Data-Efficient Energy-Aware Participant Selection for UAV-Enabled Federated Learning
CoRR(2023)
摘要
Unmanned aerial vehicle (UAV)-enabled edge federated learning (FL) has sparked a rise in research interest as a result of the massive and heterogeneous data collected by UAVs, as well as the privacy concerns related to UAV data transmissions to edge servers. However, due to the redundancy of UAV collected data, e.g., imaging data, and non-rigorous FL participant selection, the convergence time of the FL learning process and bias of the FL model may increase. Consequently, we investigate in this paper the problem of selecting UAV participants for edge FL, aiming to improve the FL model's accuracy, under UAV constraints of energy consumption, communication quality, and local datasets' heterogeneity. We propose a novel UAV participant selection scheme, called data-efficient energy-aware participant selection strategy (DEEPS), which consists of selecting the best FL participant in each sub-region based on the structural similarity index measure (SSIM) average score of its local dataset and its power consumption profile. Through experiments, we demonstrate that the proposed selection scheme is superior to the benchmark random selection method, in terms of model accuracy, training time, and UAV energy consumption.
更多查看译文
关键词
Unmanned aerial vehicle,UAV,federated learning,FL,edge computing
AI 理解论文
溯源树
样例
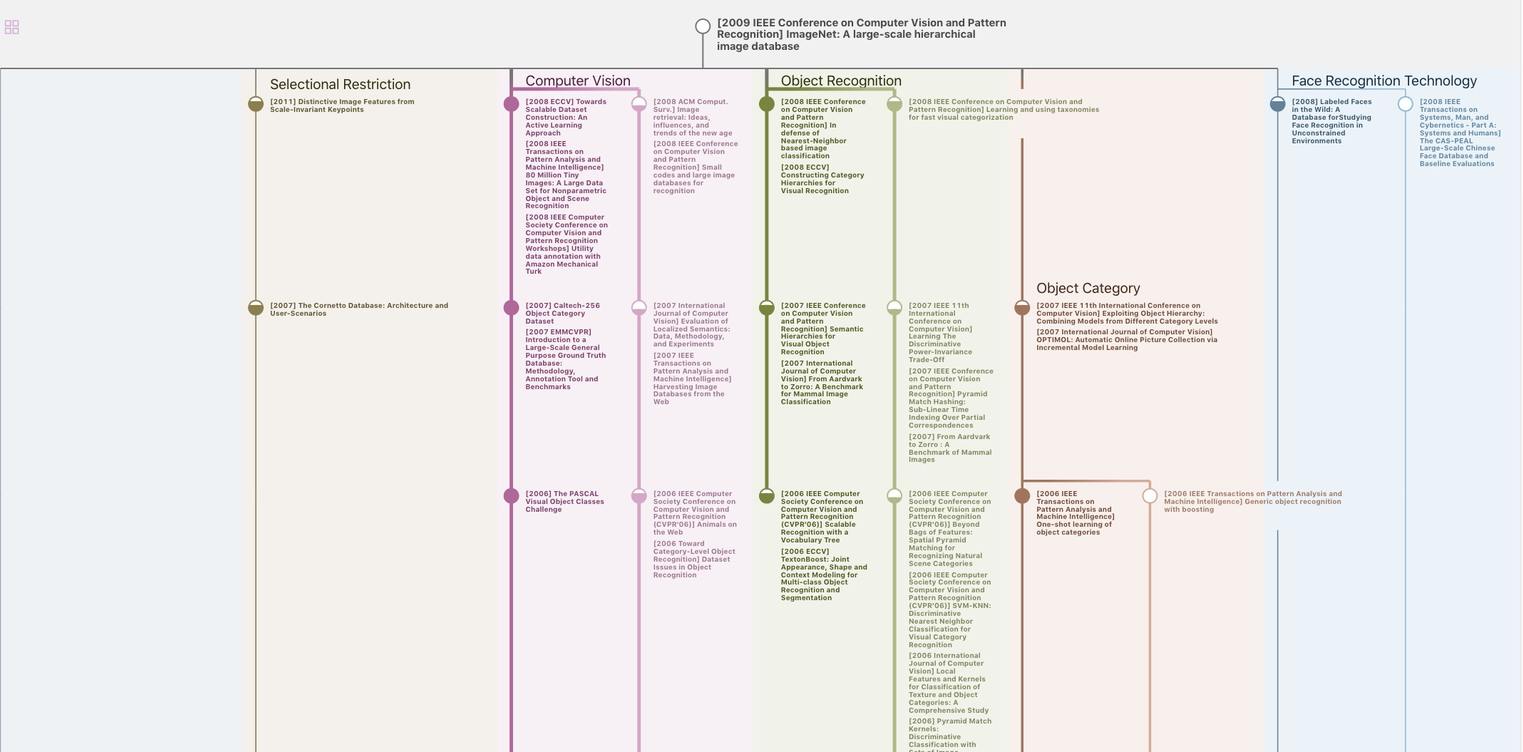
生成溯源树,研究论文发展脉络
Chat Paper
正在生成论文摘要