Self-prompting Large Vision Models for Few-Shot Medical Image Segmentation
DOMAIN ADAPTATION AND REPRESENTATION TRANSFER, DART 2023(2024)
摘要
Recent advancements in large foundation models have shown promising potential in the medical industry due to their flexible prompting capability. One such model, the Segment Anything Model (SAM), a prompt-driven segmentation model, has shown remarkable performance improvements, surpassing state-of-the-art approaches in medical image segmentation. However, existing methods primarily rely on tuning strategies that require extensive data or prior prompts tailored to the specific task, making it particularly challenging when only a limited number of data samples are available. In this paper, we propose a novel perspective on self-prompting in medical vision applications. Specifically, we harness the embedding space of SAM to prompt itself through a simple yet effective linear pixel-wise classifier. By preserving the encoding capabilities of the large model, the contextual information from its decoder, and leveraging its interactive promptability, we achieve competitive results on multiple datasets (i.e. improvement of more than 15% compared to fine-tuning the mask decoder using a few images). Our code is available at https://github.com/PeterYYZhang/few-shot-self-prompt-SAM
更多查看译文
关键词
Image Segmentation,Few-shot Learning,SAM
AI 理解论文
溯源树
样例
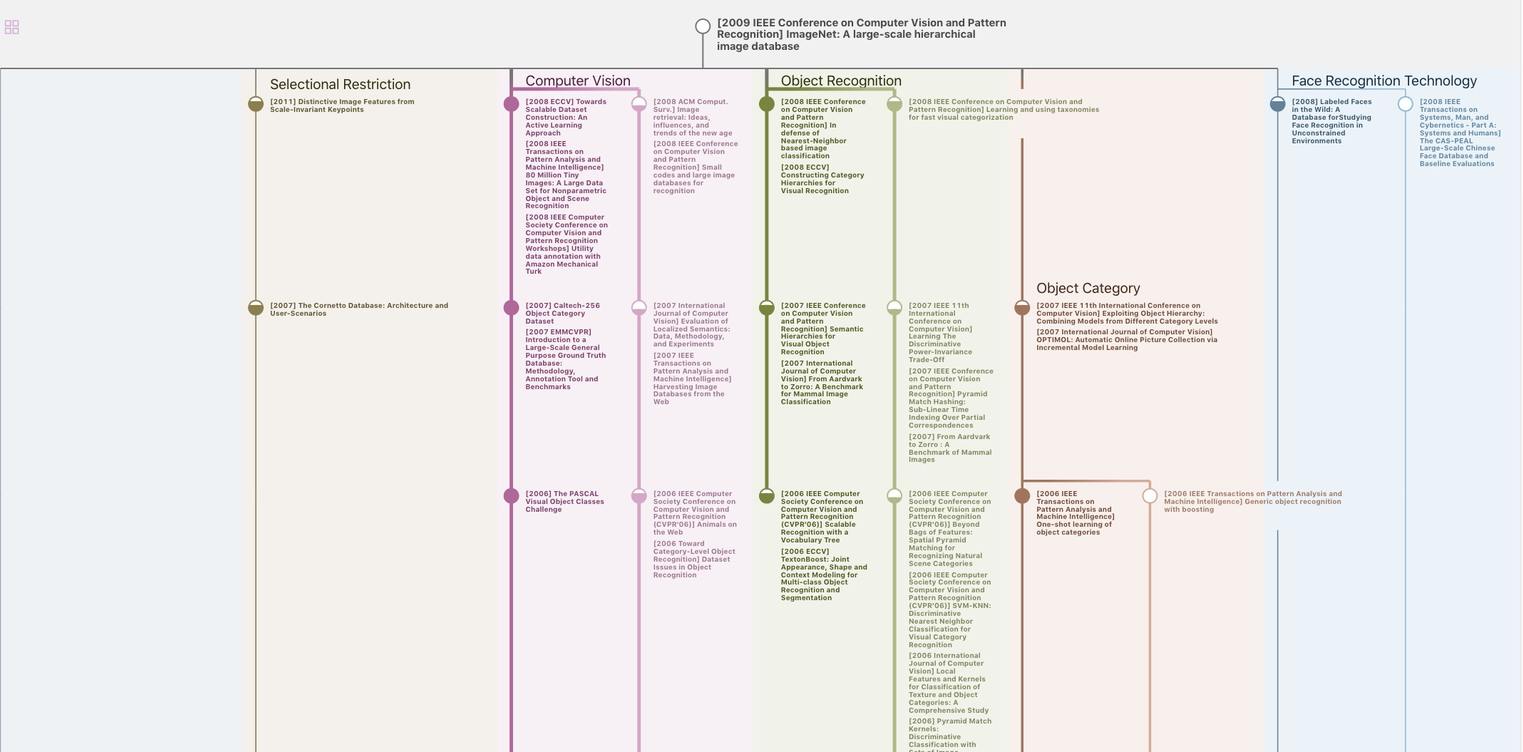
生成溯源树,研究论文发展脉络
Chat Paper
正在生成论文摘要