Spatial Omics Driven Crossmodal Pretraining Applied to Graph-based Deep Learning for Cancer Pathology Analysis.
bioRxiv : the preprint server for biology(2024)
摘要
Graph-based deep learning has shown great promise in cancer histopathology image analysis by contextualizing complex morphology and structure across whole slide images to make high quality downstream outcome predictions (ex: prognostication). These methods rely on informative representations (i.e., embeddings) of image patches comprising larger slides, which are used as node attributes in slide graphs. Spatial omics data, including spatial transcriptomics, is a novel paradigm offering a wealth of detailed information. Pairing this data with corresponding histological imaging localized at 50-micron resolution, may facilitate the development of algorithms which better appreciate the morphological and molecular underpinnings of carcinogenesis. Here, we explore the utility of leveraging spatial transcriptomics data with a contrastive crossmodal pretraining mechanism to generate deep learning models that can extract molecular and histological information for graph-based learning tasks. Performance on cancer staging, lymph node metastasis prediction, survival prediction, and tissue clustering analyses indicate that the proposed methods bring improvement to graph based deep learning models for histopathological slides compared to leveraging histological information from existing schemes, demonstrating the promise of mining spatial omics data to enhance deep learning for pathology workflows.
更多查看译文
关键词
deep learning,graph-based
AI 理解论文
溯源树
样例
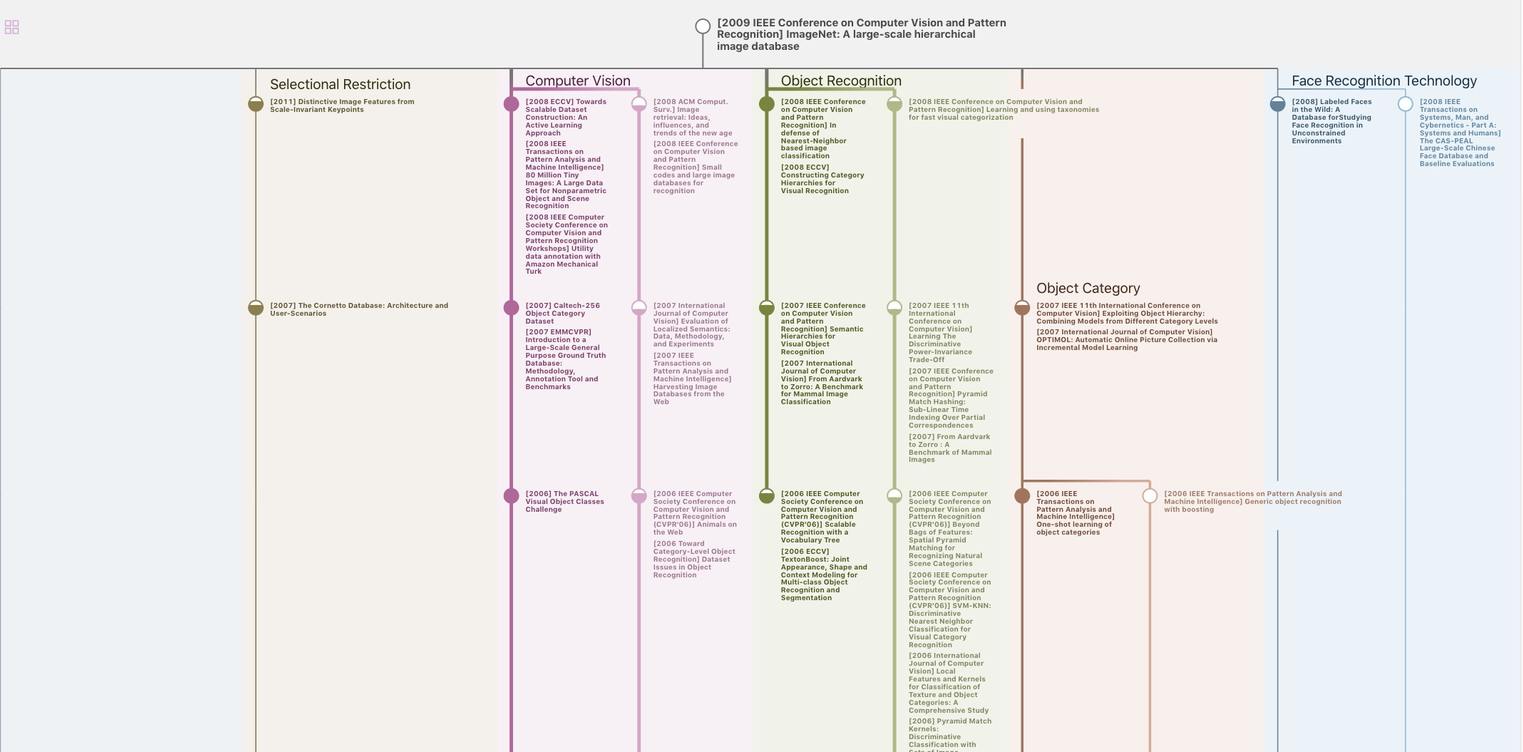
生成溯源树,研究论文发展脉络
Chat Paper
正在生成论文摘要