Foundation Models in Smart Agriculture: Basics, Opportunities, and Challenges
Computers and electronics in agriculture(2024)
Abstract
The past decade has witnessed the rapid development and adoption of machine and deep learning (ML & DL) methodologies in agricultural systems, showcased by great successes in applications such as smart crop management, smart plant breeding, smart livestock farming, precision aquaculture farming, and agricultural robotics. However, these conventional ML/DL models have certain limitations: they heavily rely on large, costly-to-acquire labeled datasets for training, require specialized expertise for development and maintenance, and are mostly tailored for specific tasks, thus lacking generalizability. Recently, large pre-trained models, also known as foundation models (FMs), have demonstrated remarkable successes in language, vision, and decision-making tasks across various domains. These models are trained on a vast amount of data from multiple domains and modalities. Once trained, they can accomplish versatile tasks with just minor fine-tuning and minimal task-specific labeled data. Despite their proven effectiveness and huge potential, there has been little exploration of applying FMs to agriculture artificial intelligence (AI). Therefore, this study aims to explore the potential of FMs in the field of smart agriculture. In particular, conceptual tools and technical background are presented to facilitate the understanding of the problem space and uncover new research directions in this field. To this end, recent FMs in the general computer science (CS) domain are reviewed, and the models are categorized into four categories: language FMs, vision FMs, multimodal FMs, and reinforcement learning FMs. Subsequently, the process of developing agriculture FMs (AFMs) is outlined and their potential applications in smart agriculture are discussed. In addition, the unique challenges and risks associated with developing AFMs are discussed, including model training, validation, and deployment. Through this study, the advancement of AI in agriculture is explored by introducing AFMs as a promising paradigm that can significantly mitigate the reliance on extensive labeled datasets and enhance the efficiency, effectiveness, and generalization of agricultural AI systems. To facilitate further research, a well-classified and actively updated list of papers on AFMs is organized and accessible at https://github.com/JiajiaLi04/Agriculture-Foundation-Models.
MoreTranslated text
Key words
Foundation models,Smart agriculture,Language foundation models,Vision foundation models,Multimodal foundation models,Reinforcement learning foundation models
AI Read Science
Must-Reading Tree
Example
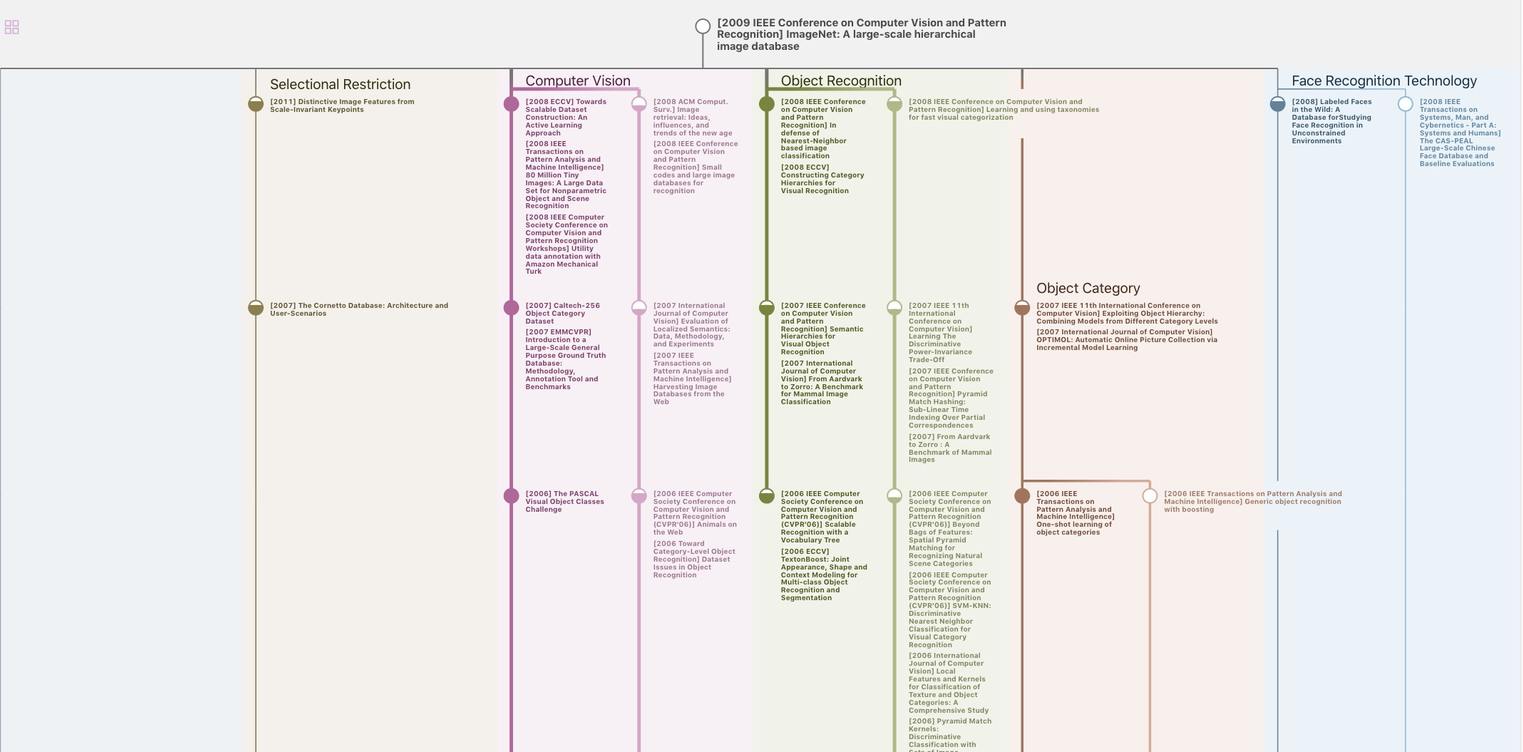
Generate MRT to find the research sequence of this paper
Chat Paper
Summary is being generated by the instructions you defined