DiffSED: Sound Event Detection with Denoising Diffusion
AAAI 2024(2024)
摘要
Sound Event Detection (SED) aims to predict the temporal boundaries of all the events of interest and their class labels, given an unconstrained audio sample. Taking either the split-and-classify (i.e., frame-level) strategy or the more principled event-level modeling approach, all existing methods consider the SED problem from the discriminative learning perspective. In this work, we reformulate the SED problem by taking a generative learning perspective. Specifically, we aim to generate sound temporal boundaries from noisy proposals in a denoising diffusion process, conditioned on a target audio sample. During training, our model learns to reverse the noising process by converting noisy latent queries to the ground-truth versions in the elegant Transformer decoder framework. Doing so enables the model generate accurate event boundaries from even noisy queries during inference. Extensive experiments on the Urban-SED and EPIC-Sounds datasets demonstrate that our model significantly outperforms existing alternatives, with 40+% faster convergence in training. Code: https://github.com/Surrey-UPLab/DiffSED
更多查看译文
关键词
CV: Scene Analysis & Understanding,ML: Deep Generative Models & Autoencoders,ML: Multimodal Learning
AI 理解论文
溯源树
样例
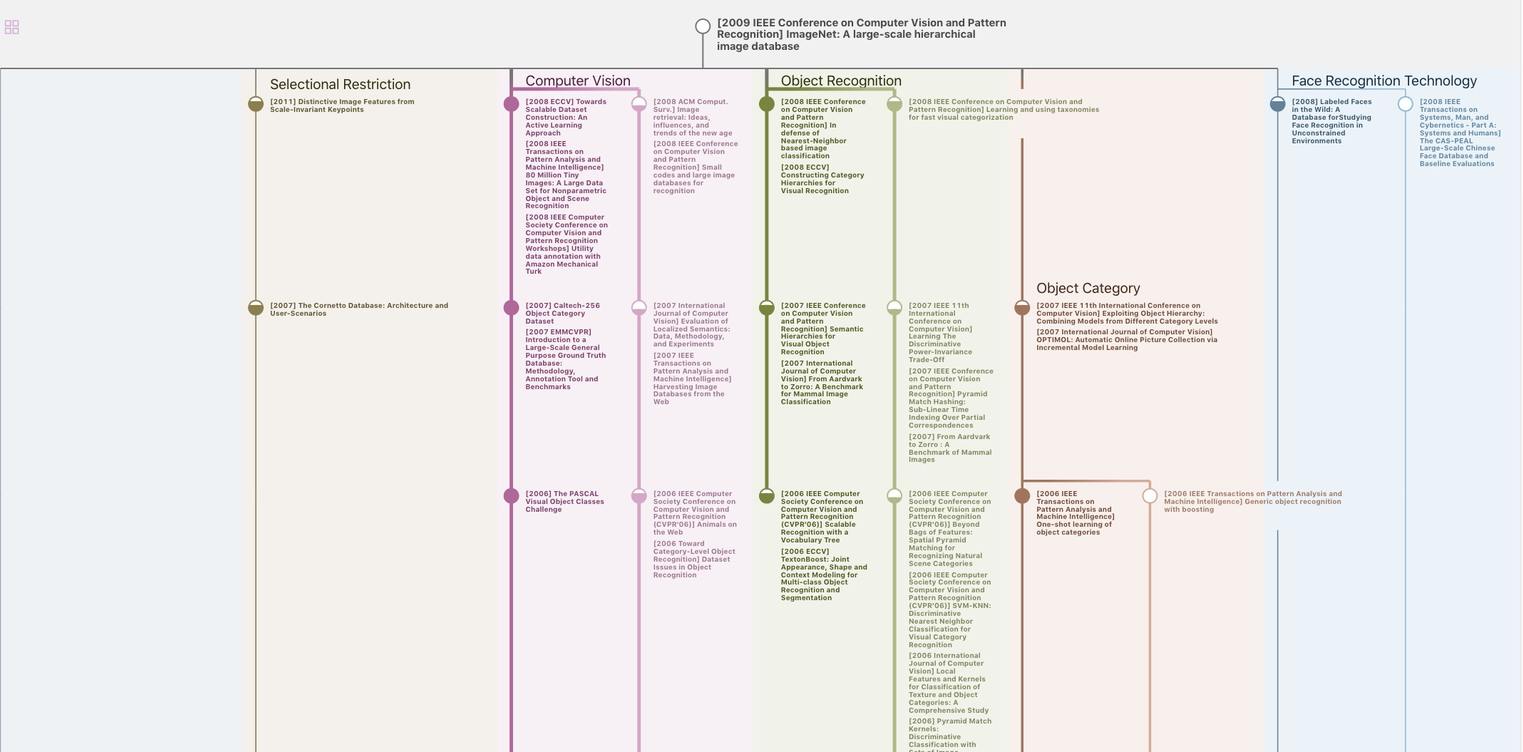
生成溯源树,研究论文发展脉络
Chat Paper
正在生成论文摘要