SST: A Simplified Swin Transformer-based Model for Taxi Destination Prediction based on Existing Trajectory
CoRR(2023)
摘要
Accurately predicting the destination of taxi trajectories can have various benefits for intelligent location-based services. One potential method to accomplish this prediction is by converting the taxi trajectory into a two-dimensional grid and using computer vision techniques. While the Swin Transformer is an innovative computer vision architecture with demonstrated success in vision downstream tasks, it is not commonly used to solve real-world trajectory problems. In this paper, we propose a simplified Swin Transformer (SST) structure that does not use the shifted window idea in the traditional Swin Transformer, as trajectory data is consecutive in nature. Our comprehensive experiments, based on real trajectory data, demonstrate that SST can achieve higher accuracy compared to state-of-the-art methods.
更多查看译文
关键词
Destination Prediction,Computer Vision,Local Services,Trajectory Data,Traditional Transformation,Convolutional Neural Network,Linear Method,Long Short-term Memory,Recurrent Neural Network,Grid Cells,Multilayer Perceptron,Prediction Task,Transformer Model,Graph Convolutional Network,Final Destination,Gated Recurrent Unit,Long Short-term Memory Model,Trajectory Length,Physics-based Models,Binary Method,Trajectory Prediction,Quadratic Method,Short-term Memory Neural Network,Vision Transformer,Multi-head Self-attention,Road Structure,Prediction Problem,Neural Network,Vehicle Trajectory,Long-range Dependencies
AI 理解论文
溯源树
样例
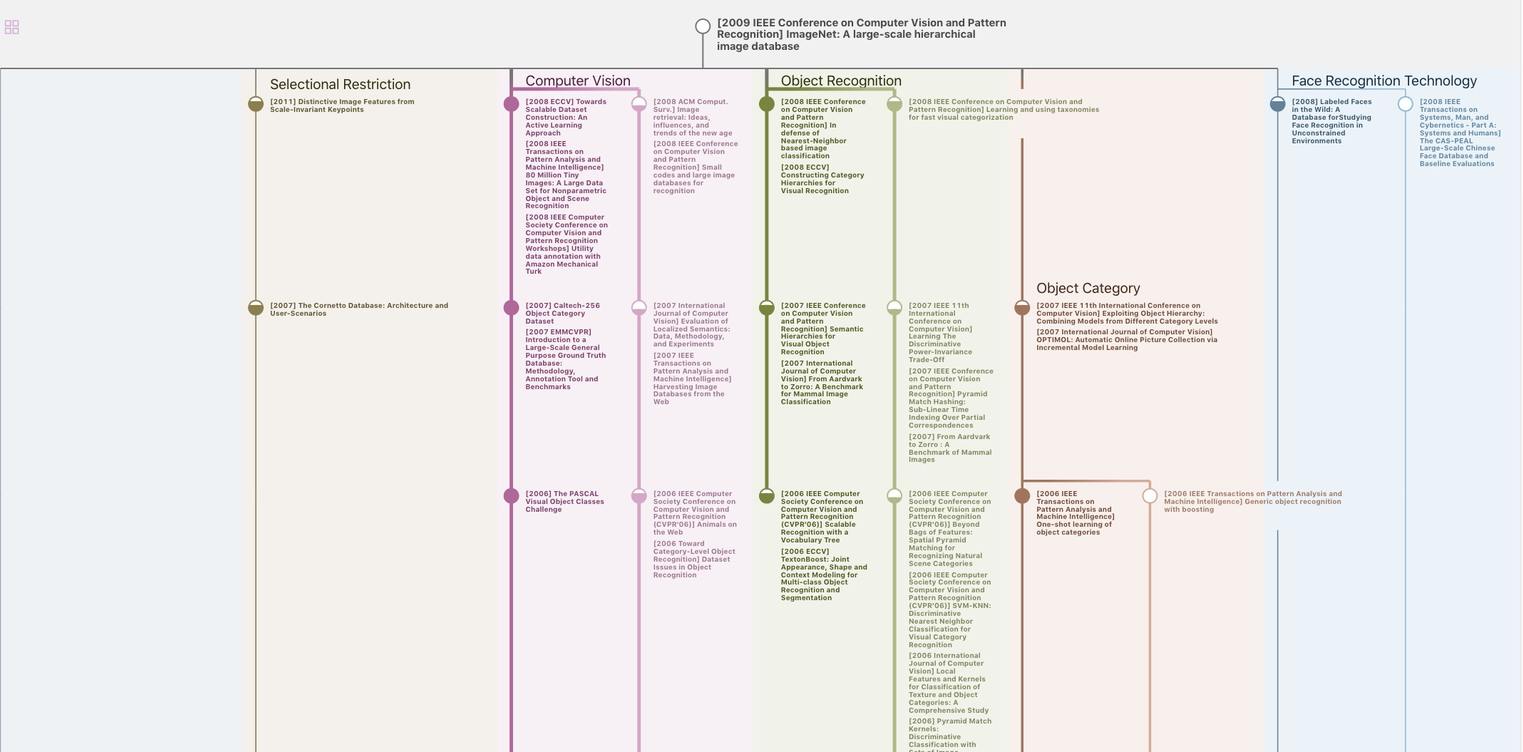
生成溯源树,研究论文发展脉络
Chat Paper
正在生成论文摘要