Improved Region Proposal Network for Enhanced Few-Shot Object Detection
CoRR(2023)
摘要
Despite significant success of deep learning in object detection tasks, the standard training of deep neural networks requires access to a substantial quantity of annotated images across all classes. Data annotation is an arduous and time-consuming endeavor, particularly when dealing with infrequent objects. Few-shot object detection (FSOD) methods have emerged as a solution to the limitations of classic object detection approaches based on deep learning. FSOD methods demonstrate remarkable performance by achieving robust object detection using a significantly smaller amount of training data. A challenge for FSOD is that instances from novel classes that do not belong to the fixed set of training classes appear in the background and the base model may pick them up as potential objects. These objects behave similarly to label noise because they are classified as one of the training dataset classes, leading to FSOD performance degradation. We develop a semi-supervised algorithm to detect and then utilize these unlabeled novel objects as positive samples during the FSOD training stage to improve FSOD performance. Specifically, we develop a hierarchical ternary classification region proposal network (HTRPN) to localize the potential unlabeled novel objects and assign them new objectness labels to distinguish these objects from the base training dataset classes. Our improved hierarchical sampling strategy for the region proposal network (RPN) also boosts the perception ability of the object detection model for large objects. We test our approach and COCO and PASCAL VOC baselines that are commonly used in FSOD literature. Our experimental results indicate that our method is effective and outperforms the existing state-of-the-art (SOTA) FSOD methods. Our implementation is provided as a supplement to support reproducibility of the results.
更多查看译文
关键词
detection,region,few-shot
AI 理解论文
溯源树
样例
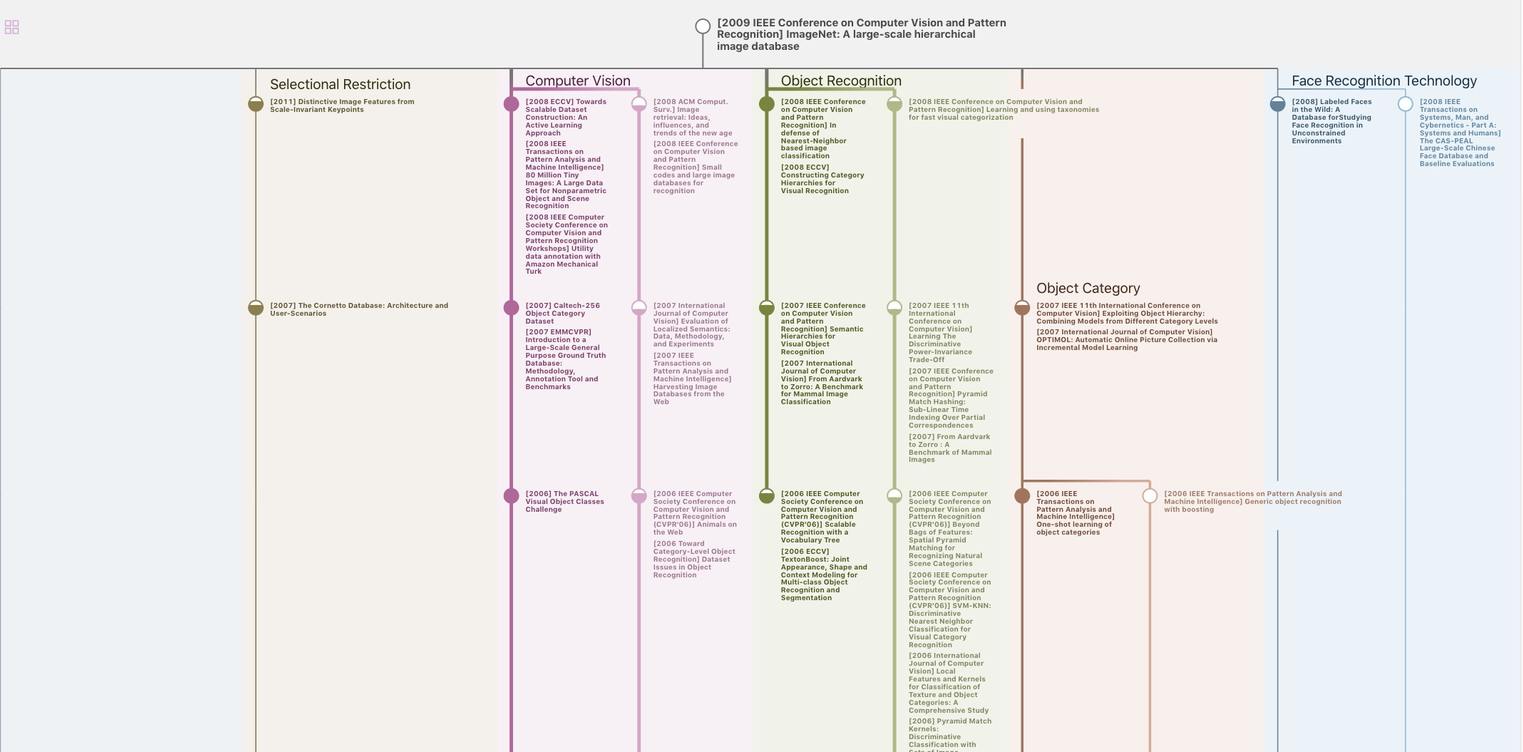
生成溯源树,研究论文发展脉络
Chat Paper
正在生成论文摘要