Machine learning: Next promising trend for microplastics study
JOURNAL OF ENVIRONMENTAL MANAGEMENT(2023)
摘要
Microplastics (MPs), as an emerging pollutant, pose a significant threat to humans and ecosystems. However, traditional MPs characterization methods are limited by sample requirements and characterization time. Machine Learning (ML) has emerged as a vital technology for analyzing MPs pollution due to its accuracy, broad application, and powerful feature extraction. Nevertheless, environmental scientists require threshold knowledge before using ML, restricting the ML application in MPs research. Furthermore, imbalanced development of ML in MPs research is a pressing concern. In order to achieve a wide ML application in MPs research, in this review, we comprehensively discussed the size and sources of MPs datasets in relevant literature to help environmental scientists deepen their understanding of the construction of MPs datasets. Commonly used ML algorithms are analyzed from the perspective of interpretability and the need for computer facilities. Additionally, methods for improving and evaluating ML model performance, such as dataset pre-processing, model optimization, and model assessment metrics, are discussed. According to datasets and characterization techniques, MPs identification using ML was divided into three categories in this work: spectral identification, image identification, and spectral imaging identification. Finally, other applications of ML in MPs studies, including toxicity analysis, pollutants adsorption, and microbial colonization, are comprehensively discussed, which reveals the great application potential of ML. Based on the discussion above, this review suggests an algorithm selection strategy to assist researchers in selecting the most suitable ML algorithm in different situations, improving efficiency and decreasing the costs of trial and error. We believe that this work sheds light on the application of ML in MPs study.
更多查看译文
关键词
Microplastics,Machine learning,Algorithm selection strategies,Model performance
AI 理解论文
溯源树
样例
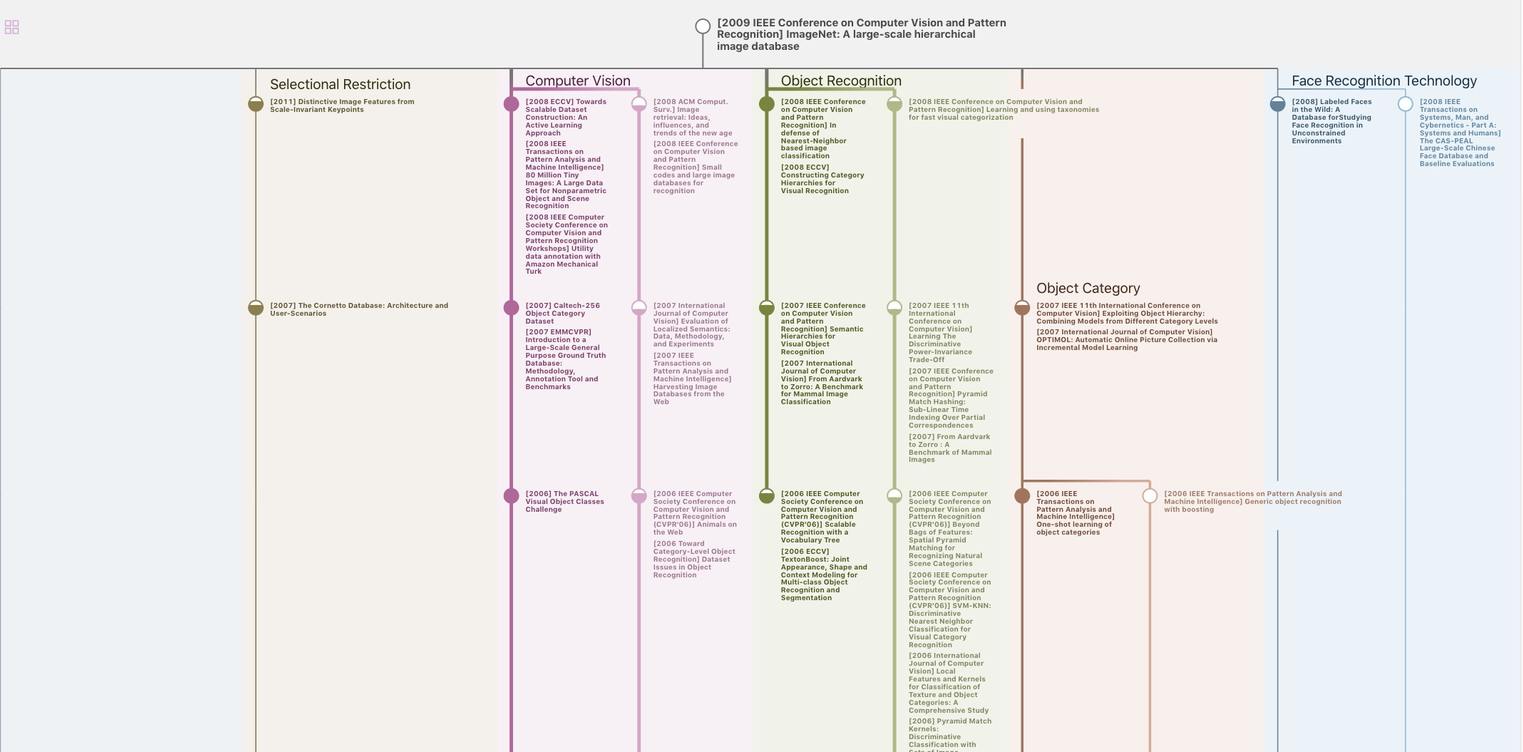
生成溯源树,研究论文发展脉络
Chat Paper
正在生成论文摘要