Reinforcement Learning Approach for Dialogue Management to Aid Child-Robot Interaction in ASD Screening
2023 6th International Conference on Artificial Intelligence and Big Data (ICAIBD)(2023)
摘要
This paper investigates how Q-learning, State Action Reward State Action (SARSA), and Deep Q Networks (DQN) algorithms in Reinforcement Learning (RL) are used for dialogue management in a child-robot interaction system. Based on observations of the child’s behavior and response to questions, the system determines how to structure the next question to maintain the child’s engagement in a dialogue that is developed based on Autism Diagnostic Observation Schedule (ADOS). To accomplish this, a virtual environment is created to simulate interactions between the child and the robot, allowing for the development of an initial model that can be refined and tested in the real world. This paper presents how robust a DQN is to withstand an increasing variety of questions in dialogues. Further, it has been found SARSA is the fastest converging algorithm to learn the optimal policy for such interactions.
更多查看译文
关键词
Reinforcement learning,ASD,child-robot interaction,Q learning,SARSA,DQN,Dialog management
AI 理解论文
溯源树
样例
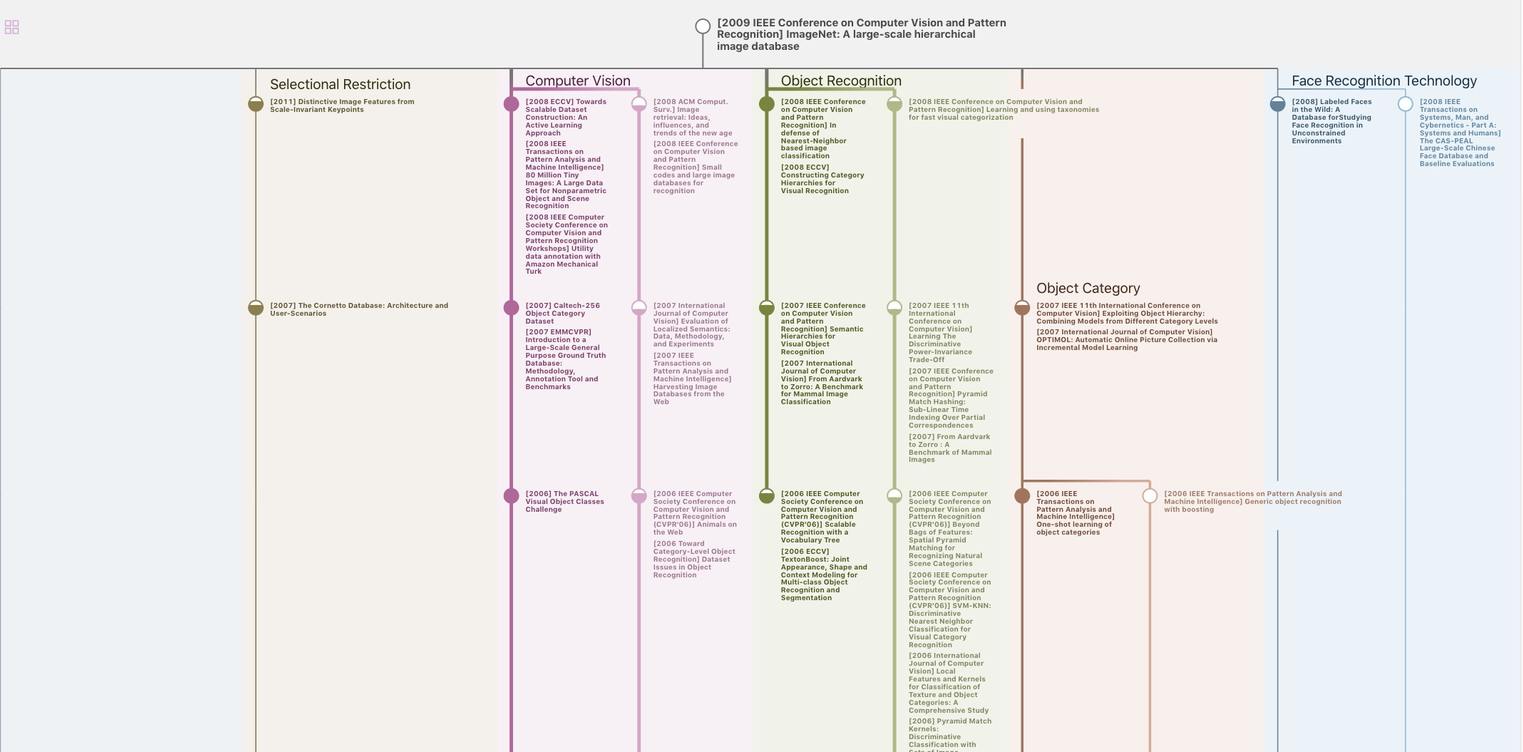
生成溯源树,研究论文发展脉络
Chat Paper
正在生成论文摘要