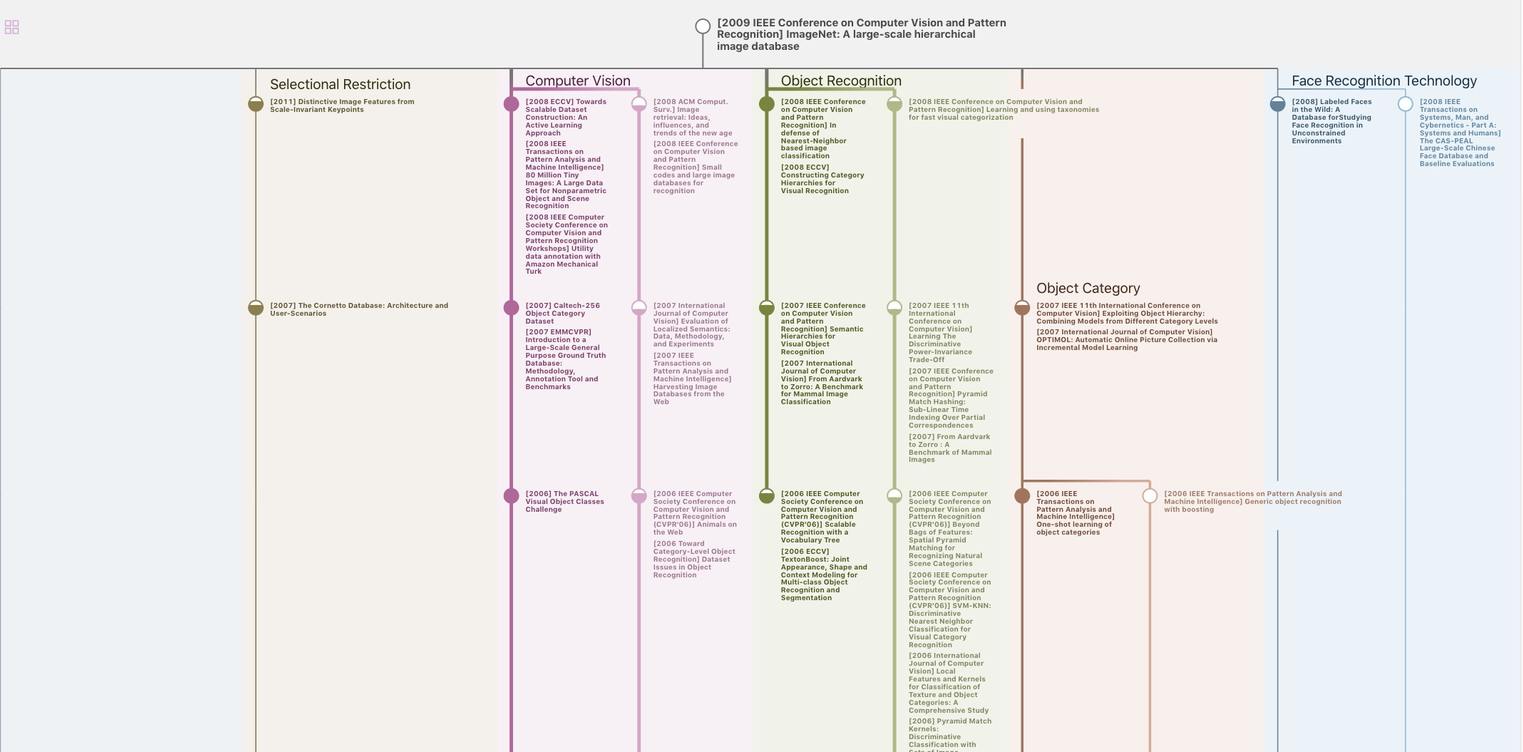
生成溯源树,研究论文发展脉络
Chat Paper
正在生成论文摘要