Machine Unlearning: Solutions and Challenges
IEEE TRANSACTIONS ON EMERGING TOPICS IN COMPUTATIONAL INTELLIGENCE(2024)
Abstract
Machine learning models may inadvertently memorize sensitive, unauthorized, or malicious data, posing risks of privacy breaches, security vulnerabilities, and performance degradation. To address these issues, machine unlearning has emerged as a critical technique to selectively remove specific training data points' influence on trained models. This paper provides a comprehensive taxonomy and analysis of the solutions in machine unlearning. We categorize existing solutions into exact unlearning approaches that remove data influence thoroughly and approximate unlearning approaches that efficiently minimize data influence. By comprehensively reviewing solutions, we identify and discuss their strengths and limitations. Furthermore, we propose future directions to advance machine unlearning and establish it as an essential capability for trustworthy and adaptive machine learning models. This paper provides researchers with a roadmap of open problems, encouraging impactful contributions to address real-world needs for selective data removal.
MoreTranslated text
Key words
Machine unlearning,machine learning security,the right to be forgotten
AI Read Science
Must-Reading Tree
Example
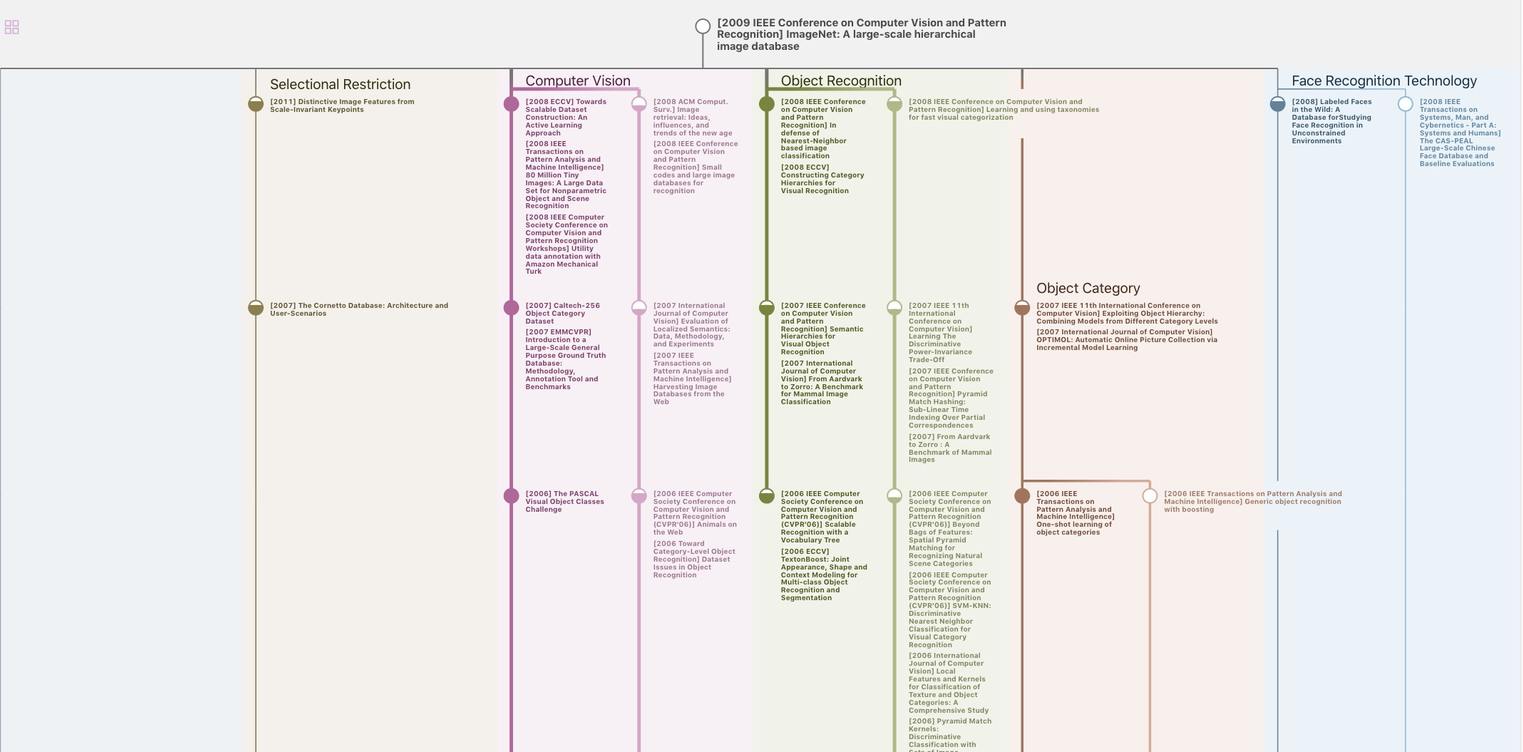
Generate MRT to find the research sequence of this paper
Chat Paper
Summary is being generated by the instructions you defined